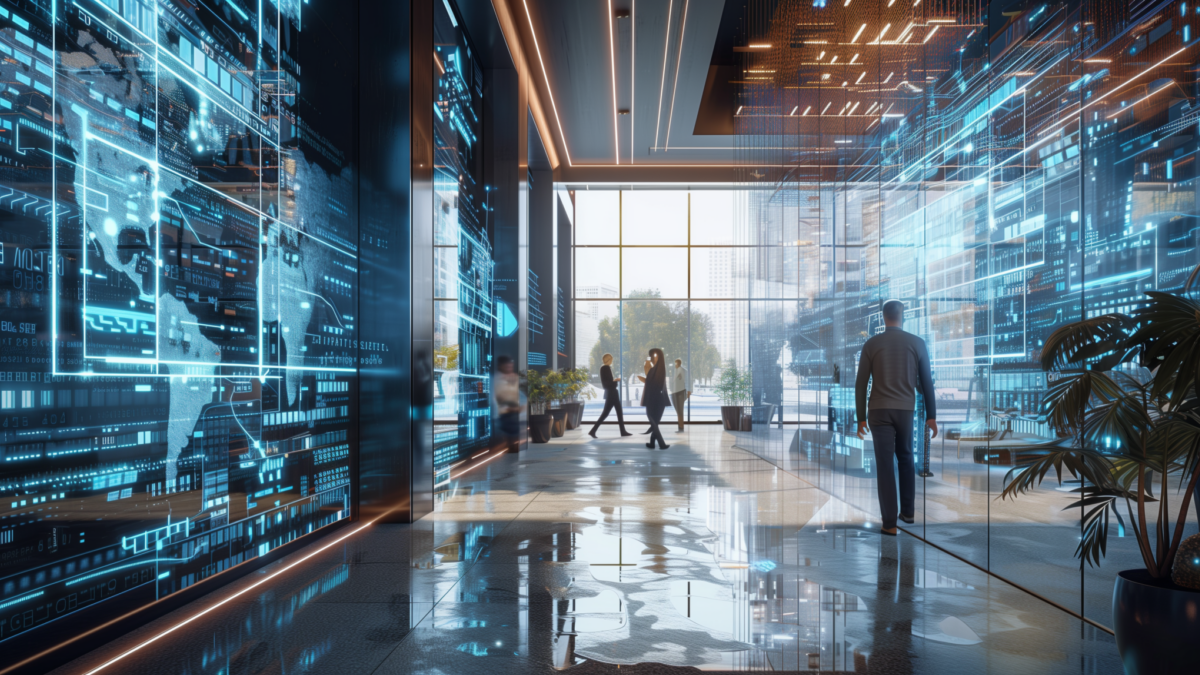
A few years back, IIA created a framework for clients to assess the critical dimensions of a data-driven culture. This framework supports data and analytics leaders who leverage our diagnostic tool called the Data-Driven Culture Inventory. With this inventory, IIA creates a customized survey to identify and characterize key elements of an organization’s data-driven culture. The assessment results provide critical cultural insights for driving analytics and AI solutions, training, career development, hiring, and planning the cultural transformation required for data-driven decision-making.
As data and analytics leaders grapple with AI change management, it’s clear in our interactions with clients that using a foundational framework is an indispensable resource in asking the right questions and considering trade-offs. More to the point, we’ve observed a steep learning—or comfort—curve for many legacy enterprises who’ve historically sidelined analytics as a niche (read siloed) investment. Indeed, using the term too casually overlooks the complex relationship with data and the extensive change management efforts required for enterprises to advance in data-informed decision-making or become truly analytically driven.
In businesses where data isn't the main driver, decision-making can be influenced by intuition, misinterpreted conventional wisdom, and confirmation bias. On other side of the spectrum, a data-driven culture is guided by the data itself—decisions, outcomes, and strategies hinge on what the data reveals, not just what leaders want to hear. This approach means following the story told by the data, even when it challenges existing beliefs or desired results.
Three Ways to Relate to Data
Organizations typically align with one of three stances regarding data's role in decision-making:
- The Way of the Caveman: Here, data doesn't guide decisions. Leaders fixate on what they want, disregarding data that may suggest alternative actions.
- The Way of the Advocate: In this approach, leaders engage with data but cherry-pick information that validates their preconceived plans, overlooking contradictory evidence.
- The Way of the Scientist: This method is rooted in an iterative, hypothesis-driven approach. Decision-makers gather data, hypothesize, test, and refine their strategies continually until they find the most effective path forward. Organizations that follow this model invest heavily in data to inform and improve business decisions.
The journey to becoming a data-driven organization entails recognizing your current cultural stance and either reinforcing it—if it mirrors the scientist's way—or transforming it if it aligns with the caveman’s or advocate's way.
The benefits of adopting a data-driven culture are many and most organizations have at least acknowledged this fact. Still, it’s worth reminding ourselves the impact. Organizations that adopt, reinforce, and reward the way of the scientist and use data to drive decision-making and action-taking:
- Are better aligned with their customers and sales, supply chain and other channels
- Deliver better products and services
- Manage suppliers and trading partners more effectively
- Avoid overstaffing functions that don’t add value, and understaffing functions that do
IIA’s research shows the value in these outcomes. Among our findings, data-driven companies have shown 2% sales, 5-50% cost savings, and 6% higher profitability.
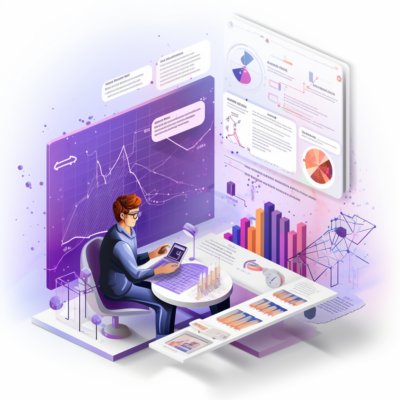
Dimensions of a Data-Driven Culture eBook
This overview of IIA's Data-Driven Culture Framework includes descriptions of seven key dimensions for organizing, growing and maintaining a world-class data-driven culture
Seven Key Dimensions to Becoming Data Driven
In IIA's years of client engagement, we've consistently observed seven key dimensions for organizing, growing, and maintaining a world-class data-driven culture. These dimensions haven’t changed with the newest wave of AI buzz. Among these, cultural norms and personal orientation stand out. Cultural norms are the communicated values of an organization regarding data's role in its operations. Personal orientation addresses how individual employees view data's importance in decision-making. Ideally, there's alignment—data-driven individuals thrive in data-centric organizations. However, mismatches between personal and organizational orientations toward data can lead to frustration and negatively impact performance. Understanding where your organization and its members stand on these dimensions is an important step in driving a data-oriented transformation.
- Cultural Norms: Corporate cultures can implicitly and explicitly influence decision-making. For instance, companies may declare their dedication to data-driven practices, yet if leaders don't model these values in their actions, a gap between aspirational and operating culture manifests. Employees notice these discrepancies and adapt their behavior accordingly. For true cultural shift toward data-driven decision-making, the focus should be on the actual incentivized behaviors within the organization. Trust, transparency, and collaboration become crucial, especially in mature organizations where debates shift from data accuracy to the nuances of its interpretation. Establishing a common ground for interpreting data is a necessary step, as it involves open dialogue and consensus-building.
- Personal Orientation: Our personal values and inherent biases deeply influence how we handle data in professional settings. Common decision traps like confirmation bias, resistance to change, sunk-cost fallacy, and favoring intuition over data often hinder our ability to make rational decisions. By understanding the actual behaviors and attitudes toward data within your organization, you can better navigate the transformation toward rational, data-driven decision-making. This understanding is key for refining approaches across all aspects of the organization, from technology to human capital.
- Technology Enablement: Technology enablement in organizations gauges the readiness of infrastructural conditions to support data-driven decision-making. This readiness includes quality data that is fit for purpose, easily accessible through intuitive tools, and delivered timely to inform decisions. A recurring issue is the lag in making this data readily online; often, by the time it reaches decision-makers, the optimal moment for action has passed. Like the advent of the dial tone in telephones sending a signal that could talk to anybody, anywhere in the world, organizations today have widely varying “data tone.” Few have achieved the level of technology enablement required to support the full range of routine operational and tactical decision-making in their organization. For many decision-makers, there is no workable “data tone.” And yet decisions must be taken.
- Decision-Making Skills: The rise of business intelligence has mistakenly assumed that access to data means that people will know what to do with it. However, without proper training in decision sciences—which is often missing outside elite MBA programs—employees are not equipped to leverage data effectively. Critical decision-making skills include risk assessment, data evaluation, decision modeling, hypothesis testing, selecting measurable actions, effective communication, and management of execution. Lacking these skills, organizations often miss the most important step: measuring the actual outcomes against projections. Decision-making capabilities must be widespread, as the hierarchical model of senior management decision-making must give way to agile, distributed decision-making at all organizational levels. In today's landscape, where decisions are increasingly data-informed and tools begin to aid decision-making processes, it's essential that employees are trained to make structured, measurable, and actionable decisions. This demands a combination of training, mentorship, and assessment tools to ensure organizational competency in data-driven decision-making.
- Leadership Behavior: In IIA’s experience, the single most motivational factor driving data-driven cultural change is the behavior of decision-makers’ immediate leaders. No matter how optimized the culture or how advanced the technology, if leaders don't embody and reinforce data-driven values, the initiative fails. When decision-makers face rejection from leaders despite clear data-driven recommendations, it sends a message that the data-driven approach isn't valued, leading employees to adapt their behavior to conform to the behavioral cues of their local leadership. For a culture to be truly data-driven, leadership at all levels must consistently and explicitly support and engage in data-driven decision-making practices.
- Peer Behavior: Cultivating a peer environment that supports mutual coaching and healthy confrontation regarding decisions is pivotal in fostering a data-driven culture. Just as employees follow the lead of their supervisors, they also align with the expectations and behaviors of their colleagues. Peers who encourage and hold each other accountable for data-driven decision-making can create a positive competitive atmosphere, often resulting in more sustainable progress than top-down mandates. A decision-maker might hesitate to critique leadership directly but can influence their peers by insisting on data-backed arguments, suggesting alternate interpretations, and scrutinizing proposed actions. Unfortunately, many opt for silence over speaking out, often due to a belief that the prevailing peer dynamics don't support healthy interactions when high-risk decisions are in play. Examine how peers in the organization interact with each other. Look carefully at the extent to which individual decision-makers feel comfortable calling out their peers for behaviors that aren’t data-driven and rewarding their peers for behaviors that are.
- Human Capital: Even with all dimensions aligned for a data-driven culture, if the company's reward systems do not incentivize data-driven behaviors, then employees will naturally gravitate toward what benefits them financially. Compensation and bonuses need to align with the strategic emphasis on data-centric decision-making. Without this alignment, employees will both note the cognitive dissonance and continue to be guided by their compensation plans. Human resources play a pivotal role, too. They must weave data skills and decision-making competencies into job roles, hiring practices, and development plans, affirming that an organization's commitment to data is reflected throughout the employee lifecycle—from recruitment to retirement.
Minimum Thresholds
The seven dimensions we've covered set the stage for a cultural transformation toward data-driven decision-making. However, failing to meet minimum capabilities in any one of these can undermine the entire effort. For example:
- Human Capital: If compensation practices do not support data-driven behaviors, employees will not adopt them. People naturally optimize for what benefits them financially.
- Leadership Behavior: Leaders who disregard data in favor of intuition or who act recklessly set a tone that can hinder cultural change. If leaders don't value data-driven analysis, those in the organization with data skills may either disengage or depart.
Get Further, Faster
To accelerate a transformation into a data-driven organization:
- Invest in Technology: If resources permit, prioritize technology that supports consistent data availability. Empower decision-makers with tools for business intelligence to foster a hunger for advanced analytics and AI. As decision-makers become sufficient in descriptive business intelligence, they seek tools and methods for forecasting and shaping future outcomes.
- Develop Skills and Encourage Peer Influence: Independent of budget, focus on enhancing decision-making skills and cultivating positive peer behaviors. Success in certain business areas can attract attention and encourage broader organizational adoption.
- Recruitment: While altering personal beliefs is challenging, organizations can refine their hiring practices to attract individuals with orientations toward data and strong decision-making skills.
- Examine Cultural Norms and Compensation: These areas might not be the first step unless they're actively obstructing data-driven initiatives. If compensation schemes conflict with data-driven goals, they should be reevaluated promptly.
Addressing these areas can lead to faster progress in creating a culture where data-driven decision-making is the norm.
Final Thoughts
It’s one thing to say, “We should be data driven.” It’s another to take action. Taking action begins with following the way of the scientist:
- Assess where you are now in terms of the seven dimensions.
- Hypothesize the results of implementing a reasonably small set of changes. If implemented correctly, these changes should produce measurably better outcomes.
- Implement the changes and measure again. Repeat.
Cultural transformation doesn't happen overnight. It demands time, investment, and dedication. The goal is to foster a culture where data-informed decisions become the driving force. With the increasing significance of analytics and AI in the business landscape, companies that master this analytical edge gain a sustainable competitive advantage.