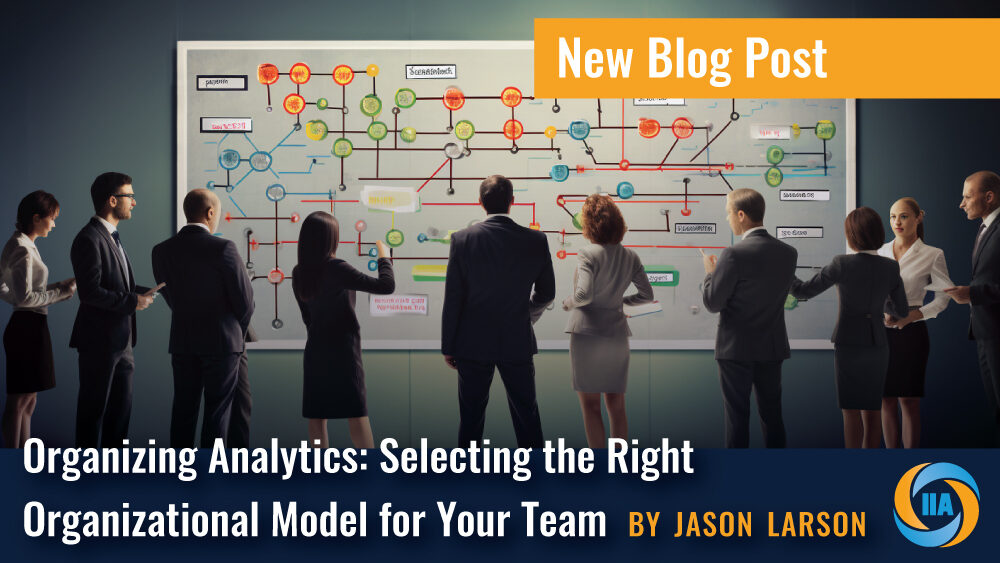
This article is the introduction to a series on IIA’s observations and guidance around organizing analytics. IIA has researched how best to organize analytics and data science teams for over a decade, and a lot has transpired since our initial writings. Big data has gone from novel to norm, data science talent is in higher demand than ever, artificial intelligence has taken the world by storm, and real-time analytics and automated decisions embedded in business processes are ubiquitous. These individual trends don’t cause wholesale change to the structure of the analytics and data science organizations, but when combined, they do lead to the need for some adjustments. This article discusses the fundamental goals and objectives of organizational structure, a new continuum-based approach to organizational models, and guidance determining where your organization falls on the continuum.
How to Organize Is a Difficult Question
One of the most frequent questions when IIA discusses organizations’ analytical efforts with our clients is: “How should we best organize our analytics and data science resources?”
The reality is that there isn’t one structure that fits all organizations. In fact, the right structure for any given organization will likely vary over time. In other words, while at any given time there may be a model that makes the most sense for a company, it should be expected that there will be changes necessary as time passes, business conditions change, and analytical requirements evolve.
Let’s illustrate by examining a company going through a typical evolution over time. The company initially started with analytical talent scattered across the enterprise in pockets where business teams saw a need. As is common, marketing was one area that dipped its toe into analytics early for the company. Other teams saw how marketing was succeeding with analytics and added their own teams. However, as the company continued to add more pockets of analytics talent, it began to cause problems. The company realized that these pockets needed to be coordinated to ensure scalability, efficiency, and consistency. This led to a centralized model where all analytics and data science resources were brought together. Over time, the company began to slowly disperse talent back out into the company’s business units while maintaining a linkage to a core central team. Most analytically mature companies end up with some type of hybrid model. With that said, the specifics of how the hybrid organization is organized, its reporting structure, and its span can change over time and necessitate further tuning.
Regardless of the structure under consideration or in place at your company, it is important to focus on ensuring that current analytical requirements can be met; that your talent pool has a variety of work to challenge it and a clear set of career paths to pursue; and that there is sufficient governance and standardization of tools, policies, and methodologies across the enterprise.
Objectives of an Organizational Structure
In addition to facilitating basic employee administration functions, organizational structures serve several fundamental objectives:
- Deploying people on the most important and value-adding projects in support of the enterprise’s business processes and requirements is about doing today’s work and maximizing people’s contribution. Resource deployment is complicated by the necessity to staff both local business unit projects and cross-functional or enterprise-wide projects.
- Developing people so they will have the skills and experience for tomorrow’s work is about maintaining the capability and health of the organization and maximizing people’s individual and collective potential. Development is complicated by the need for both business acumen and technical skill in analytics staff.
- Maintaining the wide range of analytical processes that are built ensures that they remain accurate, available, and up to date. Maintenance is complicated by the ever-changing business requirements, changes to existing data sources, incorporation of new data sources, and refinements of the toolsets and architectures in place.
These objectives are interrelated because the most important learning and development occurs on the job, and those who build a process understand best what maintenance is required. But they are in opposition whenever there’s a choice between:
- Deploying someone to apply their proven skills to an important new project;
- Having them help maintain and tune existing processes; or
- Giving the person a stretch assignment to acquire new skills.
Classic hierarchical organizational structures tend to bias toward keeping people in their “boxes.” Yet analytical talent is in high demand today, and many people will not stay with an organization unless they perceive ample variety in their jobs and opportunity to learn new things continually.
Organizational design therefore boils down to two big questions:
- What’s the best way to group people for purposes of deployment, development, and maintenance?
- What are the best ways to coordinate across groups since no individual group will be perfect for all needs the organization has?
Additional Considerations
In addition to the critical questions above, there are several other core considerations that should be addressed in order to find the correct organizational model for any point in time:
Demand Management
How will the organization maximize stakeholder engagement, alignment, and value? How will it gather, prioritize, and implement requests from stakeholders?
Analytics Maturity
What is the organization's overall analytics maturity and what challenges must be addressed for the company to further mature? What is the organization ready for today and what structure will best enable it to prepare for tomorrow?
Analytics Depth and Breadth
From business intelligence to advanced analytics to complex AI/ML models, and to fully embedded operational models, what is in place today and how can those investments be put to best use and then expanded over time?
Relationship with IT
Who will own the data and platforms that support the analytics? Who will maintain the models and analytical processes that are in production? Who will determine and apply governance and security policies? These must be clearly documented and agreed upon.
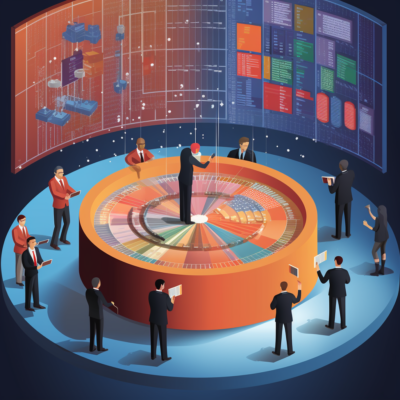
Analytics Maturity Assessment
Find out how you can get in-depth diagnostic insights, recommendations, and benchmarking on your enterprise analytics maturity, your analytics organization capabilities, and the skills of your individual contributors.
The Organizational Structure Continuum
In the past, IIA focused on six common organizational models that we saw in the marketplace. In this update, we’ve shifted focus to a continuum within which all of those models, and others, fit. This is because it has become clear that mature, successful analytics organizations almost always end up organized with some form of a hybrid model after passing through variations of one or both of the decentralized and centralized model structures. The remaining companies stick with a centralized model. We’ll still call out where the old models lie on this continuum, but we think the focus should be on the continuum as opposed to any specific model within it. The graphic illustrates this new view, including where the individual models fit.
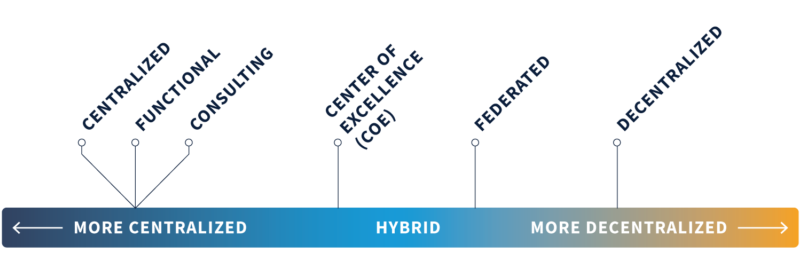
Centralized Analytics Models: Resource Deployment and Skills Development
Centralized Model Defined:
A centralized model involves the consolidation of all analytics and data science resources within a single organization within the enterprise. This organization could be situated at the corporate level, supporting the entire company, or it may be embedded within a forward-thinking business unit, especially in less mature companies.
Variations of Centralized Models:
Beyond the traditional centralized model, the consulting model fits within this organizational approach, since it’s simply a centralized model where funding is based on demand and a project-by-project basis as opposed to a fixed funding source. The functional model, while a form of centralization, is the least mature model since it implies that only one part of the business has meaningful access to analytics. Virtually no large companies remain with a functional model in place because, by definition, analytics are not a corporate-wide activity.
Strengths of a Centralized Model:
- Resource Deployment: Centralized control facilitates the deployment of team members on high-priority strategic projects, often spanning multiple functions.
- Skills Development: It allows for coordinated skills development, including emerging areas like AI, which can be shared across business units.
- Talent Attraction: As the centralized group expands its capabilities, it becomes an attractive hub for recruiting new analytical talent.
- Incentivized Support: In functional variations, the home business unit funds the team, ensuring strong support and effective utilization.
Weaknesses of a Centralized Model:
- Distance from Business Units: Centralization can create a disconnect between analytics teams and the business units they serve, leading to feelings of underservice.
- Lack of Access: Functional models limit access to analytical talent for units outside the home unit, hampering effective analytics utilization.
- Funding Instability: In consulting models, funding cuts during tough times can destabilize the analytics organization.
- Misaligned Priorities: Consulting models may prioritize projects with funding over high-value initiatives, which can harm struggling business units.
When a Centralized Model Makes Sense:
- Corporate Culture: Organizations with a strong history of centralizing support functions can successfully implement centralized models.
- Service Providers: Service providers offering analytical support to external clients often use consulting models, with funding from clients.
- Stabilized Funding: An internally focused consulting model can work if it can secure stable funding through effective selling of capabilities.
- Startup Analytics: Functional models are common during initial analytical capability building but are not recommended as a long-term solution.
- Small Companies: Centralized models are ideal for smaller companies or those with a limited focus on analytics due to resource constraints.
The centralized model offers several advantages, such as effective resource deployment and skills development. However, it comes with challenges like potential disconnection from business units. The choice of whether to adopt a centralized model depends on an organization's culture, goals, and size, making it crucial to weigh the strengths and weaknesses carefully before implementation.
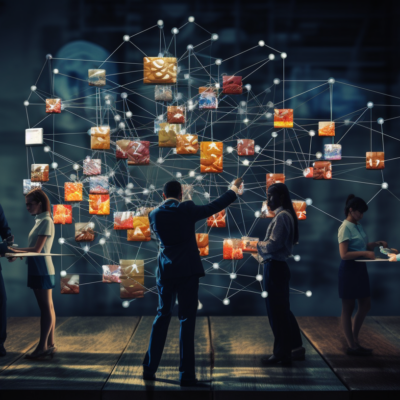
Organizing Analytics and Data Science Organization eBook
Formally and intentionally organizing your analytics teams is an integral part of any organization's analytics journey. Additionally, planning out how your company should eventually re-organize its analytics teams is also foundational to growing its capabilities and analytics maturity. Get tips from experts at IIA on how your team should be organized from the eBook below.
Hybrid Analytics Models: Balancing Innovation and Scale
Hybrid Model Defined:
In a hybrid model, analytics and data science teams are dispersed throughout various business units or functions within an organization. Crucially, a central, corporate team also exists, providing strategic direction, standardization, and support to the distributed teams. This central team can either hold direct authority over the distributed teams or function as their peer.
Variations of Hybrid Models:
COEs within a hybrid model address specific corporate needs such as AI or analytical technology enablement, catering to cross-functional requirements where resources are limited. In a federated model, the central organization supports other units with various skill sets. It may involve a voluntary leadership council at the outset but typically evolves into a structure led by an executive from the central team. COEs may also be part of the central team. This model is common in large, mature organizations.
Strengths of a Hybrid Model:
- Innovation and Scale: Hybrid models facilitate innovation and scalability. COEs within the central team can explore new areas before disseminating knowledge widely.
- Corporate Projects: The central team enables the pursuit of corporate projects spanning multiple business units.
- Standards and Training: It ensures corporate standards for tools and platforms and provides training and support. Employee mobility across teams enhances career development and satisfaction.
- Senior Roles: Growth in the organization leads to the creation of senior roles, benefiting career development and retention.
Weaknesses of a Hybrid Model:
- Lack of Power: In pure COE models, COEs often lack formal power and rely on relationships and influence. In federated models, decision-making can be challenging due to the absence of a single decision-maker, potentially leading to stalemates.
- Career Progression: Career progression may be hindered in smaller teams without multiple layers of management. Limited visibility outside one's home unit can limit opportunities.
- Communication: Effective communication and coordination driven by the central team are critical for realizing the full potential of distributed teams.
When a Hybrid Model Makes Sense:
- Shift from Dispersed Groups: Hybrid models enable a transition from independent, dispersed, and uncoordinated groups to a more cohesive structure.
- Scale and Capability: Large, analytically mature companies often adopt hybrid models to support both scale and breadth of capability.
- Adaptability: Hybrid models are easily modifiable to align with changing business needs, such as transitioning from voluntary coalitions to formal power structures.
- Leadership Roles: When there is a Chief Analytics Officer (CAO) or Chief Data Analytics Officer (CDAO), they often sit atop the central team within a federated model, making it a common structure.
It's important to note that hybrid models may not be feasible for smaller organizations due to resource limitations. These models thrive when there is a sufficient scale and a desire for long-term commitment to analytical capabilities. Smaller companies may find a more centralized approach more suitable for their needs.
Decentralized Analytics Models: Responsiveness and Flexibility
Decentralized Model Defined:
In a decentralized model, analytics and data science resources are distributed across various locations within the company, lacking formal coordination. Each team operates independently, detached from others. Although many organizations initiate their analytics journey with this model, few remain in this state over the long term.
Mapping to Traditional Decentralized Model:
The decentralized model aligns with the traditional decentralized model discussed in past research by IIA. Some argue that the functional model belongs here instead of on the centralized end because it involves a single team chartered by a business unit in the absence of corporate strategy. Decentralization implies multiple uncoordinated functional teams rather than a single team.
Strengths of a Decentralized Model:
- Proximity to Business Function: Teams in a decentralized model are closely aligned with the business functions they support, ensuring high responsiveness and dedication to their respective functions.
- Flexibility for Business Units: Business units can independently establish teams without requiring permission or alignment with other parts of the company. Few barriers exist for initiating standalone programs.
Weaknesses of a Decentralized Model:
- Lack of Corporate Support: The model lacks mechanisms to support corporate or cross-unit requirements, limiting the realization of initiatives valuable to the entire company.
- Redundancy: Teams individually invest in tools and solutions, leading to redundant costs and efforts. Solutions developed by one team may remain unknown to others.
- Divergence: There is potential for divergence in tools, methodologies, and strategies across teams, which can be detrimental to the company's overall effectiveness.
- Limited Career Opportunities: Team members have limited opportunities for career advancement as they are confined to their small teams and lack internal resources for collaboration or learning from other departments.
When a Decentralized Model Makes Sense:
- Early Analytical Journey: A decentralized model is suitable for companies in the early stages of their analytical journey. It allows a few business units to independently demonstrate the value of analytics while laying the groundwork for transitioning to a different model once a strategy is developed and value is proven.
- Subsidiaries within a Parent Company: In cases where a parent company owns multiple distinct subsidiaries, each subsidiary can choose its organizational structure independently to maximize its business. Private equity firms and global conglomerates often encounter this scenario. While there may be a centralized team within the parent company, it typically has limited scope, and subsidiaries operate largely independently. This decentralized approach aligns with each subsidiary's distinct needs.
The decentralized model offers proximity and flexibility but comes with limitations in terms of corporate support, redundancy, divergence, and career opportunities. It is most suitable for companies in the early stages of their analytical journey or those with multiple distinct subsidiaries seeking independence in their organizational structures. However, for typical organizations, a decentralized model is not an effective long-term solution.
In our next article in this series, we’ll explore the common paths organizations take as they evolve their analytical and data science capabilities over time. For additional detail on the common organizing models, download our eBook above and let us know how we can help you organize your analytics team for sustained success.