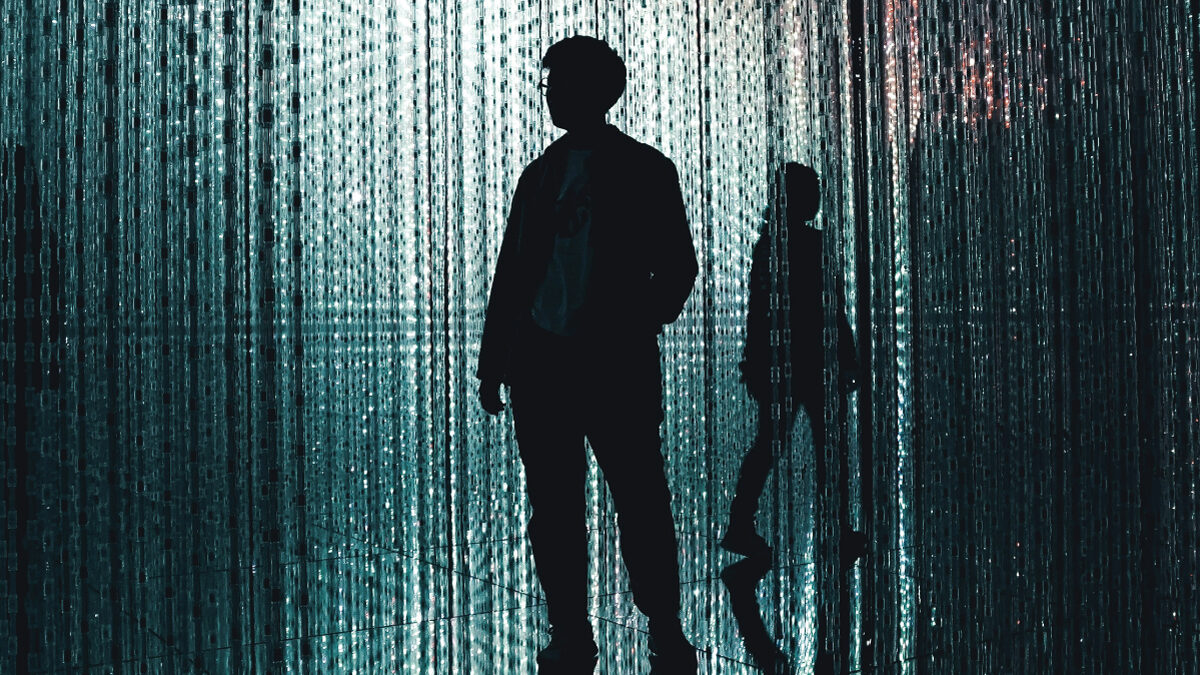
Imagine you’re the leader of the aftermarket data and analytics team at a large manufacturing company. You’re responsible for feeding insights into the digital tools used by your dealers and customers—tools that help them purchase extended warranties, manage parts inventory, improve uptime, and streamline service. Your team doesn’t run the tools themselves, but your data powers the features. And when data is missing, late, or inaccurate, everything breaks.
Now imagine trying to explain to a product team that you can’t generate critical insights for the business because a few key data elements weren’t captured when the latest application enhancement went live.
You knew what you needed. You asked for it. But somehow it didn’t make it into the build.
If that sounds familiar, you’re not alone. We hear versions of this story across industries: insight teams stuck downstream, trying to patch holes left by software development processes that weren’t built with data in mind.
That’s why it’s time to flip the script. It’s time to “design for data.”
The Problem: Data as an Afterthought
Most product teams are set up to serve their primary users—the ones who log into the tool, complete transactions, and interact with the interface. That’s understandable. But those aren’t the only users that matter.
Downstream teams—like yours—rely on the data those tools generate to power analytics, machine learning, reporting, and decision support. If the right data isn’t captured, you’re left with a broken foundation.
And the fix? It almost always falls on you.
In the absence of strong design-for-data practices, analytics teams resort to late-stage workarounds: stitching together missing fields, reconstructing timelines, inferring context, or rebuilding pipelines after launch.
The result is predictable: degraded data quality, limited insight, duplication of effort, and strained relationships between product and data teams.
Why This Happens: Functional Funding and Siloed Thinking
Most of these issues aren’t the fault of any one team—they’re the result of how IT and product development are structured.
Application teams are usually funded and staffed by functional units. They take direction from business stakeholders with specific operational goals. That funding structure shapes how work gets scoped, how requirements are gathered, and how priorities are set.
And unless your analytics team owns part of that funding or has a seat at the scoping table, your needs are often sidelined.
There’s also an organizational blind spot: many product managers and engineering teams simply don’t consider data as a design requirement. Their mental model of "the user" often excludes downstream personas like analytics teams, data scientists, or service and support functions. If they can’t see you, they won’t design for you.
So, how do you fix it?
Step One: Expand the Definition of "User"
Every digital product has more than one kind of user. In addition to the frontline end user (dealer, customer, technician), there are:
- Analytics and reporting users
- Service optimization teams
- Product managers who rely on usage data
- Regulatory and compliance stakeholders
- Other downstream systems that consume data
If your application development process only accounts for the first category, you're missing half the picture. And you're setting yourself up for downstream friction.
You need to be explicit: Designing for data means designing for all users, including the ones who never touch the screen.
One of the fastest ways to shift this mindset is to embed downstream personas in product planning. Just as UX designers use personas to represent their interactive users, data and analytics leaders should provide personas that represent data consumers.
Step Two: Build for Integration, Not Extraction
Traditional OLTP systems were designed to execute tasks: place an order, file a claim, submit a ticket. OLAP systems were designed to analyze. Historically, these two worlds were separated by design.
Today, that boundary is blurred. But the need for design discipline is greater than ever.
Data should not be a byproduct of transactions. It should be a product of thoughtful planning. That means thinking up front about:
- Which events need to be logged
- Which fields must be structured and standardized
- Which relationships between entities matter later
- Which data elements need to be traceable over time
Without that planning, you’re left retrofitting telemetry into systems that were never meant to be analyzed—or worse, fixing broken pipelines because the wrong data was overwritten or never captured at all.
As a rule of thumb: If it might be needed for insights, design to capture it now. Waiting for the perfect requirements means waiting too long.
Step Three: Treat Missing Data as a Bug
This is one of the most actionable changes you can make: update your product and engineering teams’ bug tracking processes to include missing or low-quality data as valid defects.
Why? Because missing data breaks functionality. Not for the primary user, maybe, but for downstream systems and reporting.
If a field is captured inconsistently, if a key identifier is missing, or if an event isn’t logged—that’s a bug.
Document it. Prioritize it. Fix it.
When product teams start treating data defects with the same seriousness as UI issues or functional regressions, you’ll see real progress.
Step Four: Design Governance into the Build
The worst time to discover a data quality issue is in the middle of a quarterly review or after a new feature has launched. Governance can’t be bolted on after the fact.
Designing for data means designing for:
- Ownership: Who is responsible for the accuracy and completeness of each field?
- Lineage: Where does each field originate, and how does it flow downstream?
- Access: Who can view, edit, or delete each type of data?
- Privacy and compliance: Are regulations like GDPR or CCPA being accounted for at the point of capture?
A practical approach is to bake governance into agile ceremonies: data requirement reviews, QA checklists, sprint demos, and user story definitions.
When data integrity is part of the definition of done, governance becomes everyone’s job.
Step Five: Use Artifacts to Make the Invisible Visible
One of the most effective techniques we’ve seen is deceptively simple: diagrams.
Not system diagrams. System + people + data flow diagrams.
Draw where the data originates, how it flows, who touches it, and who depends on it downstream. Include applications, services, personas, timelines, and ownership boundaries. Then share it.
This exercise surfaces the real complexity—and shows gaps that would otherwise go unnoticed until it’s too late.
Visualizing the full flow helps technical and non-technical stakeholders alike understand how small design decisions can create (or block) enterprise value.
Start Where You Are
You don’t need a new governance office or another steering committee to get started.
You can start today by:
- Embedding downstream data personas into your product planning process
- Documenting data defects as part of your QA and issue tracking
- Aligning your development and analytics teams on shared definitions of "success"
- Adding metadata capture and ownership to your sprint planning
You won’t fix every org structure overnight. But you can build better habits into the way your teams deliver digital products.
When you design for data, you design for insight. And when you design for insight, you build products that actually move the business forward.
Looking for more guidance on embedding data strategy into product development?
Explore our Data Strategy Resource Hub for more tools, checklists, and frameworks to help your team move from reactivity to readiness.