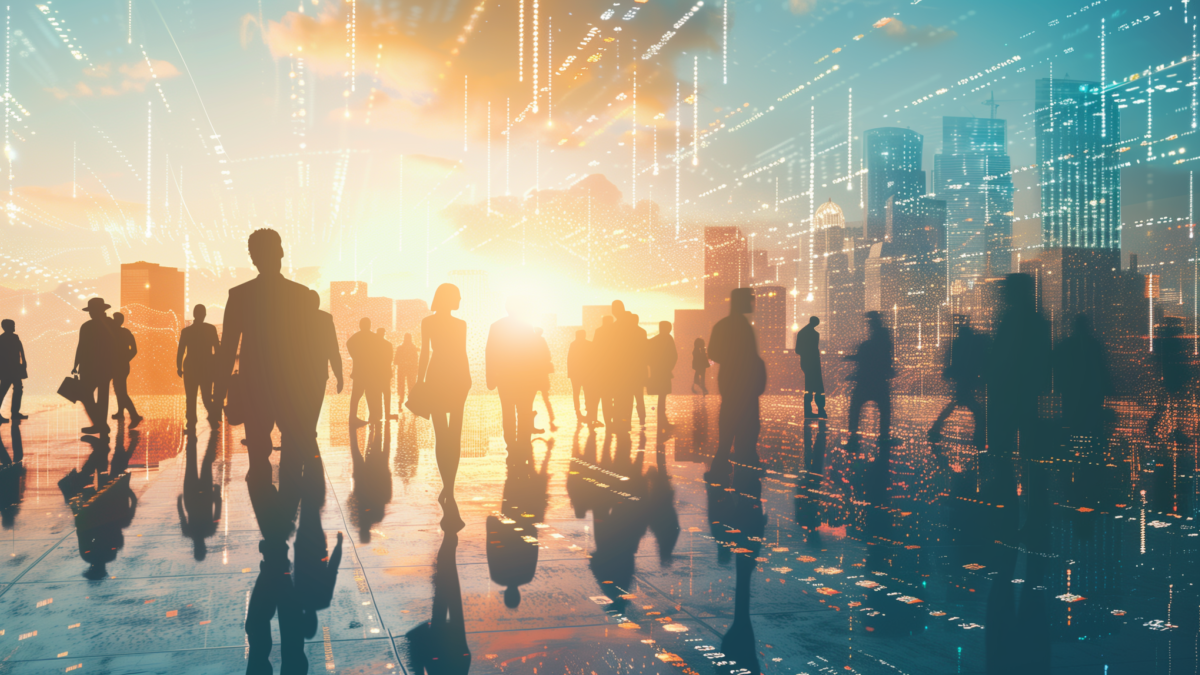
In this blog series, Jason Larson, head of content at IIA, sits down with IIA experts who serve as sparring partners for Research and Advisory Network clients. IIA’s RAN expert community, with over 150 active practitioners and unbiased industry experts, is dedicated to advising data and analytics leaders on key challenges unique to their enterprise.
In our latest Breakthrough Conversation, Sunny Zhu, IIA expert and analytics leader at Indeed shares her perspective on the intricate dance between analytics and Environmental, Social, and Governance (ESG) initiatives. The conversation explores how data-driven strategies are not just about harnessing technology but also about fostering a sustainable and equitable business culture. Zhu discusses the challenges and opportunities of aligning data analytics with ESG goals—emphasizing how this alignment not only influences company policies but also has the potential to shape societal norms. Through the conversation, Zhu unpacks the significant role of analytics in driving meaningful corporate responsibility and the impact of these efforts on various stakeholders, offering a nuanced perspective on the convergence of technology, business, and ethical governance.
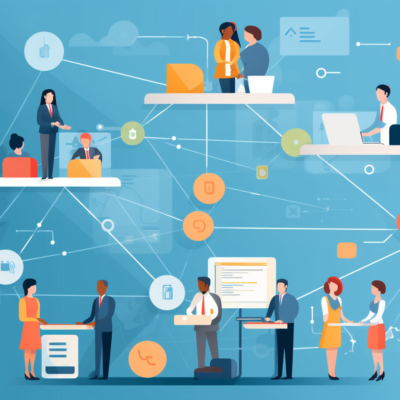
Research and Advisory Network (RAN)
Get access to the leading network of independent analytics expertise, allowing you to apply real practitioner insights against initiatives, projects, and problems.
First, briefly describe the work you and your team are doing at Indeed related to analytics and ESG strategy.
Sunny Zhu: Just to clarify, Indeed is part of a larger organization which also includes Glassdoor, both of which are subsidiaries of a Japanese company called Recruit Holdings. The ESG (Environmental, Social, and Governance) goals are actually a shared initiative between Indeed and Recruit. Each year, Recruit is responsible for reporting on our ESG performance to investors, securing funding, and demonstrating progress on these goals. So, the work we do impacts not only Indeed but also our parent company in Japan.
Thank you for the context. This is a complex environment and I’m sure you never have a dull moment.
Sunny Zhu: Very true, there’s always something interesting to work on. There's a broad spectrum of understanding and implementation of ESG across different companies, reflecting its evolving nature and the diverse focus areas within ESG itself.
Historically, ESG has been recognized for about two decades, but its components—environmental (E), social (S), and governance (G)—vary in emphasis depending on the organization. For Indeed and Recruit, the primary focus is on social aspects, though environmental and governance remain important.
For environmental goals, Indeed aims for net-zero greenhouse gas emissions by 2030. This target is relatively feasible for us as an HR technology platform, given our primary emissions are from digital infrastructures, employee commuting, and product usage, like virtual interviews which reduce travel emissions.
On the governance side, our objectives include achieving gender parity and diversity in leadership by 2030, with specific targets like ensuring 50% of management positions are held by women and 30% by underrepresented groups. We also conduct thorough analyses on leadership compensation and demographic disparities to ensure fairness and equity.
These diverse focuses help tailor our ESG strategy to meet specific, actionable goals while reflecting our corporate values and operational realities.
As I mentioned, our focus at Indeed is particularly on the social aspect of ESG. Often, when people hear "ESG," they think of environmental concerns or compliance issues—it doesn't sound very exciting, does it? But I see ESG as a way to unlock business opportunities by fostering sustainable practices and social responsibility that benefit society at large.
For Indeed, as an HR technology company that helps people find jobs, the social element is especially significant. We view this as a chance to set new standards in hiring that can hopefully improve the global labor market significantly. Our mission with ESG, particularly the social aspects, is ambitious. For instance, by 2030, one of our goals is to cut the job search duration by 50%. It sounds daunting, but it's a goal that resonates because everyone understands the importance of finding a job more swiftly—it's universally beneficial.
Another major social goal is to assist 30 million people facing employment barriers in getting hired by 2030. We've identified specific groups facing such barriers—like individuals with criminal records, those without higher education, people with long-term disabilities, veterans, and refugees. By focusing on these groups, we aim to support and empower them through their job-seeking journey.
These goals reflect Indeed's commitment to making a positive impact on the community and really showcase the potential of ESG to transform business practices for the better.
Very interesting. So, just a quick follow-up out of curiosity regarding the two ambitious social goals you mentioned: shortening job search duration by 50% by 2030 and helping 30 million people facing barriers to employment by the same year. These are significant and impactful objectives. Could you share what the current average job search duration is today?
Sunny Zhu: Yeah, that's a really interesting question. Currently, the average job search duration is about 55 days. Now, for this fiscal year, 2024, we're aiming to reduce that by 10%—down to 50 days.
Here’s the tricky part. There isn't a universally accepted way to measure job search duration. If you think about it, it's quite subjective. For instance, if I ask you how long your job search took, you might remember the day you got hired, but not the day you started looking. It's common, and it highlights the challenge.
At Indeed, our first big task is establishing a reliable measurement. Since there’s no standard, we need to come up with a method that’s simple, controllable, and reflective of the actual job search experience—though we know it won’t be perfect because even job seekers might not recall exact durations.
The next challenge is coverage. Indeed operates globally, not just in the U.S., so we need a metric that encompasses the worldwide market. Ensuring that our measurements are comprehensive and representative across different countries adds another layer of complexity. We want our data to reflect a global labor force perspective accurately.
Could we explore the challenges a bit more, such as measurement and coverage? What obstacles do you encounter in capturing this data, defining metrics, and providing analytical insights to inform business decisions?
When I think about the journey from raw data to business insights, I break it down into three key phases. First, we need to ensure we're capturing and storing data correctly—accuracy and reliability are crucial because, as the saying goes, 'garbage in, garbage out.' If the data is flawed, the analysis will be too.
The second phase is analysis. This is where our analysts and data scientists really shine. They're not just crunching numbers; they're connecting dots. It's about finding the right questions to answer—questions that align with our business context and objectives, and then applying the right methodologies to unearth meaningful insights.
The final stretch is data storytelling. This is critical because no matter how profound your analysis is, it's useless if it isn't understood or acted upon. It's all about translating complex data into actionable insights that resonate with a non-technical audience. This often means turning weeks of analysis into a compelling narrative that prompts decision-making and drives change. That last mile is where the magic happens, ensuring that our insights lead to tangible improvements in decision-making across the business.
Addressing the data storage, accuracy, and reliability in the context of ESG takes a collaborative effort. It's not as simple as having a single data engineer tackle the issue—it spans across various departments including IT, legal compliance, HR, and research and development. This cross-functional involvement is crucial because ESG touches so many areas of an organization.
Additionally, we handle sensitive demographic data and personally identifiable information, which must be managed with great care. Every step in data collection, storage, and sharing must be meticulously governed by robust standards to ensure security and compliance.
For environmental data, the quality can often be challenging. We're tasked with understanding aspects like electricity usage across various facilities, which involves standardizing data from diverse sources. For example, estimating gas emissions from employee commutes requires sophisticated modeling to make educated assumptions. We collaborate with third parties to validate our methodologies and ensure our reporting is transparent and up to standard to avoid any risks of greenwashing. This comprehensive approach is necessary to uphold the integrity of our data and, by extension, our ESG initiatives.
In the second part, when you're analyzing data, the real challenge is connecting the dots and finding the right metrics to truly understand the problem. People often think that just using the latest model or technology will solve everything. But data alone isn't enough; it's crucial to integrate it within the business context and pinpoint the exact problem you need to solve.
I've seen many analysts and data scientists dive deep into the data, slicing it every which way. Yet, despite their efforts, the results often lack meaning—they don't answer the right questions or provide insights that genuinely aid the business. The real hurdle isn't the technical aspects; it's the steep learning curve involved in understanding the domain thoroughly enough to connect those dots effectively.
The key challenge here is not just technical—it's about understanding the business context intimately and formulating the right questions. That's how you make impactful decisions and drive ESG goals forward. This middle stage of translating data into actionable business strategies is where many teams face the biggest challenges.
In data storytelling, there are essentially three components that I consider crucial. First, the data itself must be accurate and relevant. If we're not starting with reliable data, the entire narrative loses credibility. Second, the visual aspect is vital; how we present the data can greatly influence comprehension. Without clear and compelling visuals, even the best data can be overlooked or misunderstood.
The third component is the storyline or insights. This involves crafting a coherent narrative that not only presents the data but also explains its implications, guiding the audience to understand the why behind the numbers. It’s about making the data relatable and actionable.
However, the most significant challenge often lies in communication, especially within a diverse and mature organization like Indeed. Different teams may have varied levels of understanding and different perspectives on data utilization. Bridging these gaps and ensuring that everyone can follow the data story—and see its relevance to their roles—requires careful planning and clear communication. This ensures that insights lead to informed decisions across the company.
Communication really is the crux of it all, isn't it? It's one thing to think you've made yourself clear, but entirely another to see it interpreted differently than intended. That last mile—ensuring your insights lead to action and transformation—is all about effective communication. It's crucial because it involves multiple layers. It's not enough to just get buy-in from one person; you need to cascade your message and strategy across various levels of the organization. This isn't just about convincing one layer; it's about reaching alignment throughout the entire hierarchy to truly see the magic happen. That's why communication isn't just a challenge; it's a pivotal element in leveraging data for impactful decision-making.
The framework you've outlined really brings this process to life. Focusing on that middle mile of connecting the dots, if you were mentoring a data scientist or analyst, what would be the first piece of advice you'd give them? What are the best practices in overcoming this barrier?"
Sunny Zhu: That's a fantastic question. It makes me wish someone had inspired me to think this way when I started my career. To be honest, I'm not sure if there's a "best practice" per se, but I've learned a couple of important things over time. First and foremost, clarity on the business goal is crucial. Many analysts and data scientists, especially when starting out, tend to focus too much on specific questions or get lost in technical details, losing sight of the bigger picture. It's essential to understand what the ultimate goal of the analysis is and what it means to move the needle for the business.
Analyzing data can sometimes feel like a game where you're constantly trying different approaches to make sense of the data. Not every method will yield interesting results, and that's okay. What separates good data scientists from great ones is their intuition about the business and their domain. This intuition helps them to think creatively about how to approach the data and uncover meaningful insights.
The second key point is learning from your team and broader stakeholder group. Often, data scientists focus on learning the most advanced techniques or the latest technology from their immediate peers. While mastering technical skills is important, gaining perspective from those in completely different functions is invaluable. These stakeholders can provide fresh insights and context that enrich your understanding of the business problems you're trying to solve. Engaging with diverse teams helps ground your analysis in reality and ensures it's aligned with broader business objectives.
For instance, if you're a product data scientist, you might spend days analyzing data without uncovering anything significant. However, by discussing the project with your product manager, UX/UI designer, or engineer, you can gain new perspectives that are crucial for the feature you're developing. Understanding what's important to them can provide fresh insights and help you see connections you might have missed. Engaging with people from varied backgrounds and consolidating their views is essential. It’s not just about finding a consensus. It’s about understanding the range of opinions, discerning which are data-driven, and then integrating this information to form a holistic view. This approach doesn’t just apply to solving technical problems but also enriches your analytical work, ensuring it aligns with broader business goals and addresses real-world needs.
Now, let’s dive a bit deeper into that critical last mile—the challenge of communication. You've elegantly broken down the data storytelling process into its essential components: data, its visualization, and then crafting a compelling narrative. This approach really emphasizes the importance of creating a story that resonates across the business. When discussing communication, it struck me as a quest for common understanding and alignment with stakeholders. Drawing from your experiences, what key lessons have you learned about effectively communicating data stories?
Sunny Zhu: For sure, finding common ground is definitely a good starting point. It's crucial to know your audience before diving in, because different people have different levels of understanding. Knowing who I’m speaking to helps me determine the base knowledge to start from, allowing everyone to get on the same page.
Besides establishing a common ground, another challenge is the complexity of data analytics. Often, after conducting thorough analyses and feeling that the results are clear, it's surprising when others don't follow. Not everyone thinks like a data analyst or scientist, and sometimes, data professionals take for granted that others will understand their thought process.
When it comes to data storytelling, think of it as simplifying a tangled rope. It's not necessary to show the audience every step of untangling; rather, you should present a clear, straight path from A to D, focusing on the key points. This approach helps prevent overwhelming your audience with too many details.
I've learned it's crucial to distill your findings to the most relevant points that directly address the business problem. Like writing a story, your presentation should have a clear setting, conflict, and resolution, focusing only on the essential elements that lead to a clear understanding of the data’s implications. This targeted approach ensures the audience walks away with a precise understanding of what the data means and its relevance to the business challenge at hand.
This conversation has been incredibly enlightening and enjoyable. Before it comes to end, let’s look forward. What excites you most about the future of data and analytics? This can be related to your role at Indeed, particularly around ESG initiatives, or more broadly within the analytics landscape. What inspires you as an analytics leader?
That's a good question. If I think about what excites me the most, it's the rapid evolution of technology and the growing awareness of ESG responsibilities. The developments in AI, like ChatGPT, spark discussions everywhere about future applications. At the same time, the regulatory environment around ESG is tightening in Europe and beyond, which is making companies take their responsibilities more seriously.
However, what truly inspires me as an analytics leader isn't just the technological advancements or regulatory changes. It's more about the impact on human lives. For instance, as a parent, I hope that ESG efforts today will lead to a better world for our children. I want them to find meaningful work and to appreciate diversity not just in the workplace, but in everyday life. That human aspect—how companies can help create a sustainable future and enhance life quality—is what excites me most. I think about the social goals of my work, like helping people find jobs more quickly because their families depend on it, or improving workplace well-being. That mission drives me more than any complex data challenge could.