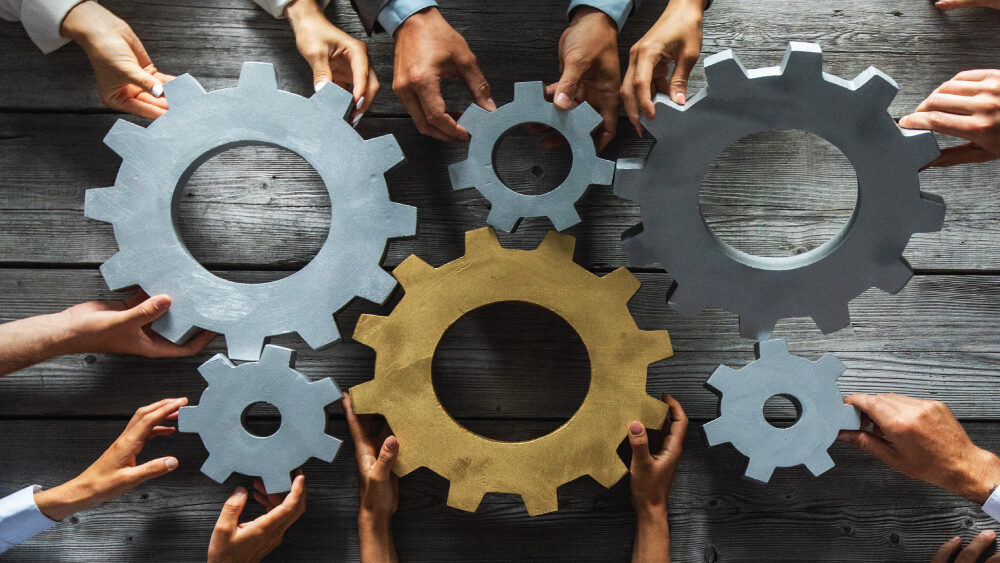
The Inequality in the Data Science Industry
Data science as a discipline has received an increasing amount of attention in the public consciousness over the past decade from many, including undergraduate students considering lucrative career paths and leaders of companies and organizations looking for efficient tools to inform or automate important decisions. While focusing on data science may build one’s tech career or optimize a company’s service, we have largely failed as a discipline to acknowledge one role data science plays wherever it’s practiced: When someone practices data science, they are either challenging or enforcing an existing structure of power.
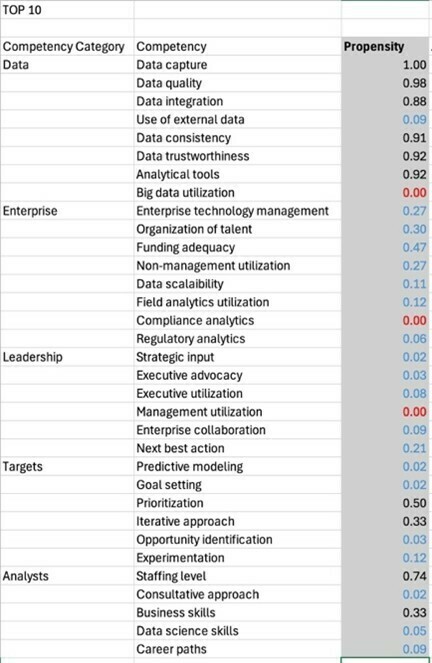
This dynamic plays out on both individual and collective levels in our society: On a collective level, it’s incumbent on organizations to fill their data science teams with data scientists who represent a well-rounded palette of diverse backgrounds and life experiences (and that’s no easy task right now). On an individual level, it’s every data scientist’s responsibility to understand their place in relation to human-made systems of power so that they don’t unwittingly act as oppressors through their practice.
Like any tool, data science isn’t inherently good or bad. It can be used to improve health for millions of people, or it can also reinforce racism and amplify inequality. Many of the harmful applications of data science occur within an industrial context: industrial data science, as opposed to academic data science. Industrial data science is ultimately data science in service of profit, while academic data science is data science in service of knowledge. In its current state, industrial data science is vastly more influential in the building and maintenance of our society than its knowledge-driven counterpart because practicing data science is costly for organizations and can only be achieved with sufficient resources.
Today, industrial data science is practiced through processing immense amounts of data to create patterns of automated decision-making called models. These models ultimately provide automated decision-making capabilities, saving an enterprise time and money. On its face, industrial data science has proven to be a powerful way to build useful customized product experiences or optimize the inner workings of an organization. We have industrial data science to thank for efficient food delivery apps, search engines, and automated recommendations of all sorts, to name a few benefits.
Representation Within the Data
Often, practitioners of data science say that generating a model is a way to predict the future, a common and dangerous misunderstanding of what a model does. Rather than predicting the future, a model projects the past into the future. With every automated decision, the rules of the world in the past are reproduced in an instant of data processing that in turn shapes the future. The result produces information that will inform tomorrow’s newer model. In this way, we’ve constructed an industrially-driven cycle of data processing that iteratively and blindly acts on itself. This means that the data we use to construct models are shaped by the construction of subsequent models in this repeating cycle.
Choosing a dataset wisely is one of the most important decisions a data scientist can make when training a new model. Often the goal is to generate a model with high predictive accuracy. To create an accurate model, a data scientist needs to find data that best correlates with the need of the model they intend to train.
Here’s an example: A data scientist at a financial institution is tasked with training a model to predict clients’ credit scores. Their next step is to comb through available sets of data about existing clients and determine among all this data, which information will likely be useful in training a model that gives credit score predictions with the most accuracy.
If the data used to train the new model considers gender or a feature correlated with gender as one of its variables, the new credit score model will likely have detected a pattern that females on average make less money than their male counterparts and have lower credit scores, since this is reflected in the real-world customer data. As an unintended and harmful result, the new model will predict a lower income for a woman than a man if all other factors are equal.
While this result may increase the accuracy of the model’s credit score predictions given the reality of the gender pay gap, the model by itself has no means of recognizing its unfairness. The culpability rests upon the data scientist to choose data and a model that will not generate unjust biases in the future. Looking at their credit score model’s output is looking at the status quo from the past, so there is a danger if the financial institution then relies on the model to generate customers’ credit scores: It will result in sexist predictions that will impact people’s lives in reality. This will produce new real-world data shaped by the biases baked into the credit score model. All of the data from the past is based on the imperfect picture of reality we have in the present, and the cycle continues when it’s used negligently to shape the future.
Some real-world examples can be found in the book Weapons of Math Destruction by Cathy O’Neil. She identifies examples of the short-sighted decision-making that lead to models with encoded racism or other biases. Those examples include models predicting teachers’ quality risk, creditworthiness, and recidivism.
For instance, O’Neil describes how the recidivism risk model was trained to predict how likely convicted criminals would re-offend in the future. The model would score a higher risk if a person is unemployed, has had encounters with police before, or came from an over-policed neighborhood. While those trends are evident in real-world data, the model does not have the complexities of context built into it — in this case, the police encounters often happen due to racist policing practices that disproportionately target Black and Latinx Americans. As a result of the systemic racism that is reflected in the data, the model recommended people of color get undeservingly longer sentences in prison.
Data scientists working on this project did not have enough awareness about the way in which racial biases in the criminal justice system are reflected in the data they used to train their recidivism model.
This illustrates how data scientists who grasp the mechanics of their work, but not the work’s context, may not have enough information to do their jobs effectively.
Within the landscape of industrial data science, a data scientist is often an interchangeable person who knows how to work with data to develop models, rather than someone with a specific background in the subject matter of the given data. This is what leaves data science as a discipline so blind to its effects. As a result, data scientists and their employers often fail to consider their context within systems of power, which ultimately perpetuates systemic inequities.
First identified by researchers Joy Buolamwini and Timnit Gebru, they discovered an accuracy disparity in commercial gender classification models used by big tech companies like Microsoft, IBM, and Face++ and published a paper on their findings. Their work showed that the images in the dataset used by U.S. tech companies for their models were more than 77% male and more than 83% white. As a result, when Buolamwini, a Black woman, tried uploading photos of herself, those commercial facial recognition programs misclassified her gender or failed to recognize her photos as a human face at all. The lack of diversity represented in the data resulted in an ineffective model.
Representation Within the Workplace
If data scientists use datasets that reflect systemic biases or lack a sufficient level of diversity, the resulting models will too. Having someone in the room who is aware of the data itself can greatly reduce the likelihood that it will be used ineffectively or unjustly. When teams consist of people from one homogeneous group, the perspective is limited by their range of lived experiences and exposure to other viewpoints. Without adequate diversity in lived experience, homogenous groups of people are prone to inadvertently making decisions to the exclusion of other identities and perspectives.
There is an increasing chorus of voices calling out on this issue of representation in the world of industrial data science. For example, Dr. Gebru, the other author of the aforementioned gender classification research with Joy Buolamwini, was later a co-leader of Google’s Ethical A.I. team. There, she openly critiqued how most of the people making the ultimate AI-related decisions at Google are men. She was later fired by the company after criticizing its approach to minority hiring and the biases built into today’s artificial intelligence systems. “They are not only failing to prioritize hiring more people from minority communities, they are quashing their voices,” she said.
For an organization, practicing data science is a privilege because it requires a lot of costly physical and computational resources. Neither a mom-and-pop shop, a creative entrepreneur starting out of their garage on a new enterprise nor a struggling non-profit has the resources to leverage data science. There are immense costs associated with obtaining, maintaining, storing, and accessing enough data for use in training a model, and then further costs in computational resources necessary to apply this model in practice. The only kinds of organizations that can practice industrial data science are wealthy: either large-enough corporate enterprises, powerful governments, or particularly well-endowed academic institutions.
The result is an imbalance of power in favor of the minority who owns the data and decides how it’s used. Industrial data science is practiced with an eye for optimization rather than comprehension. All too often, data science is used as a means to automate decisions within a system rather than to more deeply understand those decisions and their contexts. This is dangerous because it leads to short-sighted decision-making that fails to check its effect in the world, beyond whether or not an immediate, (often) profit-driven goal was achieved. It ultimately emphasizes the particular needs and worldviews of those who architect data science-driven systems.
The same biases that created such inequitable datasets have also shaped the values of individuals in the world who can practice data science in the first place. These systemic biases have inadequately filtered out people due to a host of possible reasons, from race to gender to class, and beyond. According to the U.S. Equal Employment Opportunity Commission (EEOC), around 80% of executives, senior officials, and managers in tech are male, and around 83% are white. As for data scientists in the industry, according to Zippia’s data science demographic analytics, 65.2% of all data scientists are men, and when it comes to racial statistics, 66.1% of all data scientists are reportedly white.
What can we do?
Data Feminism by Catherine D’Ignazio and Lauren F. Klein created concrete steps to action for data scientists seeking to learn how feminism can help them work toward justice, and for feminists seeking to learn how their work can carry over to the growing field of data science. . Data feminism is a way of thinking about data, both its uses and limits, that’s informed by direct experience, a commitment to action, and intersectional feminist thought. The authors discuss the problematic nature of how data science work is often a solitary undertaking. Letting one person make decisions for all without representing intersectional voices can introduce biases in the work and potentially harm unrepresented voices. One of the principles of data feminism is to embrace pluralism, which means valuing and including voices who have a connection to a dataset, in all stages of the data science process.
It is important to acknowledge two important problems. Data science is not just a quantitative field when it comes to applications related to humans. With the above examples of data science applications, it is clear that data scientists responsible for designing those systems lack the ability to detect harms and biases in their systems once they’ve been released into the world.
Representation within the workplace leads to representation within the data. Both concepts are interconnected. Conversely, representation within the data can ultimately contribute to a society that yields a more egalitarian hiring pool.
References
1 Buolamwini, Joy, and Timnit Gebru. “Gender Shades: Intersectional Accuracy Disparities in Commercial Gender Classification.” https://dam-prod.media.mit.edu/x/2018/02/06/Gender%20Shades%20Intersectional%20Accuracy%20Disparities.pdf.
2 “DATA SCIENTIST DEMOGRAPHICS AND STATISTICS IN THE US.” Zippia — Find Jobs, Salaries, Companies, Resume Help, Career Paths and More, https://www.zippia.com/data-scientist-jobs/demographics/. Accessed 28 May 2022.
3 D’Ignazio, Catherine, and Lauren F. Klein. Data Feminism. MIT Press, 2020. Accessed 28 May 2022.
4 The New York Times. “Google Researcher Says She Was Fired Over Paper Highlighting Bias in A.I.” 2020, https://www.nytimes.com/2020/12/03/technology/google-researcher-timnit-gebru.html.
5 O’Neil, Cathy. Weapons of Math Destruction: How Big Data Increases Inequality and Threatens Democracy. Crown, 2016. Accessed 28 May 2022.
This blog was originally published in Towards Data Science.