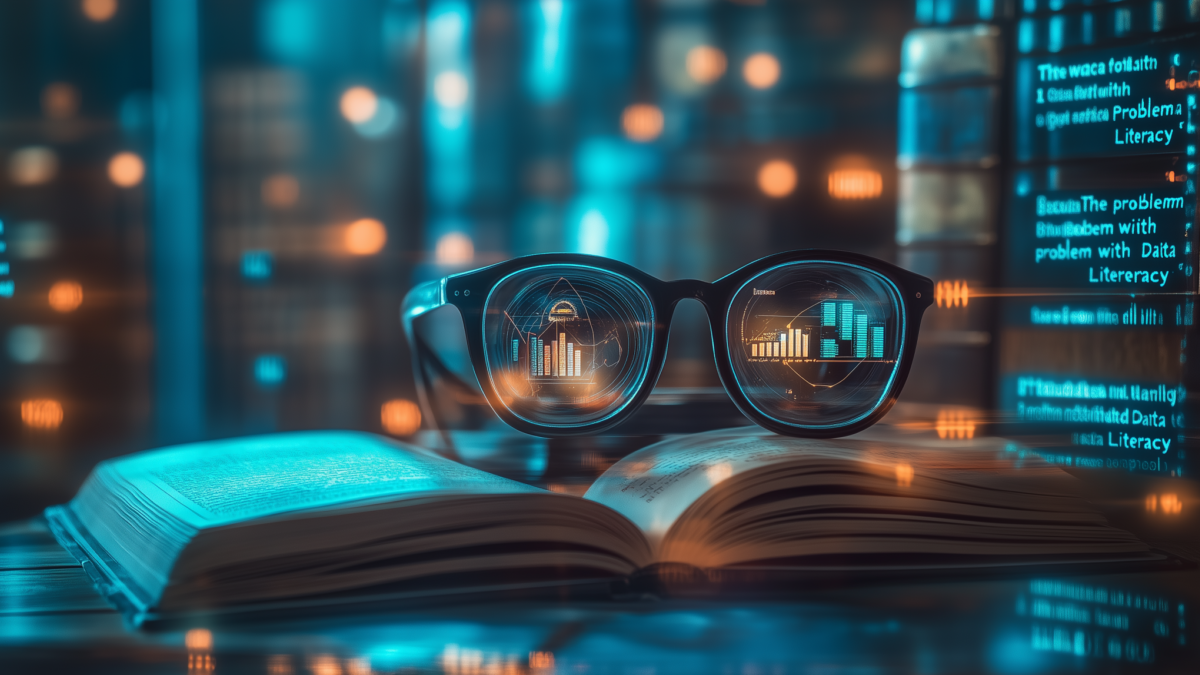
Data Rich, Information Poor
Gartner defines data literacy as “the ability to read, write and communicate data in context, with an understanding of the data sources and constructs, analytical methods and AI techniques applied.” Moreover, it’s the ability to describe the analytical use-case application and resulting business value or outcome. That’s a nice definition, but there are a couple of gaps in it. Firstly, the ability to read data is not the same thing as the ability to understand the information being conveyed by the data, leading us to the paradoxical observation that many organizations are “data rich, but information poor.” Secondly, the ability to communicate with data misses out on the important aspect of collecting data, leading us to a glaring gap in data instrumentation.
During my consulting, I’ve seen organizations put together data literacy training programs that focus mainly on Excel, Tableau or Power BI—tools to manipulate, visualize and interrogate data. These are no doubt useful technical skills to acquire, but they fall short of the larger objective of making someone “data competent.” A large swath of the working population is still data incompetent! Compounding the problem further, organizations now mix data literacy with digital literacy in an attempt to align it with the digital revolution. The American Library Association’s (ALA) Digital Literacy Task Force defines digital literacy as the ability to use information and communication technologies to find, evaluate, create, and communicate information, requiring both cognitive and technical skills. The ALA’s definition of digital literacy echoes that of data literacy, and this has obviously created some confusion for the learner. A number of researchers have begun to classify data literacy as a sub-domain of digital literacy. I tend to disagree. The domains intersect but don’t necessarily nest within each other.
Data Sensemaking
Both data literacy and digital literacy have a cognitive dimension in their definitions, whether implicitly or explicitly. I argue that the foundation of this cognitive dimension is
“data sensemaking,” which is an articulated competency. Data sensemaking is simply the ability to apply a frame or perspective to interpret meaningful information from the data and shows how the various data elements relate to each other. This frame would also allow one to develop various initial hypotheses on the opportunities or risks represented in the data. Data sensemaking is the starting point to the chain of events to derive insights—i.e., information that can improve decision-making; this requires a comprehensive understanding of the decision ecosystem. I’ve written an introduction on decision science and plan to give this topic due justice in a subsequent article in this series.
Data sensemaking is one of those competencies that’s never taught in school, and rarely deconstructed and taught in corporate L&D. We take it for granted that humans are born with this competency. But in my experience, I have seen little evidence for such a claim. Many learners are instead taught that data and information are just synonyms for each other, or they believe that the information contained within the data is deterministic and objective, or that you need more data to get more information. All of which are untrue. I have written about this topic in a prior post entitled “Information is NOT in the Data,” so I won’t repeat it here again.
Suffice to say, the ability to connect the dots when reading data is intimately wrapped up with what the dots can mean. The more we can appreciate multiple perspectives, the more connections we can make across dots. And the ability to appreciate multiple perspectives can only come from exposing oneself to a diversity of domains and experiences.
Data Instrumentation
Let’s look at the other major gap in the current approach to data literacy, which is around the ability to collect data. There is an exponentially growing need to know how to source for the right data. With digitalization of our solutions and processes, we are faced with a loss in cognition that accompanies the removal of the man in the middle, so to speak, and this cognition loss needs to be replaced by clever data instrumentation. Consider the example of Amazon Go, a chain of convenience stores that are cashier-less and fully automated, with customers able to purchase products without being checked out by a cashier or using a self-checkout station. Customers simply walk into the store, grab what they need, and walk out. Clever sensors have been installed to not only identify customer purchases and to debit their e-wallets accordingly, but to also monitor their aisle-browsing behavior, which is the observational data that is lost with the removal of the human cashier. Knowing how customers navigate and forage for products across the aisles allows Amazon to find-tune its product shelf placements to improve the customer experience and increase basket value.
Many of us today are involved in designing, delivering or supporting digitalization projects. The ability to figure out what data is required to proactively react to unplanned customer friction or to continuously improve the solution is therefore a critical competency. We need to be able to translate our human observational prowess (i.e. human data collection) into machine-equivalent data points. That is the essence of data instrumentation.
The need for data instrumentation as an emerging skill is something that organizations are becoming aware of. I’ve had the recent pleasure of working with a client to co-create a methodology for data instrumentation that starts by ascertaining the underlying assumptions and uncertainties in their existing and proposed solutions or processes, and to employ data instrumentation as a means to either validate those assumptions or reduce the uncertainties.
Conclusion
We need to rethink our approach in what it means for our workforce to be data literate in an increasingly knowledge-skewed economy. A tool-based approach has not yielded us the desired outcome thus far. We are largely missing the cognitive components in understanding the nuance and purpose of data. Data sensemaking needs to be at the very foundation of this enhanced literacy, strengthened with the ability to supplement our situational awareness with robust data instrumentation.