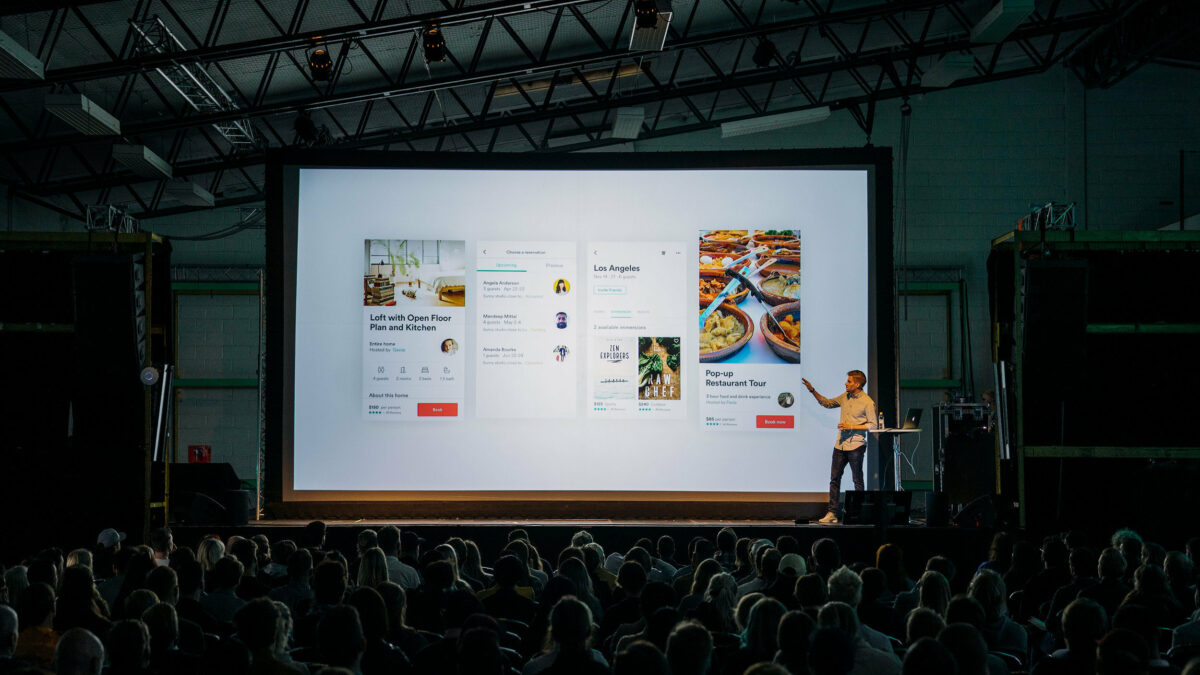
There are a lot of skills necessary to be a successful analytics or AI leader within an organization. You’re probably familiar with them; you need quantitative skills, business acumen, tech and programming capabilities, yadda, yadda, yadda. You have heard these over and over again, and my guess is that if you’re reading this you already possess many of these skills.
But I work with and speak to a lot of people in these roles, and some have the necessary skills and intelligence but are still not very successful. So I thought I would provide a list about attitudes and behaviors that often make analytics and AI leaders unsuccessful, at least in businesses. Some might be appropriate for a university or a think tank, but even there I suspect some will find them obnoxious. You may get away with some of these in talking to your own staff, but that will only encourage them to exhibit similar behaviors, which will in turn make them unsuccessful.
Here, then, is a list of attributes to avoid if don’t want to be viewed as an analytical jerk and if you want to be successful in analytical leadership roles:
1. Don’t try to make yourself look smarter than anyone else. Nobody likes a smartass, even if you are being paid a lot to solve wicked problems with cutting-edge methods and tools. There are many sub-principles of this general one; here are a few:
a. Don’t refer to esoteric terms, tools, or ideas. Don’t toss around phrases like “gradient descent” or “eigenvectors” or “posterior distribution” unless you are confident that a regular businessperson is nowhere nearby.
b. Don’t talk about what happens at prestigious academic institutions. “Have you read about the latest work from the Computational Genomics group at MIT CSAIL?” is not a question to be asked in polite company.
c. Don’t discuss what is happening at very advanced technical companies unless you already work at one. Saying things like “I was talking to some of the guys at DeepMind about their latest work on protein folding” will not endear you to anyone in the real world.
2. Don’t describe the work you have done in terms of the technical or methodological steps you have taken to accomplish it. If you say things like, “There was some heteroskedasticity in some of our variables, so we had to do some serious feature engineering and ended up with a sinusoidal function,” most of your audience will have tuned you out by the time you finished your sentence. All that business people want to know is that you can or can’t solve their problem or answer their question; they don’t care about the process you followed. As one analytics leader said to me years ago, “Nobody cares about your R2 or what you did to maximize it.”
3. Don’t communicate that you care much more about whether the analytical or technical problem is interesting and novel than you do about solving the business problem. It may be that generating a bar chart in Excel is all you need to do to shed light on a business problem or issue, and showing disdain for that activity will get you nowhere.
4. Don’t pretend that you understand something that you don’t. The analytics and AI profession is sufficiently complex that no one can understand everything about it. If you say, “I don’t understand that—can you explain it?” then people will respect you more, not less. It suggests that you are confident and secure in what you do understand.
5. Don’t assume that solving the problem is the most important aspect of an analytical problem—or more accurately, a decision-making or business problem. Any such problem has a number of steps, from framing the problem in the best possible fashion, to gathering data, to solving the problem, and then implementing the solution and delivering results. Solving the problem is often one of the easier steps in this process. The fact that you are not really in charge of the other steps doesn’t make them unimportant. And if the other steps don’t happen well, solving the problem is not very useful.
As the estimable Karl Kempf, the long term head of Decision Engineering at Intel, told me many years ago, the successful use of analytics in decisions is not about the math, but rather about the relationship between the quantitative analysts and the decision-maker. If the quants try to exalt themselves and their intelligence over everyone else, they will lose the trust of the decision-maker and their analytics won’t have an impact.
The most successful analytical leaders I have met or worked with are the most modest and self-effacing. They don’t need to be the smartest person in the room, or to impress you with their academic credentials. Their goal is to make a difference within their organizations, and for the most part they succeed.