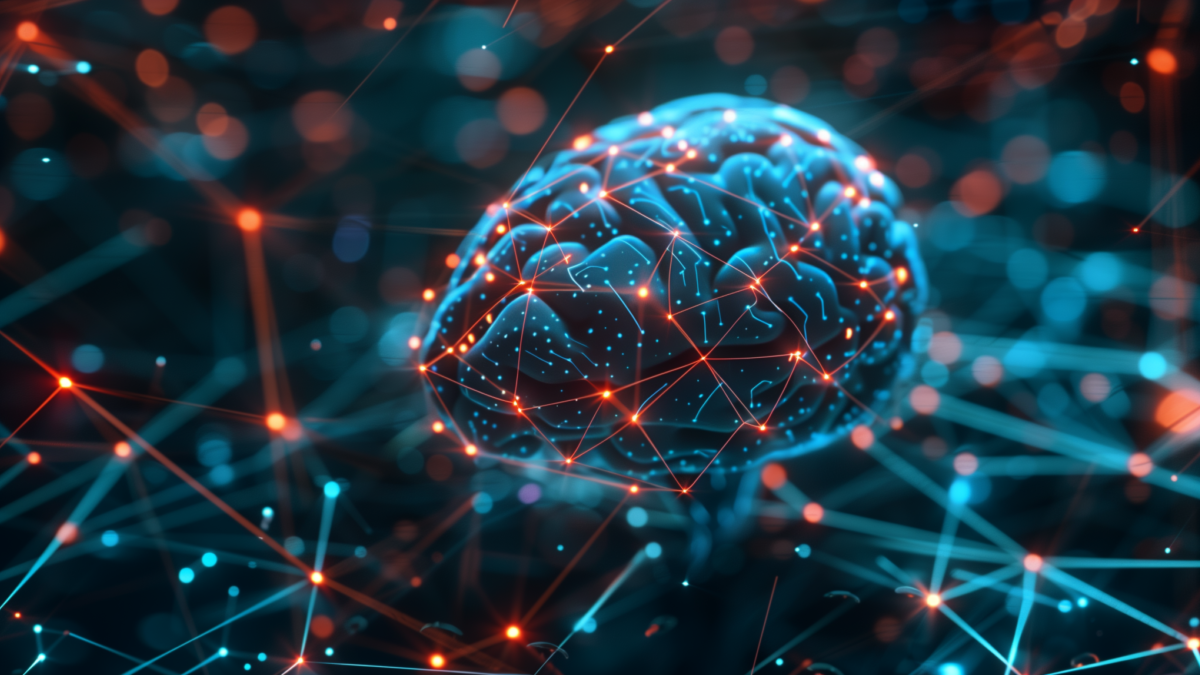
In this blog series, Jason Larson, head of content at IIA, sits down with IIA experts who serve as sparring partners for Research and Advisory Network clients. IIA’s RAN expert community, with over 150 active practitioners and unbiased industry experts, is dedicated to advising data and analytics leaders on key challenges unique to their enterprise.
Brian Kleinfelt has led the data literacy efforts at two large, multinational organizations, and guides IIA clients through challenges related to building and maturing data literacy programs. In this conversation, Kleinfelt brings a wealth of experience and knowledge to the table, as we discuss a range of issues around how the latest wave of AI has impacted data literacy programs and strategic recommendations to align data capabilities with broader business goals across various sectors of the business.
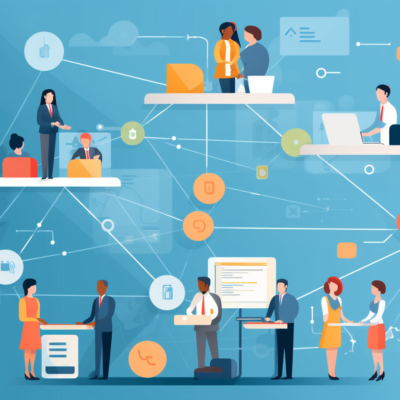
Research and Advisory Network
Get access to the leading network of independent analytics expertise, allowing you to apply real practitioner insights against initiatives, projects, and problems.
How have you defined data literacy for organizations? How has this definition evolved, if at all, with the emergence of AI technologies?
Brian Kleinfelt: Defining data literacy can vary widely, often depending on one's background. I prefer to define it as the ability to read, write, and communicate with data in context. The “in context” part of the definition is really important because the language of data often varies across different sectors like sales and marketing, as well as across different industries, each with its own nuances.
As for its evolution, I've given this quite a bit of thought. In some ways, data literacy hasn't changed because concepts like AI have always been a part of it. However, AI’s growing presence in mainstream media and organizations presents a unique opportunity. We might use AI to spark interest and drive engagement in data literacy programs. Whether we start referring to it as “data and AI literacy” or keep the current nomenclature, the goal is to engage more people. So, the essence of how we approach data literacy remains the same, but we’re now leveraging AI's visibility to enhance interest and participation.
Let's discuss the integration of AI into data literacy programs. Despite AI's longstanding presence, its visibility, to your point, has significantly increased in the eyes of corporate boards and CEOs, elevating both the pressure on data leaders and the opportunities for impact. How do you capitalize on this heightened awareness to drive engagement in data literacy initiatives? Where should organizations start?
Brian Kleinfelt: The approach is somewhat dependent on the organization's context. Early on, as senior leadership began focusing on AI, one of our initial steps at one company was to roll out basic training. This helped start the education process on AI fundamentals across different groups within the company. We categorized our audience into two main groups for simplicity: data and analytics practitioners, and the broader business staff.
For the practitioners, who already had some familiarity with AI or were engaged with it in their roles, the focus was on hands-on experience. At a different company, during a similar shift toward AI, we introduced a basic “Introduction to Artificial Intelligence” course. This was tailored slightly to our industry to help employees connect new concepts with their existing knowledge. At the same time, we were incorporating new tools like Databricks into our operations. We emphasized practical application—getting our staff to use these tools directly rather than just learn about them theoretically.
This hands-on approach didn't just apply to our tech teams. Given the partnerships across the tech industry—even among competitors—it was essential for everyone to understand and utilize these collaborative tools effectively. With tools like Copilot X integrated from the start, we could demonstrate their potential and how they could simplify their work.
For the broader business audience, the goal was to provide a basic understanding of AI. This is crucial because there's a lot of fear driven by media misconceptions about AI, like fears of job loss to automation. It's about demystifying AI and showing its real-world applications, rather than letting sensationalist views shape our understanding.
Returning to the topic of training, could you discuss the challenges of aligning your organization's understanding of AI? Specifically, how do you address the nuances between broad AI concepts and specific sub-technologies like generative AI and machine learning? Is there a lot of confusion around these terms, and does it take significant effort to overcome these definitional challenges? Once you've established a foundational training program, is it easier to progress to more advanced topics?
Brian Kleinfelt: I haven't encountered significant issues with defining AI and its sub-technologies. However, it's always beneficial to clarify these terms during discussions. In the “Introduction to Artificial Intelligence” training I mentioned earlier—which is a concise 25 to 30-minute session—we specifically addressed the techniques we used internally, such as machine learning, neural networks, computer vision, and NLP. We provided four distinct examples, each illustrating what these technologies are and how they differ from each other. But, of course, this is just a basic overview. Given the vastness of the field, you could have a master's program dedicated solely to machine learning. The challenge for organizations is the overwhelming amount of information available and the impracticality of creating training that covers every aspect in depth for every potential user. So, we often rely on existing resources, like authoritative YouTube channels that explain these techniques and approaches at the right level for the audience. Like the introduction of computers into the workplace, AI's integration is inevitable and will become the new normal. Thankfully, we've seen a positive reception to these trainings as people recognize their value. Now, the focus is on aligning leadership to effectively build and implement a robust data literacy program.
Considering the introduction of generative AI, could you compare the data literacy programs before and after its adoption? GenAI has become quite accessible to those unfamiliar with AI technologies, seemingly creating two different eras in data literacy. Have you noticed any differences in the acceptance or resistance to these programs before and after GenAI's integration? Could you share your observations on the overall sentiment toward these changes?
Brian Kleinfelt: Reflecting on the conversation about the post-genAI data literacy program, I don't see it as drastically different from the earlier version. It's mainly about updating the training content and enhancing support systems. Data literacy transcends just creating training programs; it's about building a comprehensive support and enablement system.
In my experience with two organizations, we've seen significant benefits from establishing a supportive environment where individuals felt comfortable seeking help. This approach prevented them from potentially feeling embarrassed in front of their supervisors by not knowing something. Instead, they had a reliable place to ask questions or seek general advice.
We facilitated a lot of internal requests for support, ranging from connecting staff with the right experts to providing resource links that are readily available within the organization. These mechanisms of support remain constant, regardless of the technology focus.
This includes everything from addressing initial problem-solving approaches that tie directly to business value, to decisions at the end of the cycle—how we make them, justify them, and execute them. These inquiries span the entire spectrum of the literacy program.
It's important to note that for many of us in the workforce this wasn't part of our early education. Unless you’ve come out of college recently, much of this is new territory that requires thoughtful introduction and integration into our professional lives.
Excellent points. I'd like to delve deeper into the scope of your data literacy programs. However, I'm also curious about the dynamics between your data and analytics professionals—what we like to call the supply side—and the business stakeholders, or demand side. IIA often discusses this framework in addressing the challenges data organizations face. Could you share the specific challenges you encountered while promoting AI literacy and how you addressed them? In particular, could you compare the approaches and challenges between educating analytics professionals and business folks in data literacy? What have been some of the most significant pain points, and how have you overcome them?
Brian Kleinfelt: Addressing the distinct needs of data and analytics professionals versus business users is central to our data literacy programs. It's well-acknowledged in our field that there's often a significant gap in understanding between these groups. For example, data professionals may not fully grasp business needs and the specific language used, and the reverse is true as well.
Educating and supporting business users tends to be more straightforward. There’s a wealth of accessible content aimed at enhancing basic knowledge and comprehension of data terminologies. However, creating resources like a definition library can be challenging. The effort required is substantial because it demands comprehensive representation from all business areas. This is crucial because different departments, such as marketing and sales, may define key terms like “new customer” differently. Aligning these definitions across the company is essential to ensure data consistency. Without this alignment, data reported to leadership can be confusing and appear contradictory, like comparing apples to oranges.
A major challenge in implementing data literacy programs, whether for business users, data professionals, or both, is that “data literacy” often becomes just a buzzword. Organizations may claim they're fostering data literacy but lack a deep understanding of what it truly involves or how to effectively implement it. This superficial approach can lead to stagnation. To overcome this, it's crucial to have strong executive sponsorship from leaders who truly grasp what data literacy entails. If we don't have a shared understanding of what data literacy entails, we're at a standstill.
Another major challenge, although not one I've personally encountered as much, is the allocation of responsibilities. Often, data literacy responsibilities are added as a side project for someone with an analytics background but without program development experience. Many data literacy programs end up being merely a series of training sessions. While my background is in training, and I value it, training alone is insufficient—it only takes you so far.
Leadership support is crucial, as is dedicating time and resources to develop a comprehensive program. It involves significant buy-in across the organization, even if you have an executive champion. My role as a data literacy lead often involves engaging with various vice presidents across departments like sales, marketing, and IT to demonstrate value and understand their primary needs. While they generally express similar needs, they still want their voices heard. This level of relationship building is challenging if data literacy is merely a secondary responsibility. I've observed some programs lose momentum and eventually fizzle out due to this lack of dedicated focus.
Given the significant role that building relationships and securing buy-in played in your job, can you share an example of a stakeholder who was particularly challenging to persuade? What strategies did you use to effectively engage them and overcome those hurdles?
Brian Kleinfelt: Certainly, there's a notable example I can share. There was a division within an organization that initially had only lukewarm interest in our data literacy initiatives. However, this division was closely associated with our executive champion. I managed to engage the vice president of this division by inviting them to one of our decision-making workshops. This workshop walked participants through our decision-making framework, which seemed to spark their interest. They attended actively, and I believe that experience showcased the practical application and value of data literacy, especially from a business perspective.
The key takeaway for this vice president wasn't about learning technical skills like programming in Python or developing machine learning models; it was seeing how these principles could be pragmatically applied to our business processes. The workshop demonstrated a clear, structured approach to working with data that, once widely adopted, would ensure everyone in the organization understood how to address issues, tie efforts back to business value, analyze data effectively, choose the right tools, and communicate findings both internally and externally.
The turning point was getting them personally involved and interested, which is often crucial for gaining the support of stakeholders who might otherwise be too busy to engage. This personal involvement can lead to a deeper understanding of the program's value and a greater willingness to support its integration across the company.
That's a great example. Thank you for sharing. I'm particularly interested in a couple of points you raised—the challenge of side projects for data literacy programs, often managed by analysts, and the prevalence of a checkbox mentality around data literacy, which can feel more like a buzzword than a meaningful initiative. Could you elaborate on how you've managed to navigate these issues, especially the latter? How do you move beyond the superficial approach to data literacy that many organizations seem to settle for?
Brian Kleinfelt: Demonstrating value is really what it all boils down to, right? The main goal is to improve business outcomes by fostering a more data-literate organization. Do I think there's a perfect way to measure ROI on that? Honestly, we're not there yet. It's a huge task that involves monitoring, surveying, and evaluating business results—comparing those who are data literate to those who aren't—and it's pretty much a full-time job.
What we try to do is multifaceted. For example, one of the ways we try to gauge the success of our programs is by adopting models like the Kirkpatrick four levels of evaluation, recently expanded to Phillips' five levels. This framework helps us to check if people enjoy the training, what they've learned, and most importantly, if they are applying these skills in their work.
We don't have someone watching every move our employees make. But what we can do is send out surveys to those who have participated in our programs or use our resources extensively. We ask them, “Have you applied what you learned? Yes or no? If yes, how? What changed because of it?”
Then, we take those success stories and we shout them from the rooftops. We showcase these examples as proof of how changes in data literacy can really transform how we work across the organization. It’s about showing, not just telling, that becoming more data literate can make a real difference.
Reflecting on your experiences in developing or enhancing data literacy programs, what advice would you offer to your past self? Are there specific pitfalls to avoid that would not only accelerate momentum but also ensure the creation of a comprehensive, effective program? This seems to be a recurring theme in our discussion.
Brian Kleinfelt: That’s a key question, really. Reflecting on experiences, one intriguing aspect is how near misses can reinforce the behavior that led up to them. This ties into how we approach building data literacy programs. There's always a debate: Should you roll out the program to the entire organization at once, or should it be more targeted at first?
In my first attempt, I launched the program organization-wide all at once, and it worked out. But, honestly, I think there was a bit of luck involved. If I had to advise my past self, I’d suggest starting more focused. Identify where you can make the most impact and ensure you have strong leadership support. This approach helps in highlighting early successes, which is crucial.
For example, when we showcased how we revamped our decision-making process or introduced a new data visualization tool that dramatically met customer needs, it naturally encouraged others to follow suit. Success breeds interest. People are drawn to successful outcomes; it's just human nature. So, focusing your initial efforts where they can quickly show value might be more effective than a broad, all-encompassing rollout.
That’s an insightful recommendation. Given your experience integrating AI into data literacy programs across various organizations, and considering future trends in AI, are there any specific developments or aspects that you think need more attention in data literacy or combined data literacy and AI programs? Although core elements like the curriculum have remained stable despite the emergence of technologies like Generative AI, looking ahead, what areas do you believe we should focus on?
Brian Kleinfelt: It's fascinating to discuss trends, especially around AI ethics and safety. I'm a staunch advocate for data and artificial intelligence, but I'm not immediately concerned about large-scale societal impacts. In the long term, of course, we need to be cautious about how this advanced computational ability could be misused by those with nefarious intentions. There's undeniable potential for AI to contribute positively, with organizations exploring how to leverage data in solving global challenges like hunger. Yet, we're somewhat in the Wild West phase of AI development. It's crucial that we address data bias and ensure AI's recommendations are responsible and just.
Regarding explainable AI, especially with tools like Microsoft Copilot X integrated directly, it's crucial to understand how these tools derive their recommendations. Yes, it suggests next steps or analytical approaches, but the underlying logic must be transparent. Do we have human oversight in these processes?
This discussion naturally leads to whether we are data-informed or data-driven. I advocate for data-informed decision-making. While some operational decisions, like shutting down a malfunctioning plant, can be automated, the majority of organizational decisions still require a human in the loop. For instance, AI might suggest a course of action that was proven disastrous decades ago due to factors it can't comprehend, the way the model was trained, and information it doesn’t have access to.
Then there’s the issue of low-code/no-code tools. They've been around for a while and offer significant capabilities. However, they also pose risks if users lack a strong foundation in the underlying principles like data analysis or mathematics. These tools empower users to make decisions that may seem sound but are fundamentally flawed. For example, an enthusiastic mid-level employee might use such a tool for a complex analysis without fully understanding the implications, leading to potentially harmful business decisions.
Data literacy programs serve as a safeguard, ensuring individuals understand how to use data responsibly. They're not just about facilitating more efficient data usage; they're about preventing misuse and guiding informed decision-making across all levels of an organization.
The cynic in me says that humans tend to be lazy, especially under stress. It's like choosing the path of least resistance when we're overwhelmed and juggling many tasks in a high-pressure environment. Given this, how do we promote healthy habits and behaviors around these powerful tools? To your point, now that we have access to just about any answer, how do we ensure people are making informed decisions rather than just guessing, unless they're deeply experienced in a specific area?
Brian Kleinfelt: If I had the answer to that question I would be sitting on the beach in Hawaii with billions of dollars in the bank [laughs]. You know, I've been exploring the capacity of the human brain a lot lately, particularly its ability to process the enormous amounts of information we now face. We're at a unique point in history where it seems we've surpassed the brain's capacity to handle all this data effectively. This overload often results in people opting for quick solutions, fixing issues later—which, in some industries, can lead to serious consequences.
The inevitability of using data is much like the adoption of computers in the past—no one today seriously opts to write memos on a typewriter instead of using a computer. Similarly, we will all need to adapt to using data effectively. We must find ways to quickly upskill people and enable their performance in a world that's accelerating all around them.
Looking to the future, ideally, you wouldn't need a specific data literacy program in your organization. Education from K-12 through higher education should ensure that everyone is data literate by the time they enter the workforce.
As we wrap up our conversation, do you have any final thoughts or key messages you'd like to share with your peers on this topic?
Brian Kleinfelt: Definitely, the top thing I'd emphasize, and it's something I've repeated, is the necessity of a strong executive sponsor who not only grasps what data literacy is but also empowers you to implement it. Training alone isn't sufficient. It's about creating an entire support system to foster effective use of data across the organization.
Regarding investment, it doesn't have to be massive right away. I've talked at conferences about starting small. That's how I began—slowly building up a team and support structures. The scale of your effort should match the size of your organization. A team of one might suffice for a hundred employees, but a larger organization will need more resources.
You also need a competent data literacy lead. Whether they come from a data background or a training and program management background like mine, what's crucial is their commitment to the role. For a substantial company, investing in this position won't break the bank.
Lastly, support needs to be comprehensive. Our approach considers a wide range of needs—from new data analysts to senior data scientists, and from entry-level staff to senior leaders. For example, the executive champions guide I developed was very well received. It included key data literacy points, target audience details, and ready-to-use resources. I make it as easy as possible for leaders to advocate for data literacy. They just need to endorse and share the prepared resources. It's crucial because if leaders aren't invested in data literacy, the initiative won't resonate throughout the organization.