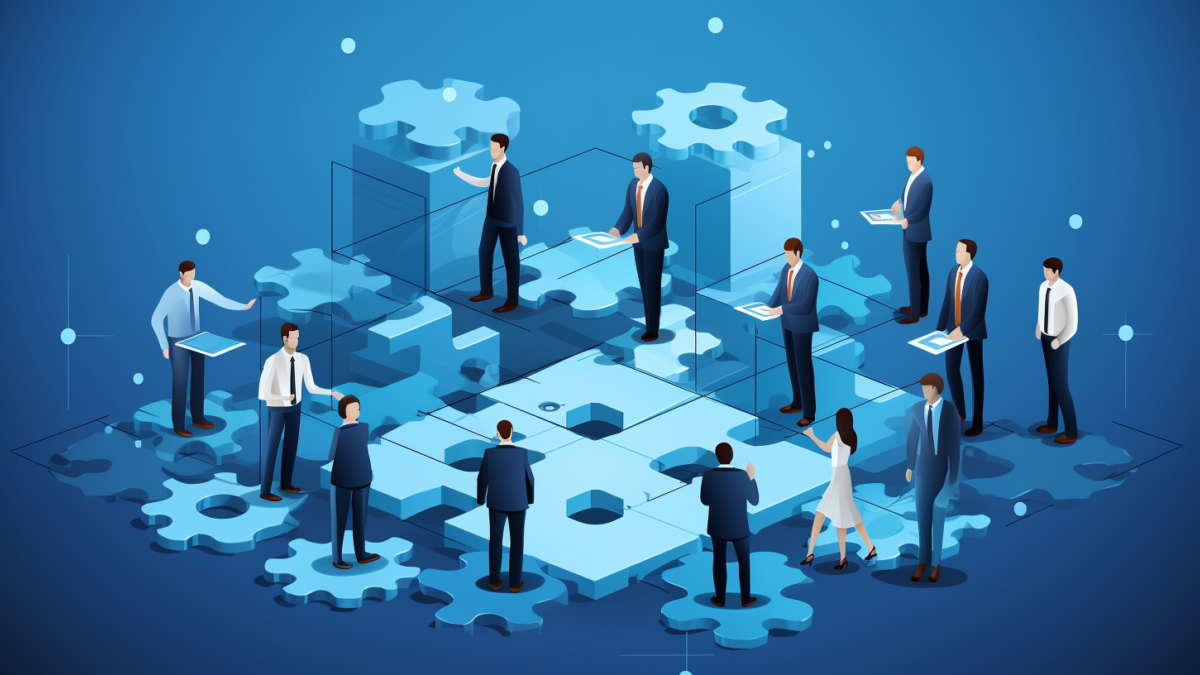
This article is the final installment of our series on organizing analytics. Up to this point, we’ve explored how to select the right organizational model for your business, the common paths of model evolution within a company over time, and ways to coordinate analytics across the enterprise. To round out our series, let’s discuss the various teams and specialties that play into a data and analytics organization as it grows into a mature, business-driven function.
The advice that follows is most appropriate for larger analytics organizations with at least 50-60 members.
Until a certain level of scale is achieved, it is difficult to break the organization into focused teams as opposed to having individuals fulfill a variety of roles as required.
Organizations with a few dozen or less total analytics and data science employees are probably best served to keep everyone in one group with everyone pitching in to help in multiple areas. In an organization that size, there just aren’t enough resources to have people focused on only one area or activity.
As always, different companies will have differing requirements. For example, digital natives will deviate somewhat from traditional companies and normally have a larger percentage of focus placed on data science and data management. Less mature companies often need to emphasize basic business intelligence and getting the core architecture set up before expanding into some of the more advanced areas.
While the organizational components may differ in size over time, each will typically have a manager or director overseeing the team and there may be multiple levels of management as teams get larger. All of them, as will be discussed, should in turn roll up to a single executive, whether that be a CAO, CDO, VP, or CDAO. We’ll next outline each component of the analytics organization and what it is responsible for. Note that this discussion is at the team level and not the individual level. Individual level roles are an entirely different and substantive discussion.
Leadership
An analytics organization needs a leader, ideally a Chief Analytics Officer (CAO), who collaborates closely with a Chief Data Officer (CDO), recognizing their interdependent roles in data utilization and analysis. Sometimes, both roles merge into a Chief Data and Analytics Officer (CDAO), a demanding position due to the distinct and complex skill sets required for managing data and driving analytics. Since analytics focuses on generating revenue and data management on controlling costs and ensuring security, a CDAO might be more adept in one area over the other, often necessitating a senior deputy to balance competencies. The placement of this leadership within the corporate structure is less critical than the acknowledgment of the need for such a role to guide the organization. Further insights into the intricacies of a CDAO's responsibilities are explored in Tom Davenport’s “Succeeding as a Chief Data and Analytics Officer: The Quest for Visible Value” (RAN subscription required).
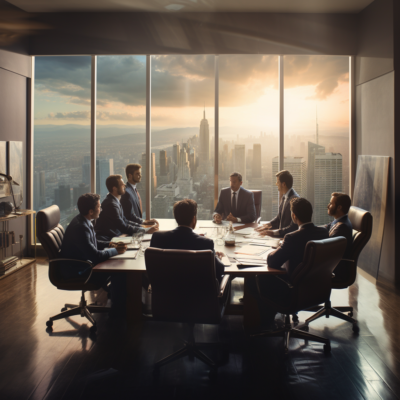
D&A Leadership - Delivering Value Quickly (Webinar)
In this interactive session, you get boots-on-the-ground perspectives from two trusted leaders in the field. Kari Jones and Sandy Steiger will share their practical insights on:
- Successful approaches to mapping out your first 90 to 180 days
- Prioritizing analytics projects to demonstrate the value of your program early on
- Change management processes that emphasize relationship-building and communication skills
The Data Management Team
In an analytics organization, the data management team holds distinct roles from IT's operational data management. Their core responsibilities include overseeing data governance for highly-utilized and produced data, validating data quality for analytics, and managing specific data tools and platforms, such as data lakes, marts, and advanced technologies like GPUs or graph databases. The team comprises IT-savvy professionals like computer scientists and data engineers, focusing on supporting analytical processes, contrasting with the broader remit of IT data engineers. Often, this team constitutes a significant portion of the organization, highlighting the resource-intensive nature of preparing for analytics over execution. In some structures, the data management team might report to a Chief Data Officer (CDO), who can be part of IT, instead of directly to a Chief Analytics Officer (CAO).
The Business Intelligence & Report Automation Team
This is the team that implements and manages basic, ongoing analytical requirements. Tasked with creating, testing, automating, and maintaining the multitude of essential reports and dashboards, they enable data transparency and efficiency across the company, regardless of how sophisticated the organization is. This foundational work prevents analytics teams from being overwhelmed by basic queries, freeing them to pursue higher-value projects. Collaborating with data management and architecture teams for optimal performance, and with business partners to refine and expand reporting, they produce the tools most interacted with by corporate users daily. The trustworthiness and value of this output are crucial, as they set the standard for the organization's confidence in more complex analytics. Moreover, this team is charged with automating newly identified reporting needs and monitoring information usage to continually tailor their services to the evolving needs of the users.
The Data Architecture Team
This is one team that causes conflict related to how to configure it. Typically, IT will own data architecture. However, few stakeholders have a bigger stake in the architecture being done right than the analytics organization. While IT will often officially own data architecture, it is important to have a team working very closely with the IT team to ensure that the architecture meets the needs of the analytics and data science organization. When architecture sits under a CDO, this collaboration might be more natural than when architecture sits under general IT. When architecture sits within the CAO organization, the team is generally very small and is more often responsible for the architectural design and high-level requirements than low-level requirements and execution.
There are plenty of architectural considerations related to the analytical platforms and tools that need to be addressed. While the underlying operational systems are best left to IT, the analytical components need to have a shared responsibility.
The scale, breadth, and efficiency of metric generation, report creation, and deep data science activities must be maximized. The data architecture team within the analytics organization understands both the architectural and the analytical requirements well enough to represent and advocate for the organization’s requirements to the core architecture team.
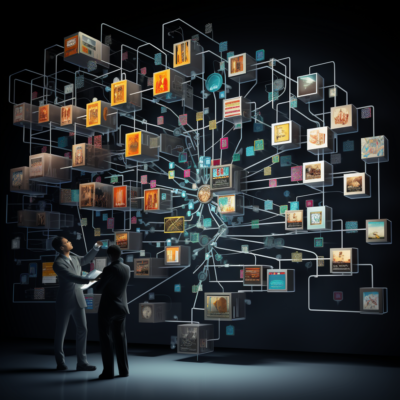
Organizing Analytics and Data Science Organizations Pt. 1 (eBook)
Experts at IIA have put together this eBook as part of a series on organizing analytics orgs. Part I describes and offers guidance on:
- The fundamental goals and objectives of organizational structures
- How analytics organizations commonly evolve and their paths
- Questions and considerations tied to planning your next structural move
The Operational and Ad-Hoc Analytics Team
This team is focused on basic, “light” analytical tasks, supporting various business functions such as HR, marketing, sales, risk, and operations. These members are typically more business-oriented and less technical compared to other analytics teams, often transitioning from a business role with a keen interest in analytics, melding business acumen with substantial technical know-how.
This group addresses immediate "why" questions from business leaders, investigating deviations and problems. Successful one-off analyses may transition into regular reports, handed off to the report automation team for systematic execution. Hence, they act as a conduit for new analytical opportunities, identifying and escalating novel business needs.
Additionally, they play a crucial role in assembling and delivering business review presentations, synthesizing data into actionable insights for executives. They're seen as critical allies for leaders needing assistance to compile and interpret business data.
Moreover, as "analytics translators" mentioned in “Coordinating Across the Enterprise,” they facilitate the flow of complex analytical demands to appropriate analytics divisions, often being the first to integrate within business teams as the analytics function expands.
The Executive Insights Team
As the analytics and data science organization becomes successful and proves itself to the company, senior executives will often get more involved with directly asking questions instead of funneling them through their own teams. While it is terrific to get senior engagement, these senior executives are often quite demanding and want quick response times. This can cause a lot of distraction and conflict of priorities for the other teams defined here.
At some point, it is worth taking some of the best communicators and presenters within the organization and moving them to a small team targeted at directly supporting senior executive requests. Members of the executive insights team serve in a highly consultative capacity and must be versatile enough to be able to handle a wide range of basic requests themselves, while also knowing when to hand off to other parts of the organization when something more complex is required. It is critical that this team achieves a high level of mutual trust and respect with the senior executives they support.
The Data Science Team
The data science team epitomizes advanced analytics and AI sophistication, with expertise in statistical methods, AI, and natural language processing, among others. Data scientists typically specialize in specific domains, ensuring the team collectively addresses the company's diverse data science needs, while individuals may only cover certain facets.
This team's hallmark is innovation, employing a vast array of tools and extensive data sets, often resulting in significant resource use and associated costs. Despite the high visibility and excitement surrounding their work, the data science team forms a small fraction of a mature organization due to the extensive demand for basic reporting, ad-hoc analysis, and data management.
Their work requires interaction across the organization, needing support for architectural modifications and data access, and collaborating with executive insights and report automation teams for deploying their findings.
The allure of data science careers necessitates rigorous candidate vetting to ensure proper skill alignment, especially distinguishing true data science capabilities from those with only business intelligence and reporting experience. The concern is that without genuine coding and algorithmic understanding, reliance on low-code or no-code tools may lead to misleadingly error-free processes that fail to achieve intended outcomes or make unsound statistical decisions.
Specialization Happens as Organizational Size Increases
As analytics and data science organizations expand, individual teams within also grow and become more embedded in their stakeholder groups. This often leads to a hybrid model where team members are distributed across units yet maintain a connection with the central analytics body. Strong ties are essential for these distributed teams to both the analytics core and their business units.
Growth enables specialization; report automation experts may dedicate themselves to specific business units, data management professionals might focus on particular data types or pipelines, and data scientists could specialize in certain algorithms or fields. Such specialization encourages a natural alignment with the parts of the company that require these distinct skills, further promoting hybridization.
However, generalists remain invaluable for understanding a wide array of business needs and integrating the appropriate specialists, akin to general practitioners in medicine who refer patients to specialists. A mature analytics and data science organization will strike a balance between specialists and generalists, and equitable distribution of resources among its teams.
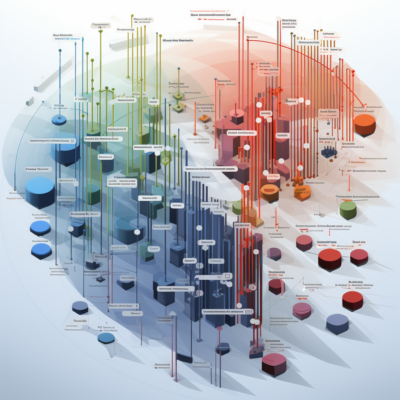
Organizing Analytics and Data Science Organizations Pt. 2
Experts at IIA followed up on the first edition of “Organizing Analytics and Data Science Organizations” this time focusing on developing an enterprise view of priorities and resources in the modern era. The eBook builds upon the foundational knowledge of the fundamental goals and objectives of organizational structure from the first ebook and offers further guidance on:
The evolution of analytics organizations and its many components and variables
Determining your position on the continuum of organizational models
Common paths of analytics organizations and key questions to ask when planning future structural moves
Putting Your Company’s Approach Together
In this series we’ve made the point that the structure for analytics and data science organizations will need to evolve and change over time. Not only will the overall organizational structure morph and flex, but the various organizational components, design variables, and coordination mechanisms discussed will also come into play to greater or lesser degrees as time passes. Even once the overall organizational structure is set, it will be necessary to continue to support that structure from a variety of directions. To summarize the broad areas of organizational support to consider:
- Coordination mechanisms are necessary because whatever organizational model is in place, there will be a need to coordinate across both the various analytics teams and their supported business units. There are several common coordination mechanisms that supplement a formal reporting structure by enabling groups to plan and work together to develop an enterprise view of priorities and resources.
- Design variables must be considered because the data science and analytics function must work in the context of how the business already operates. To evaluate, design, implement, and refine organizational structures, it is necessary to also consider additional variables that must work in harmony for any organizational model to succeed.
- Capability incubation is a critical activity that helps ensure that a company is innovating sufficiently to meet future needs. To support this, it is often necessary for the analytics and data science organization to participate with broader centers of excellence, often owned and led by another unit of the company, by loaning talent for a period of time for a broader corporate benefit.
- Organizational components come into play because as an analytics organization grows, it will be necessary to begin to break the organization into multiple sub-teams with specific charters and specialties. While the components may vary in size as the organization grows, there is no avoiding the need to break resources into specialized teams that coordinate to achieve the required results.
By now it should be obvious that there are no easy answers when it comes to setting, managing, and evolving the structure of an enterprise analytics and data science organization. While there are some consistent themes in terms of both high-level structural models and lower-level execution approaches, every organization will need to find its own path. Regardless of your organization’s starting point, you can be certain that change and adjustment will be a constant. This should be viewed as a purely positive fact because the needed change will be driven by the increasing maturity, span, and capabilities of the organization. While change is only required as progress is made, if the need for change is not accepted, then progress will stall.
Wherever your company is on the maturity curve and whatever size the analytics organization is, the themes and options discussed within this blog series can be of assistance. To the extent that IIA can be of assistance during your journey, do let us know. In the meantime, we wish you success in evolving your own analytics and data science organizational structure in a way that enables not just the team’s success, but the success of your company as a whole.