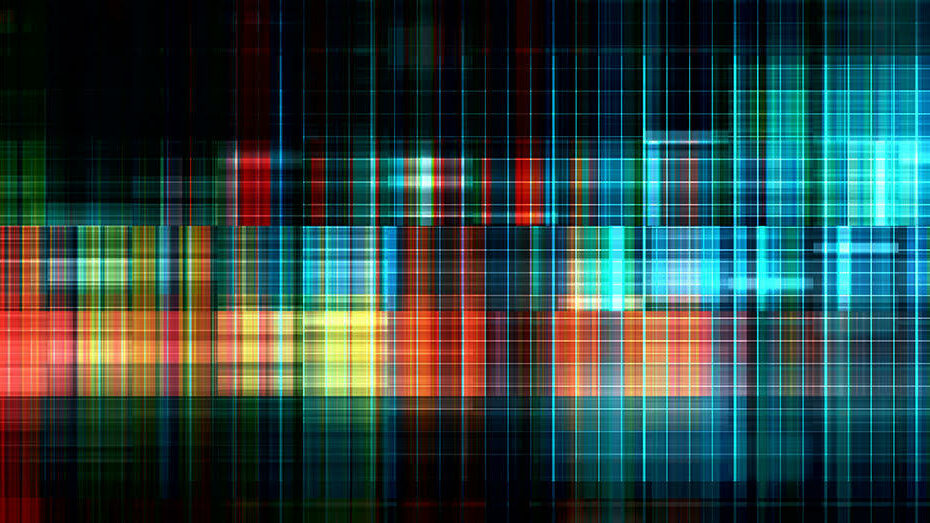
In 2018, IIA published a client-only research brief titled “Organizing Analytics.” The piece begins with how far analytics has come in recent years – the ubiquity of “big data,” the demand for data science talent, analytics in the cloud – and then proceeds to diagram and discuss the pros and cons of the six basic organizing models that still ring true today: Centralized, Functional, Consulting, Center of Excellence, Federation, and Decentralized. It’s a thorough, thoughtful paper that A) underscores the evergreen nature of the basic organizing models; B) reveals the fact that each of these models is basically centralized or decentralized; and C) argues that the mantra driving any structural change should be: Do what makes things easier.
In our previous Leading Analytics Podcast blog, we distilled the leadership qualities of high-performing CDOs, CAOs, and CDAOs into six core mindsets. The purpose of this exercise was to call attention to the unique skills, temperament, and backgrounds present among today’s analytics leaders that make for an entirely new pedigree of leader. In this blog, we’ll share both common and innovative approaches that data and analytics leaders are taking to organize their teams for success.
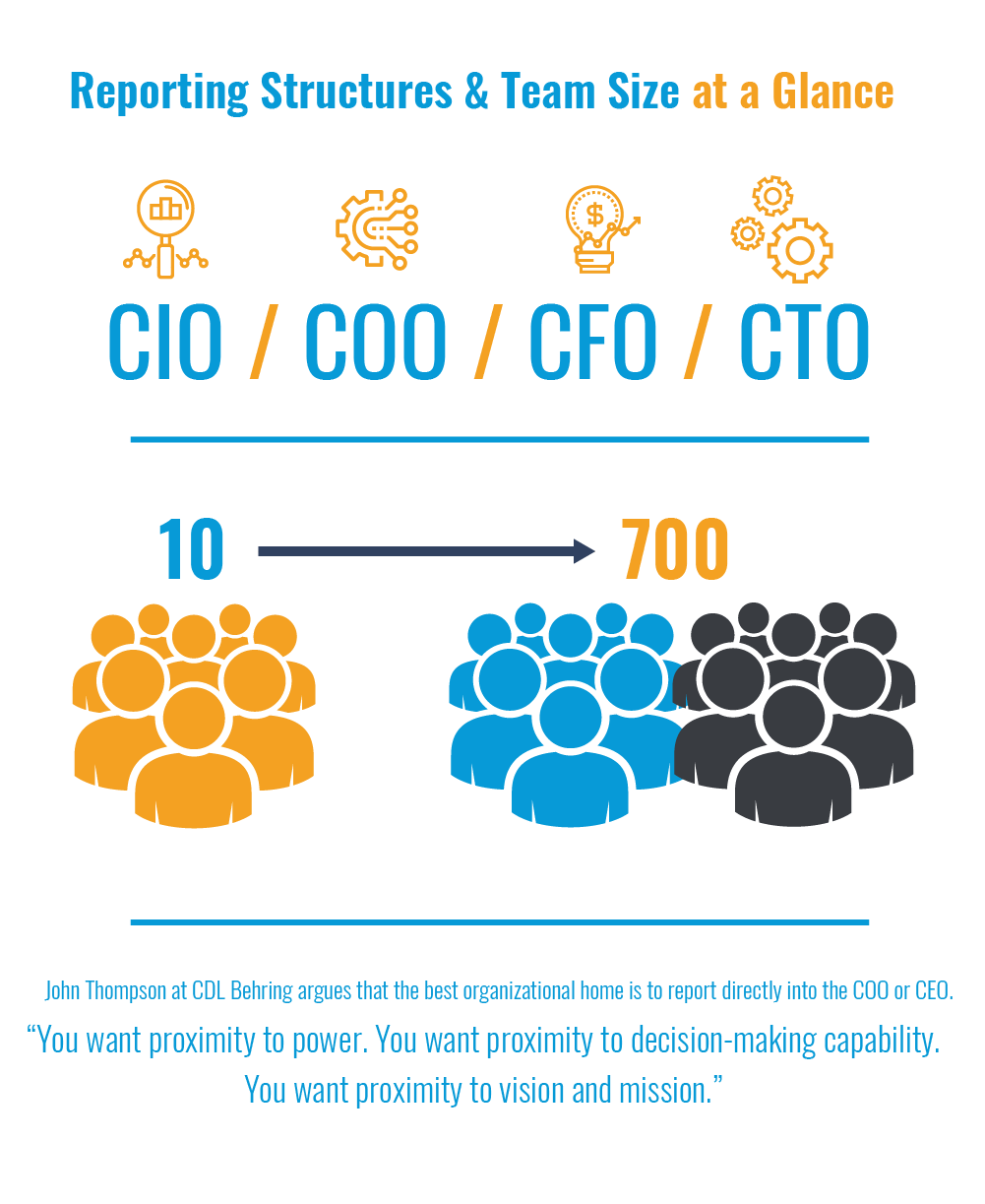
The Elusive Magic Bullet and Fundamental Questions
First, let’s address the elephant in the room. There is no magic bullet when it comes to organizing your analytics team. But there are a few fundamental questions that you should ask when building a team from scratch or embarking on a reorg. First, why have structure at all? What are you trying to achieve in the act of organizing your analytics team? What are you aiming for? If you’re trying to build enterprise solutions, then a centralized model is your blueprint. If you’re aiming for agility, then a federated model is most likely your best path. And what do your people get in the model that you create? This may be the most important question of all. Are you trying to build a community of data scientists or something else entirely?
To coalesce these questions into organizing principles at a team level, John Thompson, Global Head of Advanced Analytics at CDL Behring and author of Building Analytics Teams, described two main organizing principles around the data science practice in a recent ALC discussion: You either organize it as a group of artisans or artists, or you organize it like a factory. The artisanal approach brings together a small team of data scientists who take on every aspect of an analytics project – from drawing up the requirements to interfacing with the IT department to getting models into production. With the factory approach, you have a couple of people doing data ingestion, a couple doing data integration, and so on. According to Thompson, either structure works, but the organizing principle you choose should be driven by organizational culture. At CDL Behring, Thompson has moved to a hybrid model with contractors and vendor partners taking over the mechanical, repetitive work like data ingestion and data integration, which leaves the data scientists to operate like an artisanal team. This approach has worked well for his company.
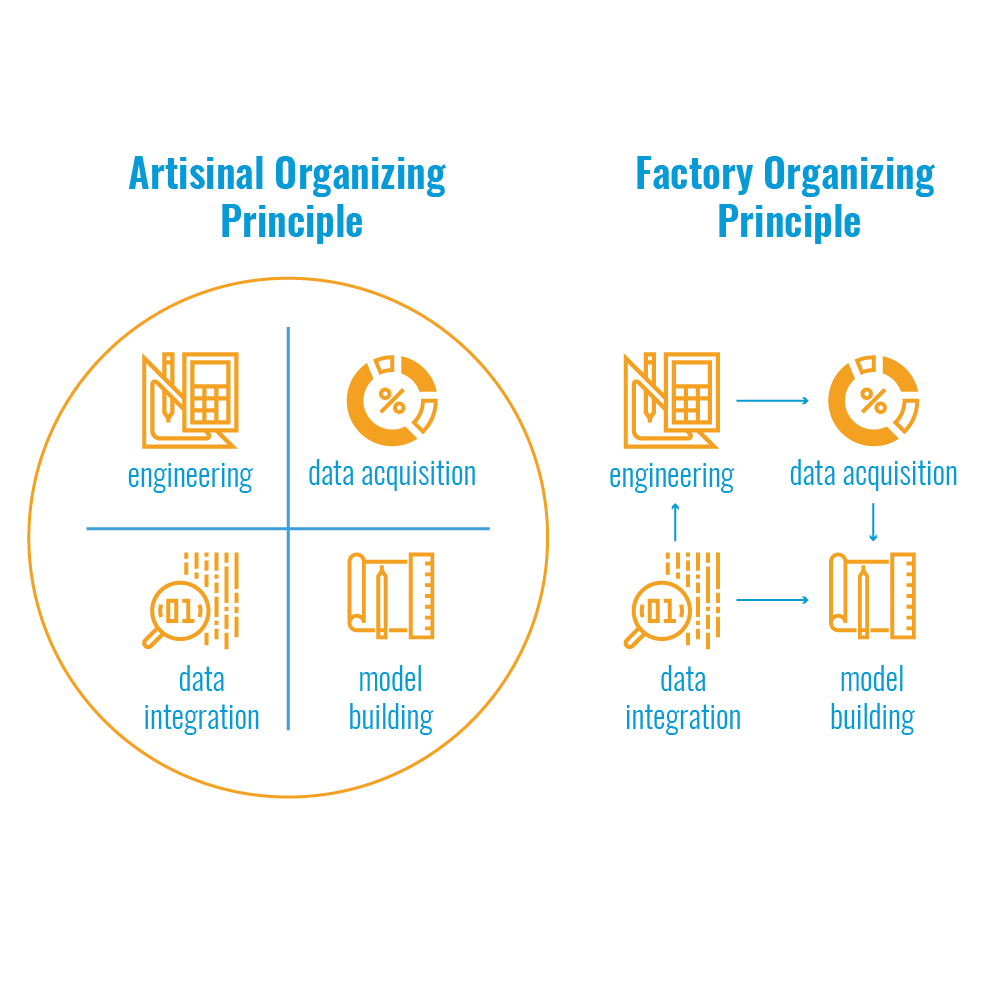
Hybrid Models Are All the Rage
At the macro level, the hub and spoke model or hybrid variants on this theme proved to be the most popular among our podcast guests. Many of the models took on the flavor of the “hub” acting as the central analytics team that sets strategy across the business units and deploys capabilities to come up with solutions. This model strikes a nice balance between governance and nimbleness – and puts your data and analytics team in the position to influence the organization from the top-down, bottom-up, and side-to-side. Also, these hybrid approaches allow you to gain functional expertise while leveraging the central team for bigger, enterprise-wide endeavors. But the most interesting thread in our discussions with CDOs, CAOs, and CDAOs, is the promising trend of situating analytics teams within the context of business objectives. In the past, analytics teams were too often divorced from corporate strategy and reduced to taking orders with little to no business context. Today, the business lines are blurring – in a good way – and CDOs are capturing a common language for their teams and the enterprise. While challenges remain in cultural barriers, budgeting, and scope of work for CAOs, the popularity of enterprise-wide data and analytics strategies has elevated the status of data and analytics leaders and their teams.
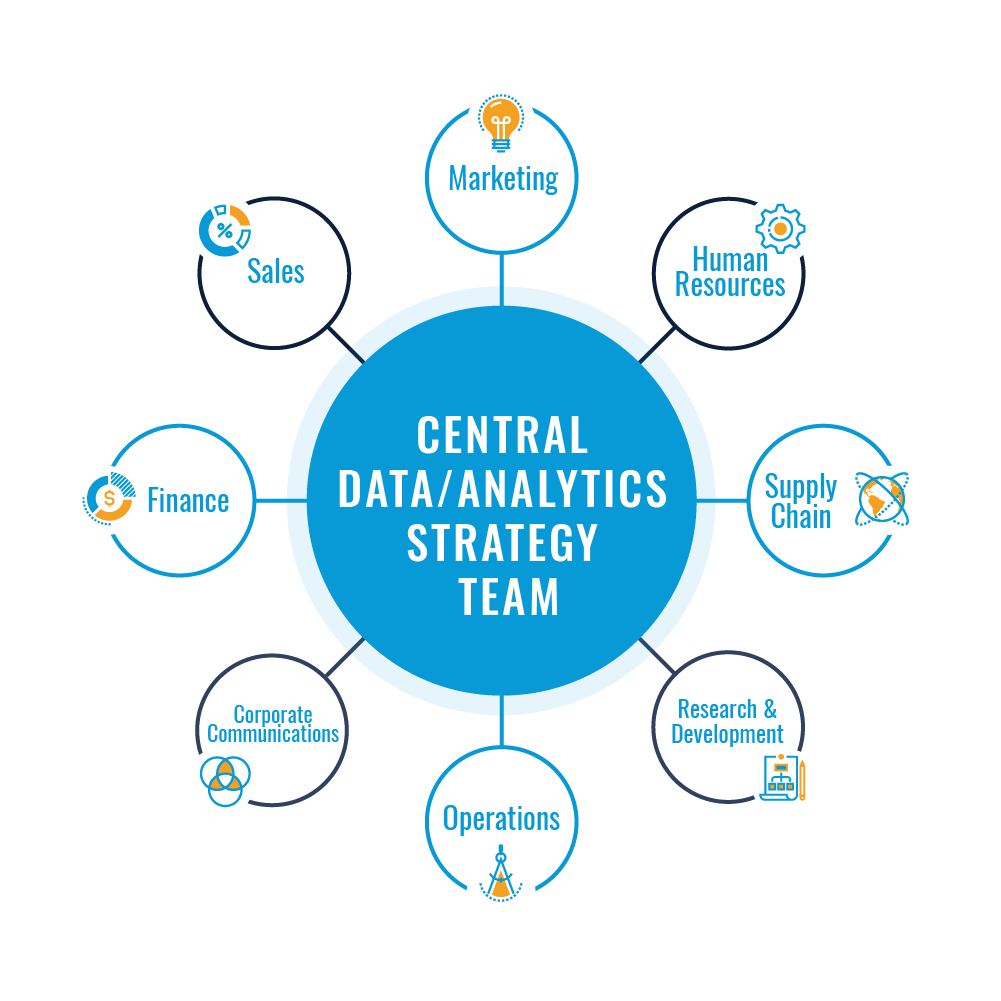
It would be fruitless to declare a supreme organizing model, and that is not the point of this piece. The pros and cons of the “basic six” are well-documented in IIA’s research brief referenced at the top of this article. Instead, our interviews with data and analytics leaders revealed that smart businesses have adopted the Socratic method with analytics. The force of good questions in relation to the culture of the organization and goals of the business and your analytics team have sparked tailored approaches punctuated with the slogan: “It may not work for you, but it works for us.” This means that it makes the most sense to think of operating models on a continuum – from fully embedded analytics anarchy to a fully centralized data dictatorship – and to seek out the sweet spot between these poles.
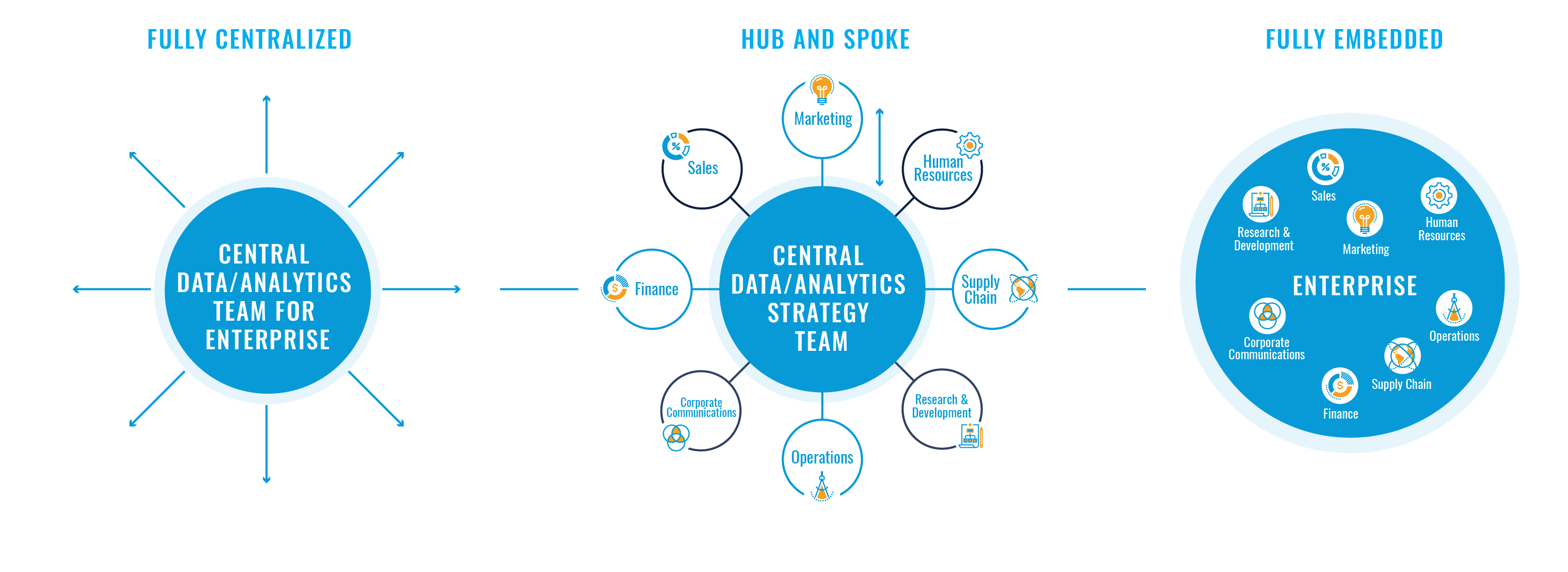
Like the train of a peacock tail, the plumage of analytics organizing models has evolved to court the business. Read below to see what our data and analytics leaders had to say in their own words.
Dreamers, Hustlers, and Hackers
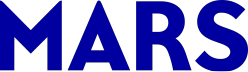
We don’t differentiate too much between technology, analytics, and the business. We believe they need to come together in a seamless way to be able to either help improve the shape, or the bottom line, or to look at the unmet needs of customers. So…it’s about these three things coming together synergistically. And that is also how we’ve designed our teams. Our teams have evolved from functional roles into a more cohesive unit. Heavily borrowing from Scott Cook’s ideology (co-founder of Intuit), we’ve created teams that are a combination of Dreamers, Hustlers, and Hackers. The Dreamers are our business translators and business strategists. The hackers are the data scientists, data engineers, and software developers. And the hustlers are people who manage the day-to-day – from project management to behavior and change management. We’ve recruited and designed our teams in a way to encompass all three. This makes it possible for us to speak the same language and act as the bridge between the business and the technology.
Tarun Kataria, Vice President, Demand Analytics, Mars
The Data Science Pipe
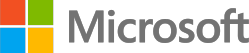
Data science and analytics is throughout the entire company. If you're in finance, if you're in marketing, if you're in sales and engineering…part of your role is to understand and make strong data-driven decisions. But in my role, I partner with Kevin Scott, our CTO, to help organize what we call the data science pipe. And we link into all the data scientists across the company, both from an education perspective, but also from a community perspective. And we leverage each other's talent to try to address both my mission and the commercial missions of Microsoft.
John Kahan, Vice President and Chief Data Analytics Officer Emeritus, Microsoft
A Co-Op Model and Clarity in Charter
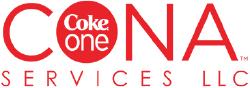
I report to the CEO and we are uniquely structured since we spun off from the Coca-Cola Company…in a sense we have it all but in a co-op model…I’ve seen many different models and I don’t think there’s a one-size-fits-all here. However, where the analytics function is placed is a good indicator of whether management views the role analytics will play as a driver or enabler of business strategy. To me, what is most important is the charter for the analytics team clear? Are they empowered to drive the kind of outcomes that the organization hopes to achieve? And is there a leader with end-to-end accountability to achieve these outcomes? At the end of the day, outcomes are delivered in the field, not in the back office or IT.
Saurabh Parikh, Chief Data and Innovation Officer, CONA Services
Hub and Spoke with Analytics COE
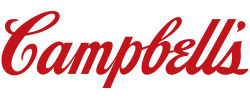
We have a hub and spoke model with COE of analytics within IT. But there are many embedded teams with analytics power and savviness within finance, and sales and marketing, etc. I think claiming world dominance of analytics within a single function is disconnected from reality.
Maria Macuare, Global Chief Data Officer, Mondelez International (former VP of Data and Analytics at Campbell Soup Company)
Decentralized with Analytics Leadership Team
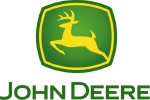
I report through the sales and marketing organization. Analytics leadership across the enterprise at John Deere comes together on the Analytics Leadership Team. We’re a decentralized function within John Deere. There’s 10-12 analytics teams of scale within the organization. The Analytics Leadership Team provides a format for analytics leaders to come together and provide cohesive leadership on foundational items that are common across our teams, like talent, culture, and technology. I love reporting through the sales and marketing function; what I’ve learned over the years is that what works in one company might be a train wreck somewhere else. It’s so highly dependent on the company’s culture and where they’re at in their analytics journey. Also, in my experience, org design is rarely a battle worth fighting. It’s such a political conversation…if you take your org structure as a bit of a given, you can build an operating model that will work with just about any org structure.
Kira Barclay, Director, Analytics, John Deere Financial
In our next Leading Analytics blog, we’ll dive into how CDOs, CAOs, and CDAOs define success for their teams and the top-5 metrics they prioritize to track performance. Until then, happy organizing!
To listen to the complete episodes referenced in this blog post and explore IIA’s Leading Analytics podcast library, check out our website or download on your favorite podcast platform.