Need to design a data strategy that drives tangible business value? You’ve come to the right place. Download our free Practical Guide to Building a Business-Aligned Data Strategy to the right, or keep reading to answer the following questions:
Jump to section:
- What is data strategy, and why does it matter?
- Why do enterprises struggle with data strategy development?
- What key elements inform a modern demand-driven data strategy?
- What is data literacy and how does it turn data strategy into behavior?
- How do you turn data literacy into data fluency?
- What top data strategy questions are IIA experts fielding right now?
Practical Guide to Building a Business-Aligned Data Strategy

What is data strategy, and why does it matter?
Is your data strategy hindered by tech-first thinking?
Are you struggling with a lack of governance and stewardship?
Is your data architecture optimized for scalability?
Establishing a clear data strategy and strong data foundation is critical to making the difficult and necessary transition to advanced analytics and AI. Many enterprise leaders consistently identify gaps in foundational data competencies, particularly in areas like data capture, data quality, data integration, data consistency, and data trustworthiness. These core elements appear again and again in top priority lists in IIA’s Analytics Maturity Assessment clients and serve as the scaffolding for any successful data and analytics program. This hub explores how to strengthen those capabilities while embedding governance, improving data access, and ensuring your tools and architecture are aligned with business outcomes, not technical perfection.
Most organizations recognize that data is their most valuable asset, but too many struggle to use it effectively. Instead of unlocking business insights, AI-driven innovation, and operational efficiency, their data ecosystem is fragmented, unreliable, and underutilized.
If your enterprise is lost in a data fog—surrounded by information but unable to see clearly—this resource hub is for you.
Here, we break down the core challenges, best practices, and proven frameworks for designing a scalable, AI-ready data strategy that aligns with business objectives and drives real, measurable value.
Top 10 Focus Areas for D&A Leaders
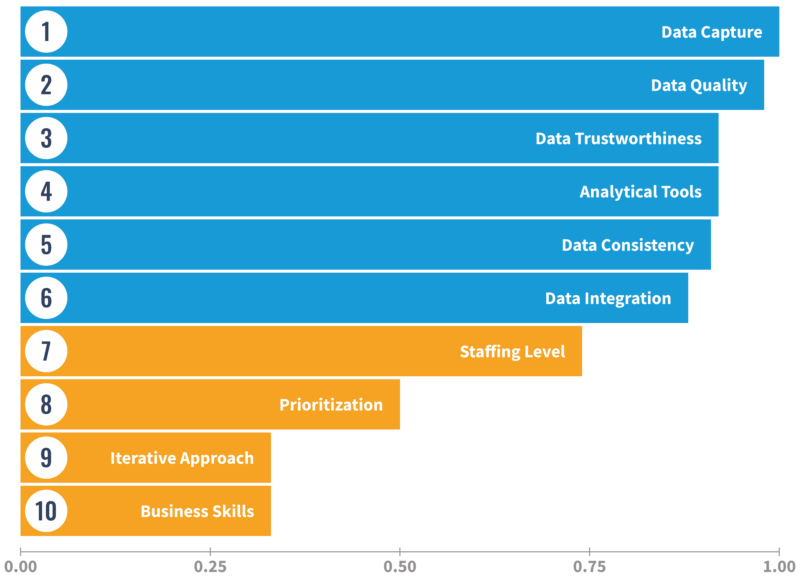

Most organizations today recognize that data is one of their most valuable assets—but that’s where the clarity ends. The reality is that data strategy failures are rarely due to technical limitations. They stem from misaligned priorities, fragmented ownership, and a fundamental misunderstanding of what a data strategy is supposed to do.
A common mistake is starting with the data supply side—thinking in terms of systems, tools, and platforms—rather than from the demand side of the information economy. Data strategies that begin with “What do we have?” or “How do we clean it?” end up generating elegant architecture slides but little real business value.
Here are the most persistent pain points we see across non-digital-native enterprises who are striving to transition to advanced analytics and AI at the enterprise level.
Why do enterprises struggle with data strategy development?
1. Strategy Without Alignment or Vision
Many organizations jump into data initiatives without first answering: “Why are we doing this?”
Data strategies are often disconnected from corporate strategy—or worse, treated as technical modernization efforts with no business case.
There's rarely a documented end-state vision, which makes long-term alignment and measurement impossible.
EXPERT EXCHANGE: What’s the right year-one strategy for launching a new D&A shared service? »
2. Governance Is Underdeveloped or Misunderstood
Most organizations still treat governance as a compliance exercise rather than a value accelerator.
Data ownership is ambiguous, and roles like stewards and curators aren’t clearly defined or empowered.
Without clear adjudication processes, decisions about access, quality, or compliance stall projects.
EXPERT EXCHANGE: What’s the best way to get started with data governance and data security? »
3. Data Quality and Consistency Remain Elusive
“Garbage in, garbage out” still rules, especially when AI and automation are layered on poor-quality inputs.
Different teams define core terms differently (e.g., “customer,” “revenue”), eroding trust across departments.
Data consumers are often forced to rely on manual patches or rogue workarounds.
BREAKTHROUGH CONVERSATION: Data Quality as a Forcing Function with IIA Expert Liz Marsh »
4. Architecture Is Fragmented and Over-Engineered
Tool sprawl, cloud migrations, and disjointed platforms create an illusion of modernization without true integration.
Too many organizations fail to articulate their theory of operations—how the platform will actually support real users doing real work.
There's little clarity around what’s prioritized for ingestion, who curates new datasets, or how quality tradeoffs are managed at scale.
EXPERT EXCHANGE: How should we approach governance and quality during a cloud migration? »
Data Principles According to Information Model:
Governance Foundation Cheat Sheet
Download this Governance Foundation Cheat Sheet to help you map data principles according to your unique information economy.
Extended Roundtable
Peer Insights:
Integrating AI Into Your Data Strategy
Download a discussion summary and read success stories and actionable strategies you can implement at your organization.

What key elements inform a modern demand-driven data strategy?
To escape the cycle of stalled modernization and tactical firefighting, organizations need to adopt a demand-driven mindset. IIA’s framework emphasizes building for the business, not just building infrastructure.
1. Define Business-Aligned Strategy and Vision
The first step is aligning your data strategy with corporate or analytics strategy. If it’s not tied to business priorities like growth, cost reduction, or risk management—it’s not strategic.
Key Takeaways:
Start with the demand side—what do data consumers need to succeed?
Define your aspirational end-state, not just in technical terms, but in terms of how people should experience data.
Socialize the strategy early and often with both IT and business leaders.
EXPERT EXCHANGE: How do you stand up a new team under a chief data officer (CDO)? »
2. Establish Clear Principles and Ownership
Rather than imposing abstract policies, IIA recommends establishing principles that resolve recurring conflicts—such as the tradeoff between data access and data quality.
Key Takeaways:
Decide whether availability or quality should take precedence in analytics environments.
Formalize lineage expectations and build them into your metadata and access platforms.
View stewards as customer-facing roles who serve both supply and demand side constituents.
EXPERT EXCHANGE: How do we formalize informal data stewards into a sustainable community? »
3. Invest in Data Quality That’s Fit for Purpose
The goal isn’t perfect data. The goal is usable, timely, fit-for-purpose data for each business need—especially in environments where analytics may benefit from “noisy” inputs.
Key Takeaways:
Prioritize transparency and metadata over perfection—know what you’re working with.
Create quality dashboards and anomaly detection systems that speak to business impact.
Build cross-functional quality councils that span business and IT.
EXPERT EXCHANGE: How should we approach selecting a master data management (MDM) tool? »
4. Design a Flexible Architecture with Operational Clarity
Data platforms don’t deliver value without a clear theory of operations—who does what, when, and how. IIA recommends pairing architectural choices with user-centric operating models.
Key Takeaways:
Focus on use cases to drive platform capabilities—not the other way around.
Define how metadata is captured, how lineage is traced, and how governance decisions are made within the platform.
Plan for both self-service access and controlled curation.
EXPERT EXCHANGE: Can we get expert feedback on a centralized data platform architecture?
5. Sequence Roadmap and Execution with Demand in Mind
Your roadmap should build incrementally toward the end state, guided by real business use cases. Think of each project as both a value delivery mechanism and a platform investment.
Key Takeaways:
Use high-impact use cases to justify foundational platform investments.
Solve for general capabilities (like ingestion, standardization) that can be reused across domains.
Revisit your strategy quarterly—it’s a living document, not a static slide deck.
Sample Theory of Operations – Logical Platform Model and User Roles
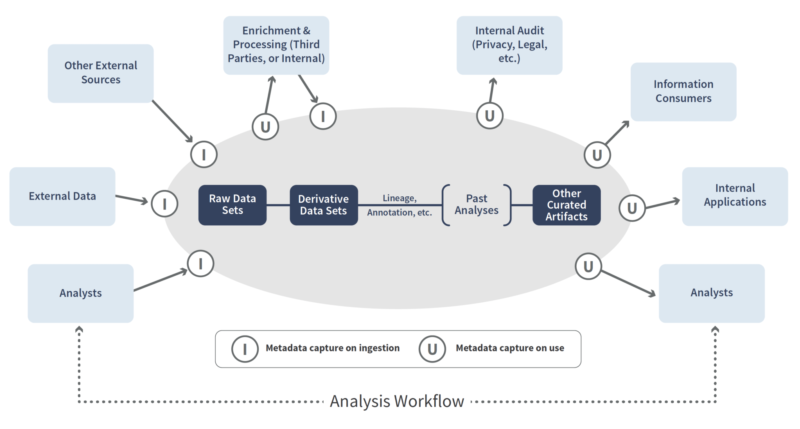

What is data literacy and how does it turn data strategy into behavior?
Even the most robust data strategy will stall without a workforce capable of acting on it. That’s why data literacy is the linchpin between your strategic intent and actual business impact. It enables people—not just platforms—to turn data into decisions.
Contrary to popular belief, data literacy is not just about teaching employees to read dashboards or use self-service tools. It’s about fostering a shared language of data across functions, clarifying definitions, elevating communication between technical and business teams, and aligning people’s day-to-day actions with enterprise data goals.
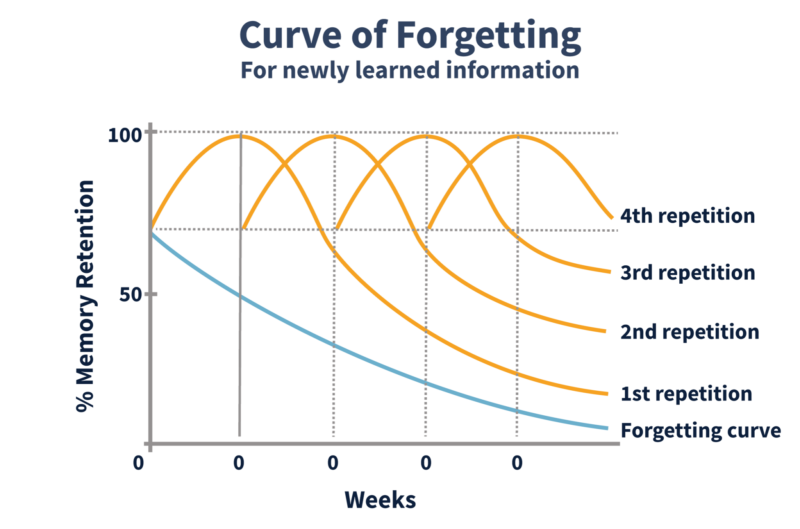
Why Data Literacy Matters Now
Data literacy becomes essential once data is widely available. Ultimately, data literacy is a second-order problem in that the ability to make use of data and analytics isn’t a problem until you have enough data and analytics available to cause a problem. In other words: if you're facing literacy gaps, you’ve succeeded in scaling data access, now it’s time to unlock its business value.
Learn MoreThe Role of Data Literacy in Executing Strategy
Accelerates Strategic Initiatives: Organizations that link data literacy programs to concrete initiatives—like churn reduction, AI deployment, or revenue growth—see faster alignment and stronger outcomes.
Builds Trust in Data Quality: Teaching employees how to question and interpret metrics fosters healthy skepticism, not paralysis. People stop asking, “Can I trust this data?” and start asking, “What does this data mean for my decision?”
Bridges Business and Technical Teams: True literacy is bidirectional. Business users must learn to ask better questions. Technical teams must learn to communicate in business terms. When both improve, adoption skyrockets.
BREAKTHROUGH CONVERSATION: The Emergence of AI Literacy with IIA Expert Mike Congdon »
BREAKTHROUGH CONVERSATION: Leveraging AI’s Visibility to Mature Your Data Literacy Program with IIA Expert Brian Kleinfelt »
EXPERT EXCHANGE: How can we strengthen our data literacy program for the core D&A team? »
What Effective Data Literacy Programs Include
IIA observes three critical layers where data literacy efforts should take root:
1. The Business Context
Connect programs to KPIs and active initiatives.
Enlist sponsors who understand how data fluency fuels strategic success.
Use literacy programs to reduce friction, confusion, and rework between teams.
2. The Individual Context
Design persona-based learning (e.g., apprentice, detective, whisperer, sage).
Provide job-relevant use cases and real-time application.
Support peer-to-peer coaching, especially among high-scorers on literacy self-assessments.
3. The Organizational Context
Build communities of practice that reinforce data behaviors.
Use systems, dashboards, and applications to reinforce definitions and support onboarding.
Measure progress through BI usage rates, sentiment surveys, and volume of training requests
Webinar:
Building a High Data Fluency Information Ecosystem
Get started on your journey from data awareness to data mastery. Join IIA Expert Ryan Sousa, former CDAO at Seattle Children's Hospital, as he explores the virtuous learning cycle of data fluency—a framework that transcends mere data literacy to foster continuous growth and innovation across enterprises.
Watch Now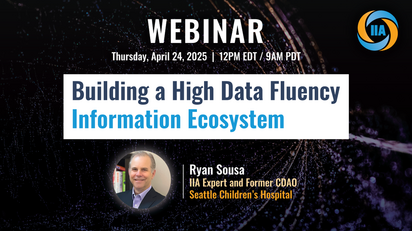

How do you turn data literacy into data fluency?
A successful data strategy doesn’t just modernize platforms—it changes how people think and work. If your goal is to create a more agile, AI-ready enterprise, you must make data literacy an operational competency, not a one-time training event.
That’s why forward-looking D&A leaders are embedding data literacy efforts into:
New hire onboarding
Analytics and AI upskilling programs
Quarterly strategic planning
Enterprise KPIs
Understanding Data Fluency
Building upon the practice of data literacy, it's crucial to explore the concept of data fluency, as articulated by IIA Expert Ryan Sousa. While data literacy focuses on the foundational ability to read and interpret data, data fluency encompasses a more advanced capability: the seamless integration of data into decision-making processes, fostering continuous improvement and innovation.
According to Sousa, data fluency involves the ability to swiftly exchange and explore ideas, take action, and evaluate results, thereby reducing the time from idea to action. This concept is central to the virtuous learning cycle, an iterative process where each decision, action, and evaluation builds upon previous experiences to drive ongoing growth.
In this cycle, as data fluency increases, the quality and speed of decision-making improve, accelerating the entire process. Automation further enhances this cycle, enabling near-instantaneous transitions from decision to action, which necessitates a high level of data fluency.
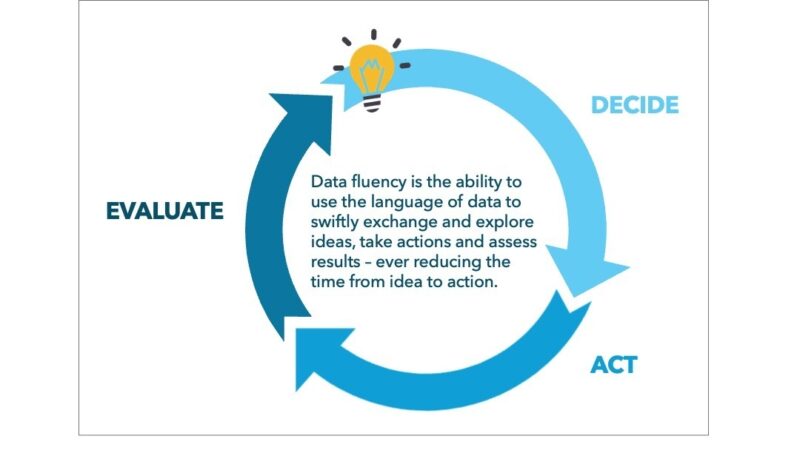
Building Blocks of Data Fluency
Sousa identifies four critical components that underpin a data-fluent organization:
Data Consumers: Individuals and teams who utilize data to inform decisions and drive outcomes. Highly fluent consumers go beyond static reports to explore data, identify patterns, and provide feedback to improve data quality and utility.
Data Producers: Those responsible for creating and maintaining data assets. They ensure data is accurate, accessible, and relevant, facilitating effective use by consumers.
Data Community: The collective culture and practices that promote data sharing, collaboration, and governance across the organization.
Data Platform: The technological infrastructure that supports data storage, processing, and analysis, enabling seamless interaction between producers and consumers.
The Journey to Data Mastery
Evolving from data literacy to data fluency is a progressive journey that enhances an organization's analytical maturity. We can outline this progression as follows:
Data Awareness: Recognizing the existence and potential value of data.
Data Proficiency: Developing the skills to analyze and interpret data effectively.
Data Fluency: Integrating data seamlessly into decision-making processes.
Data Mastery: Achieving a mature data culture where data informs virtually all significant decisions, driving innovation and competitive advantage.
Advancing Your Organization's Data Fluency
To dive deeper into building a high data fluency information ecosystem, watch this webinar hosted by Ryan Sousa, IIA Expert and former CDAO at Seattle Children’s Hospital. This sessios provides actionable strategies to enhance data fluency, including assessing and evolving the four building blocks critical to nurturing a culture of innovation and continuous improvement.
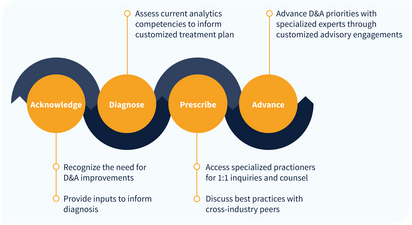

What top data strategy questions are IIA experts fielding right now?
Here’s what your peers are struggling with and how IIA’s Experts helped them make progress:
What’s the right year-one strategy for launching a new D&A shared service?
How do you stand up a new team under a chief data officer (CDO)?
What’s the best way to get started with data governance and data security?
How should we approach governance and quality during a cloud migration?
How do we formalize informal data stewards into a sustainable community?
How should we approach selecting a master data management (MDM) tool?
Can we get expert feedback on a centralized data platform architecture?
How can we strengthen our data literacy program for the core D&A team?