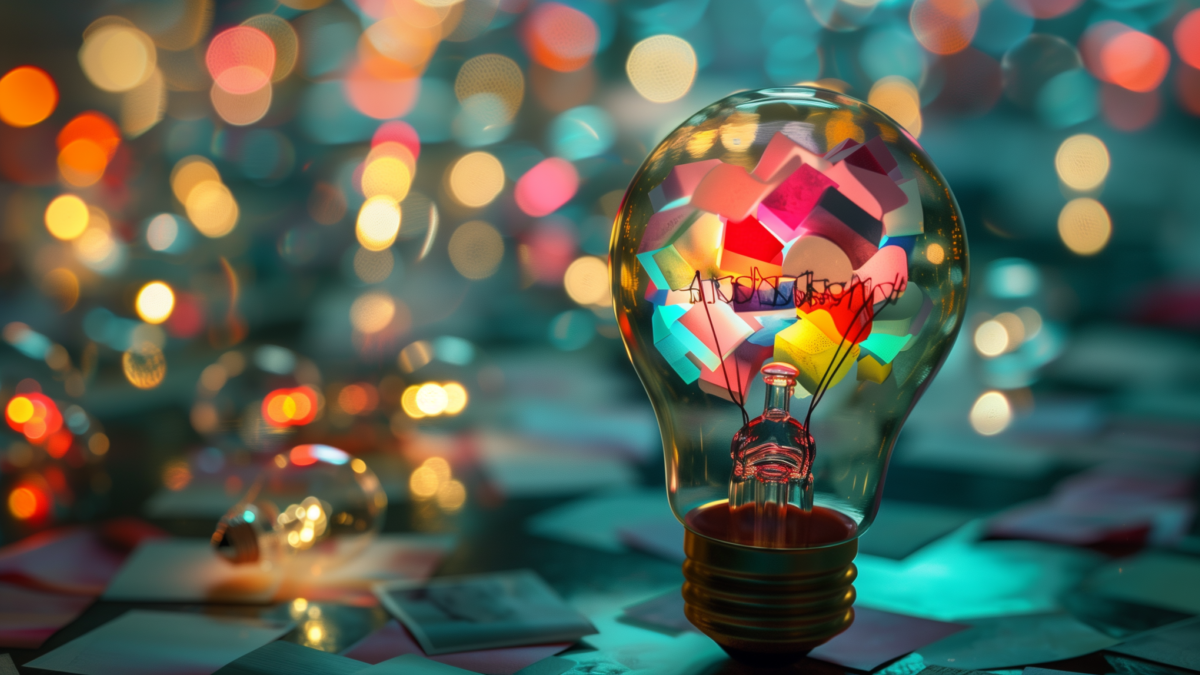
In this blog series, Jason Larson, head of content at IIA, sits down with IIA experts who serve as sparring partners for Research and Advisory Network clients. IIA’s RAN expert community, with over 150 active practitioners and unbiased industry experts, is dedicated to advising data and analytics leaders on key challenges unique to their enterprise.
In our latest Breakthrough Conversation, we dive into the impressive applications of natural language processing (NLP) within the pharmaceutical industry and beyond, with insights from Ritu Saxena. As an IIA expert and director of analytics engagement and data science at Merck, Dr. Saxena offers valuable expertise on maximizing the potential of NLP to elevate business outcomes, effectively navigate complex regulatory frameworks, and propel innovative advancements. This discussion not only sheds light on the practical challenges involved but also uncovers the future opportunities awaiting the integration of NLP into diverse sectors. By emphasizing the art and science of incorporating advanced analytics into business processes, we gain a deeper understanding of how NLP can generate substantial value. Please note that these are her own personal views and not reflecting on the company policy or execution.
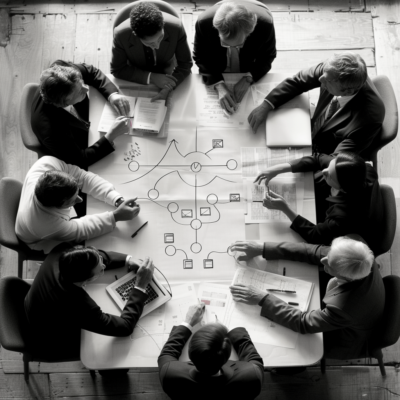
Research and Advisory Network (RAN)
How could you benefit from hundreds of C-suite level D&A executives at your fingertips? Get access to the leading network of independent analytics expertise, allowing you to apply real practitioner insights against initiatives, projects, and problems through RAN today.
How about we start with a discussion about a recent project or projects where NLP was used to improve business outcomes?
Over the past few years, my focus on utilizing NLP has greatly intensified, capitalizing on my decade-plus experience in multiple industry verticals, including the pharmaceutical industry. A key focus has been on making sense of unstructured data—from customer comments to professional feedback—which we often refer to as voice of customer. In healthcare, this involves extracting insights from the interactions healthcare professionals have with patients, often captured in electronic medical records (EMRs). These insights can be invaluable assets for informed decision-making across various departments.
Another big area where NLP has been invaluable is in mining medical literature. There’s a wealth of information out there that can be summarized and analyzed to help steer research directions—something I really wish I had during my PhD days! Additionally, NLP can be used to enhance AI/ML models by integrating structured and unstructured data sources. This approach can significantly boost the efficiency of digital therapeutics and enable their creation. These diverse applications of NLP not only generate excitement but also have a transformative impact on data handling and decision-making processes.
You’ve touched on boosting customer engagement, mining medical literature, and even enhancing AI model outputs—three substantial areas. Now, if we step back a bit, you’ve clearly pinpointed areas where you’re adding significant value with NLP and other technologies. Do you have a particular framework or method you use to identify where these tools can be most effective?
Yes, in my cross-industry experience, I have realized that whenever we talk about bringing technology like NLP into our projects, we should always start with the business needs, not with technology. I never lead a discussion with NLP, AI or machine learning. It always begins with the business questions and the value that we can deliver. In other words, our focus should extend beyond merely joining the AI bandwagon. It is imperative to carefully evaluate what is genuinely informative for making business decisions, distinguishing between what is merely "nice to have" and what truly adds value.
And when it comes to use cases, we have to be clear about the KPIs we’re measuring. In my experience, quantifying the value of NLP in monetary terms can be tricky since it encompasses more than just financial aspects—it entails assessing whether NLP can alleviate workload, speed up insight generation, or enhance current processes. For instance, could NLP make a predictive model better?
Another huge factor is cost savings, like if NLP insights can be developed in-house instead of outsourcing for any company. Additionally, we evaluate data availability and feasibility, inquiring whether obtaining the needed insights will take an extended period. If so, we weigh the benefits against the timeframe and determine if it is a worthwhile endeavor or a one-time requirement. All these considerations form part of the framework we can use, and it’s been effective across various industries I’ve worked in.
So, what isn’t NLP good for? You've mentioned the hype around AI and GenAI. With lots of internal customers getting excited, how do you clarify what NLP is really useful for? How do you steer those conversations with your business stakeholders?
Good question, allow me to share a great example from several years back which perfectly illustrates the points you raised. A request came in from a business unit asking about using NLP and text mining for a project. The voice of customer data had only about 100 responses. It became apparent that implementing complex NLP visualizations or extravagant dashboards would be overkill. Instead, it made more sense to directly read through the responses, avoiding unnecessary time and resources spent on elaborate NLP techniques.
Another thing is when requests are vague or just exploratory. While I understand the curiosity and desire to explore new possibilities from a business perspective, it is crucial to evaluate the potential value against the effort required. Also, we have to be realistic that NLP isn’t a magic solution. It takes significant time and iterations, especially to set up the right data ontology, particularly in medical terms.
And there’s always the regulatory side to consider. Just because we can do something doesn't mean we should, especially in pharma. Compliance and regulatory checks are stringent. Often, we have to start with a “no” to using unstructured data because of data access and compliance issues. But it’s all about clearly defining the problem, understanding the intent, and ensuring we’re prepared to handle any compliance challenges that might come up.
So, you mentioned that often the default answer to NLP projects is “no.” This must simplify some discussions, but I can imagine it also introduces challenges. As a leader, how do you communicate the realities—the pains and the effort involved in such projects—without sounding too negative or shutting down enthusiasm? How do you balance being realistic with encouraging innovation?
Honestly, I'm rarely concerned when the response is a “no,” because it's usually for the right reasons. For example, some projects, like summarizing publicly available content or identifying key opinion leaders, don’t face as many regulatory and compliance hurdles since they mostly involve social media or published articles. However, in the commercial space, especially in pharma, we deal with lots of third-party data, which adds layers of complexity.
Over the years, I've tried to develop several strategies to get people excited about using NLP. It starts by not focusing on the technology itself but by challenging the current ways of doing things. For instance, comparing the traditional processes to how things could be improved with NLP, emphasizing increased productivity and ease of work.
One effective approach is to involve stakeholders early on, giving them a clear vision of the potential improvements. This approach is inspired by a Japanese philosophy called Nemawashi. Conducting individual meetings to pique interest before getting everyone together to decide on the project’s direction helps in alignment. By completing a small proof of concept (POC) or a minimum viable product (MVP), we can make a significant impact. The objective is not only to showcase the potential benefits but also to make stakeholders feel a sense of ownership, as if the idea was theirs.
Finally, and most importantly, building trust is crucial, especially when it comes to navigating regulatory compliance. I try to foster a sense of ownership among the business folks, turning them into champions of the initiative. It’s all about creating a supportive environment where the business value of NLP is self-evident.
Tell me more about Nemawashi.
I lived in Japan for two years and have also worked for a Japanese pharmaceutical company. From this experience, I picked up some effective, low-friction methods for getting things done in the workplace. These approaches revolve around achieving results smoothly and minimizing disruption—something deeply embedded in Japanese work culture.
A complementary practice would be to shift the spotlight from ourselves to one of the business professionals at the final report stage. Throughout our iterative problem-solving and the sharing of interim results, we prepare a business professional to present the final outcomes. This approach has been really effective. It feels like a win-win scenario, as we enable business professionals to take ownership and become ambassadors of the insights we generate.
That's a smart approach. You’ve mentioned a few challenges along the way, especially when it comes to integrating these capabilities and gaining buy-in for your projects. Could you dive a bit deeper into those challenges? Maybe highlight two or three key issues that others should be aware of when adopting similar approaches?
Certainly, there are a few key challenges that stand out. Firstly, regulatory approval is a persistent hurdle; getting a green light on unstructured data initiatives is a complex task that requires substantial effort and clarification. Then there's the issue of data quality and diversity. In the pharmaceutical industry, as well as other fields, unstructured data may not always possess the desired level of quality, and notable gaps may exist. It is crucial to emphasize that the dependability of results relies heavily on the quality of the underlying data.
Another big challenge is health equity. Data can often exhibit biases, favoring certain groups, leading to skewed outcomes. This is something the industry is increasingly recognizing. We use AI and ML methods to try to balance these biases, but it’s a delicate process that requires thorough communication with stakeholders.
Integrating these tools into everyday workflows poses yet another challenge. Even if a proof of concept is successful, the real work lies in integrating these insights into daily operations—a step that's often overlooked. Strong interdisciplinary collaboration and engagement is needed from subject matter experts early in the process to guide the development and fine-tune the output.
So, it's not just about building the models. It's about embedding these tools into the fabric of our workflows and ensuring they deliver consistent value over time.
Before I let you go, let's look forward a bit. Could you share your thoughts on the next big opportunities in ML, NLP, and AI, particularly in the pharma industry or even more broadly across different sectors?
Looking forward, it's clear that the pharmaceutical industry needs to fully utilize unstructured data—not just one-off proof of concepts, but rather integrated as a routine part of our reporting processes. For instance, when leveraging clinical notes, EMR data, and even claims data, we have the opportunity to revolutionize our understanding of market trends and patient outcomes. Despite the challenges related to compliance, there is immense potential to enrich our insights and drive positive transformations in our industry.
Additionally, we can't ignore the advancements in AI and GenAI, particularly technologies like LLMs and ChatGPT. Our sector might be more conservative but it's essential to stay informed similar to non-traditional competitors like tech giants who are swiftly entering healthcare. Collaborative initiatives, such as the partnership between a major pharmaceutical company and OpenAI, serve as examples of the proactive steps we should be taking to seize these opportunities.
Overall, there's a tremendous amount of work to be done but also significant opportunities for improving patient outcomes through better data usage and upskilling our teams to handle new AI technologies effectively.