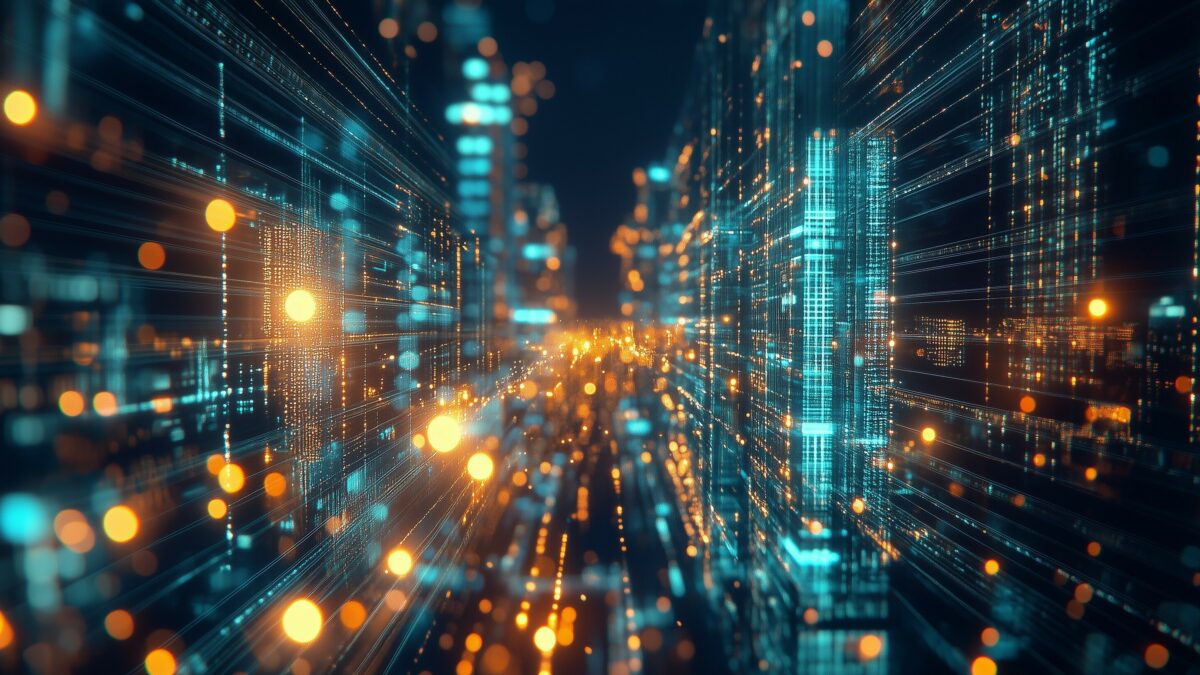
As 2025 unfolds, the attention given to artificial intelligence continues to spotlight an enduring challenge: data quality. Earlier this year, I shared five key insights from IIA’s Analytics Maturity Assessment (AMA), which validated top challenges for D&A leaders and underscored opportunities to be seized this year. Doubling down on data quality was a key theme. The truth is the current hype cycle around AI—namely large language models— has exasperated data quality challenges for enterprise D&A teams. In every assessment conducted over the past two years, data quality consistently ranks among the top priorities for improvement. Data quality is the pain that won’t go away.
But the data issues don’t stop there. In fact, the top six competencies that our clients address based on tailored recommendations and roadmaps flowing from their assessment diagnostics fall under the “data” category: Data capture, data quality, data integration, data consistency, data trustworthiness, and analytical tools to optimize a company’s data. These competency gaps are reflected in data that go back to 2018.
Top 10 Focus Areas for D&A Leaders
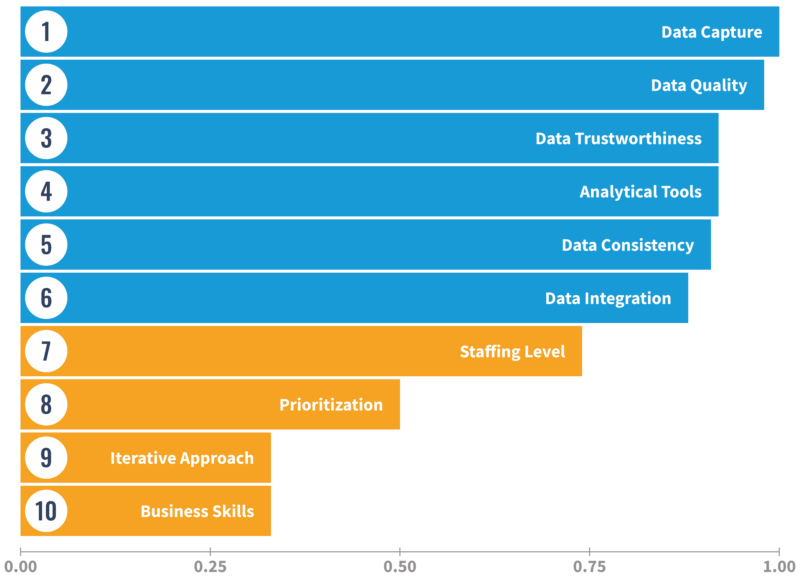
As every D&A leader knows intimately, the data pain is not new. And neither is AI. My deliberate use of “hype” in this piece’s title reflects a common misconception: that hot Silicon Valley companies like OpenAI invented artificial intelligence with the release of tools like ChatGPT. Of course, equating GPT with AI broadly is misleading. Media portrayals suggesting AI’s sudden emergence with public LLM usage—highlighted by tools like GPT—have unfairly pressured the D&A leaders we respect and support. Pressure from boards, CEOs, senior leaders to “just plug in AI” to solve all the business problems. If only it were that easy. We deeply empathize with the analytics community because—to add insult to injury—they are now having to (re)advocate and (re)educate the business about the fact that the company has been using “AI” for years in the form of machine learning and other advanced analytics techniques (techniques that have been lazily pegged as “traditional” by too many in the industry).
LLMs are an impressive example of deep learning. Deep learning is a subfield of machine learning. Machine learning is a subfield of the very broad and fuzzily defined practice of “artificial intelligence." Machine learning underpins the majority of AI—especially when it comes to leveraging data insights to improve operational performance, generate new revenue streams, and the like. Full stop.
This is not to say that IIA does not see real gains in experimenting with and implementing AI (including LLM techniques) to drive tangible business value. Many of our clients are doing very exciting things in this space and it’s been gratifying helping them plan, prioritize, and execute on their new AI initiatives. But we also see enterprises put the cart before horse, time and again. The trap of chasing shiny objects is real.
What may seem like a digression about AI is really a belief statement, if you will, that IIA holds close to its daily operations: In due time, LLMs will be replaced by the next AI craze, and we will go through another round of speculative euphoria (and hysteria) ad infinitum. Case in point: see the stirrings of anti-cloud operations on TDS. That’s what tech progress (or fluctuation) is all about. Going all the way back to Tom Davenport’s Competing on Analytics, first published in 2007, the bedrock for analytics success, from descriptive to prescriptive to creating new data with deep learning, is having a strong data strategy and flexible data ecosystem.
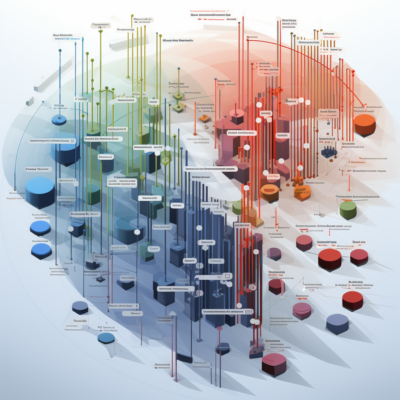
Webinar: Building a High Data Fluency Information Ecosystem
Get started on your journey from data awareness to data mastery. Join IIA Expert Ryan Sousa, former CDAO at Seattle Children's Hospital, as he explores the virtuous learning cycle of data fluency—a framework that transcends mere data literacy to foster continuous growth and innovation across enterprises.
For IIA, the driving force for your data strategy must be demand driven. In other words, the reality is that data strategy failures are rarely due to technical limitations. They stem from misaligned priorities, fragmented ownership, and a fundamental misunderstanding of what a data strategy is supposed to do. A common mistake is starting with the data supply side—thinking in terms of systems, tools, and platforms—rather than from the demand side of the information economy. Data strategies that begin with “What do we have?” or “How do we clean it?” end up generating elegant architecture slides but little real business value.
This is where leveraging the AI hype comes in. In the same vein as IIA Expert Brian Kleinfelt’s approach of leveraging AI’s visibility to mature your data literacy program, a similar mindset can be used for the creation—or reimagining—of your data strategy. If the corporate strategy doesn’t provide obvious points of connect with “analytics” or “data,” and you don’t have an analytics strategy, then you probably shouldn’t be doing a data strategy. If your company wants an “AI strategy” before you’ve solidified a demand-driven data strategy, then you most definitely should not be “doing AI”—and rather get started on your data strategy today.
In short, there is no better time to build a business case for investment in data-related challenges. If your CEO wants AI, what they really want is a solid data strategy and foundation. This is the ticket to an analytically-driven operation.
Further, establishing a demand-driven approach to enterprise D&A is the stickiest door that many of our clients must pass through to realize the potential of their programs. That is why we developed the market’s most robust assessment that is solely focused on demand, and not supply, which is a far more common analytics maturity assessment tool—and only tells one side of the story. A majority of our 1:1 Expert client guidance focuses on demand-side challenges. In the end, adoption is all about building a deeper bond with the business, and doing the hard, “soft skill” work that goes into analytics engagement.
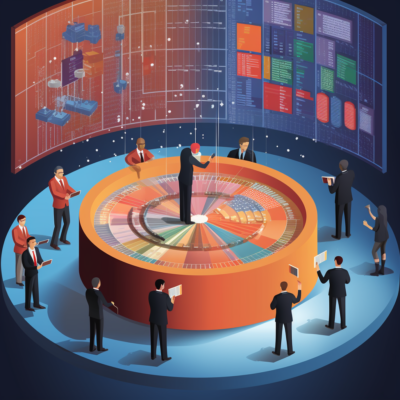
Analytics Maturity Assessment
Our industry-leading diagnostic tools and tailored solutions harmonize data supply and demand, helping you drive alignment, adoption, and ROI.
That is also why we created this resource hub for the data and analytics community to help them create a strong demand-driven data strategy. Bookmark this page and refer to it often as we will be adding insights and practical guidance over time, including our foundational step-by-step data strategy framework and additional key takeaways from 1:1 Expert exchanges. One of my favorite resources is the governance foundation cheat sheet, a tool that can help you map data principles according to your unique information economy.
Fear not D&A leaders, we’re tracking AI trends as closely as everyone else, but what really gets us up in the morning is supporting your organization with the foundational aspects of D&A success—starting with refining your company’s data strategy.