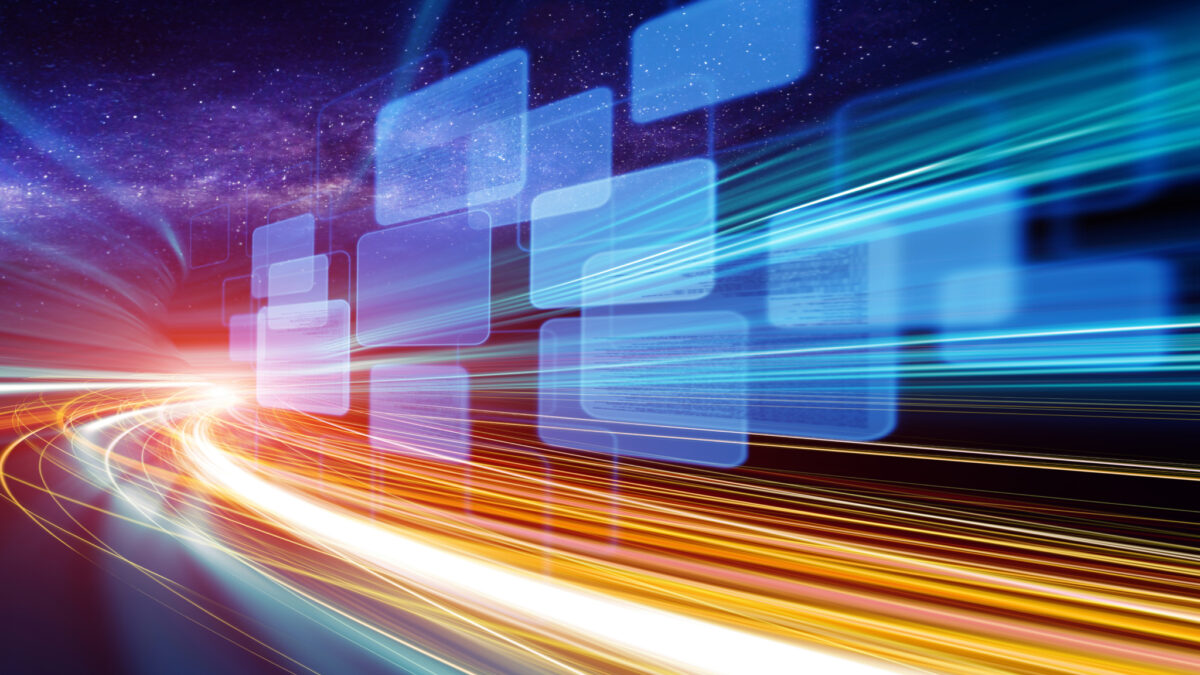
In a previous post, I described the importance of developing an analytics and AI strategy, and some aspects of the process by which a strategy can be developed. In this post, the focus is on the specific components of the strategy—on the topics and issues it should address. Of course, the specific strategic foci an organization addresses may vary, and determining which ones to include can itself be the focus of the early stages of a strategy process.
The strategy components I describe in this post are in rough order of when they should be developed. For example, it’s difficult to develop a talent strategy without knowing what the strategic portfolio, use cases, and technologies will be. Some strategy components, such as a change management strategy, are important but may not be explicit in a strategy document.
The Strategic Portfolio
One key issue that should be addressed early in the strategy process is what areas are included in the AI and analytics portfolio. There are a variety of possibilities, including the following:
- Business intelligence, which typically employs descriptive and visual analytics
- Predictive and prescriptive (“advanced”) analytics, which uses more complex statistical methods
- Artificial intelligence, involving various technologies like machine learning, natural language processing, etc.
- Data management and infrastructure, including the development of new platforms and maintenance of existing ones
- Data engineering for particular applications.
There may be other functions as well.
It’s important to decide and announce not only what areas are included, but those that are not. Of course, this is dependent on organizational history and context, including the areas of responsibility for the leader of the analytics and AI organization—e.g.., Chief Analytics Officer vs. Chief Data and Analytics Officer. Independent of a specific organization’s context, I would argue that advanced analytics and AI are so similar that they should almost always be combined. And it is also helpful to have significant data management functions in the analytics and AI camp as well.
Operational and Organization Model Strategy
Perhaps the second issue that an organization should address in its analytics and AI strategy is the business model and fundamental organizational structure by which it will operate. This strategic component deals with how scarce analytics and AI resources will be allocated, and what analytics and AI-related services will be provided to internal (and in some cases external) customers.
Operational models are often combined with organizational and funding models. Several common (but not exhaustive) combinations include hub-and-spoke or federal models; center of excellence models; self-service models; or strategic applications only (data products).
The choice of operational and organizational model is likely to change over time in most companies. One large retailer, for example, reorganized and changed quickly from a centralized model focused on building important analytics and AI applications, reporting to a chief digital officer on the business side, to a decentralized model reporting to the chief information officer and focused on meeting all needs (service bureau) for analytics and AI services.
Problems/Opportunities/Use Cases Strategy
One key issue that almost every organization faces with analytics and AI is where in the business to apply them. What business problems, issues, or opportunities can benefit from the use of these powerful tools? Analytics and AI can be used to reduce costs; improve speed to execution; drive innovation; reduce or explain complexity; and change relationships with customers or suppliers. More specifically, analytics and AI can be applied to improve various business processes; enhance or enable products and services for customers; make better decisions; automate tasks; pursue new markets; or support other objectives. Some objectives seem to yield more value than others; a 2021 MIT Sloan Management Review survey found that companies that use AI primarily to explore and create new forms of business value are 2.7 times more likely to improve their ability to compete with AI than those who use AI primarily to improve existing processes.
There are also many possible industry- and company-specific objectives that could be part of an analytics and AI strategy. For example, a company might want to better understand and develop relationships with a particular type of customer, and it could use machine learning to analyze highly granular data on the customer. It might also be interested in analysis and predictions from data in a customer loyalty program. Or it might want to embed analytics or AI capabilities into a particular type of product.
Some organizations place a strong emphasis on a particularly broad use case in their analytics and AI strategy. At Morgan Stanley, for example, Chief Data and Analytics Officer Jeff McMillan has focused for many years on a wealth management “next best action” system and communications platform. The system allows the firm’s Financial Advisors to identify customized investing opportunities at scale using machine learning, and the communications platform delivers them to clients on a regular basis.
This decision of which use cases to emphasize should largely be driven by business strategy. A large wireless telecom firm, for example, was beginning to experience small losses in revenue after many years of rapid growth. Its analytics and AI applications were focused on market growth—such as personalized recommendations of new products and services, intelligent agents to increase customer satisfaction, and highly granular customer analysis to identify those most likely to spend more with the company.
Technology Strategy
What technologies should an organization acquire or develop in order to meet its objectives with analytics and AI technology? That is a complicated question that is important for organizations to answer. Analytics and AI are not one technology, but a collection of them, including data management tools, business intelligence tools, visual analytics, statistical machine learning, neural networks, natural language processing and generation, robotic process automation, and so on. Even familiar technologies like spreadsheet analytics and rule engines can still be useful for some applications. Companies need to decide which technologies they plan to use, and in many cases to also pick particular versions or vendors of them. Beyond the choice of technologies, companies need to decide whether to build or buy the technology capabilities, whether to use proprietary or open source software, whether to use a single vendor’s tools or employ “best of breed,” whether to use a broad “platform” or single applications, and so on.
There are a set of matches between particular technologies and business problems that are likely to be better than others in technology strategies. A full mapping is beyond the scope of this post, but if, for example, your organization has voluminous and rapidly changing but structured (i.e., in rows and columns of numbers) data about customers, machine learning will probably provide a better understanding of their preferences. If you have sounds or images to identify, deep learning neural networks are likely to be the best bet—and so on. Even very familiar domains like business intelligence, however, are changing rapidly and need to be re-examined often.
Talent Strategy
A key question for any organization pursuing analytics and AI initiatives is where to get people who can do such work. This is not, of course, a new issue; companies have had challenges for many years in securing qualified quantitative analysts, and then data scientists. The good news is that since these challenges surfaced, universities (more than 200 of them in the US alone) have been churning out large numbers of graduates who are broadly educated in analytics and data science. The bad news is that they may not have been trained on the particular tools and methods your organization has adopted.
The choices for talent strategy are similar to those for technologies: buy, build, or rent. To buy people is to hire those who already possess the needed skills (or “acquihire” them in an acquisition). This will be particularly difficult if your company is not in large E ast or W est C oast cities, and it is not willing to pay large compensation figures, including stock options. It will also be important to such individuals that you have plenty of data to work with and that your organization is undertaking some interesting challenges in the field.
To build people is to train them in the needed skills. This will be much less difficult if the candidates for such training already have basic statistical and data management skills.
It is relatively rare for companies to engage in substantial efforts to train or retrain their employees in analytics and data science skills, but it should be more common. Cisco Systems, for example, created a distance learning program for aspiring data scientists with two different universities. The program lasts for nine months and concludes with a certificate in data science from the university. Several hundred data scientists have been trained and certified, and are now based in a variety of different functions and business units at Cisco. In addition, the company created a two-day executive program led by business school professors on what analytics, data science, and AI technologies are and how they are typically applied to business problems. The program also covers how to manage a workforce that includes data scientists, and how to know whether their outputs are effective.
If a company has a “self-service” approach to analytics and AI and plans to employ recent technologies like automated machine learning that facilitate “citizen data scientist” roles, its “build” strategy for talent would be somewhat different. The goal would be to educate employees about how machine learning works and how to use the relevant tools, rather than to create data scientists.
A third basic option is to “rent” an analytically informed workforce by hiring consultants or vendors to deliver services. This strategy is widely practiced by companies that don’t have the in-house expertise to build analytics and AI applications. It can be successful if the vendor or consulting firm has sufficiently well-trained people (although they also suffer from the shortage and some may inflate credentials). Some service providers have a mix of onshore and offshore capabilities. If a company is interested in building its own capabilities in the analytics and AI space over the long term, it should staff projects with a mixture of rented people and its own employees.
However, the people who are building analytics and AI applications are not the only important aspect of a talent strategy. People are also needed who have business analysis skills and can frame the business problem to be solved and do a high-level translation into what technologies might address it. Design thinking skills play an important role as well—both for user interfaces and the business processes to which analytics and AI will be applied. In addition, executives and managers who understand the value and purposes for these resources can “pull” them into their functions and processes. This can rarely be done successfully as a “push” from technologists.
Perhaps the most important component of a talent strategy is to encourage and help senior executives and business function/unit leaders to rethink how their businesses will work with analytics and AI. They may need to dramatically change their business models and processes to take advantage of these capabilities. A senior executive at a professional services firm noted, for example, that, “In the short term AI is cannibalizing our revenue. Therefore, our strategy is to figure out new sources of revenue.” This sort of conversation is clearly important enough to incorporate in the strategic discussions of a leadership team.
Change Management Strategy
Projects employing analytics and AI are not just about technical change, but also about changes in organizational culture, behavior, and attitudes. Approaches to change management may not have changed much over the years, but they may need to be called out in an analytics and AI strategy if they are not well understood or followed. Change management is important because analytics can be threatening to existing decision-makers, and AI often creates fears about automation and job loss. And since both often involve the management or application of knowledge and insights, they may be threatening to powerful and autonomous knowledge workers.
For example, for more than a decade, there have been systems based on AI that can detect potential cancers in medical images. Several studies have suggested that these systems provide more reliable diagnoses than human radiologists. Yet the systems are not widely used, have not replaced radiologists, have not lowered medical costs, and aren’t likely to do so soon even with improvements in detection accuracy. There are many reasons for this reality, but change management or the lack of it is certainly among them.
A broad description of the change management approaches that might facilitate successful analytics and AI projects is beyond the scope of this post. However, all of the usual approaches, including stakeholder analysis, readiness, communication, coaching, and training, are all relevant. A strategy for change management, should it need to be explicitly articulated, should focus on the types of behaviors that are being sought and the interventions planned to achieve them.
Summary
Analytics and AI are perhaps the most disruptive technologies and management approaches available today. They aren’t going away, and can confer significant competitive advantage to companies that exploit them successfully. Therefore, companies need to articulate a strategy for how they will introduce, develop, and manage these powerful capabilities. An implicit strategy is better than none, but an explicit strategy initiative covering a variety of components will both engage more participants and provide more direction to executives and employees.