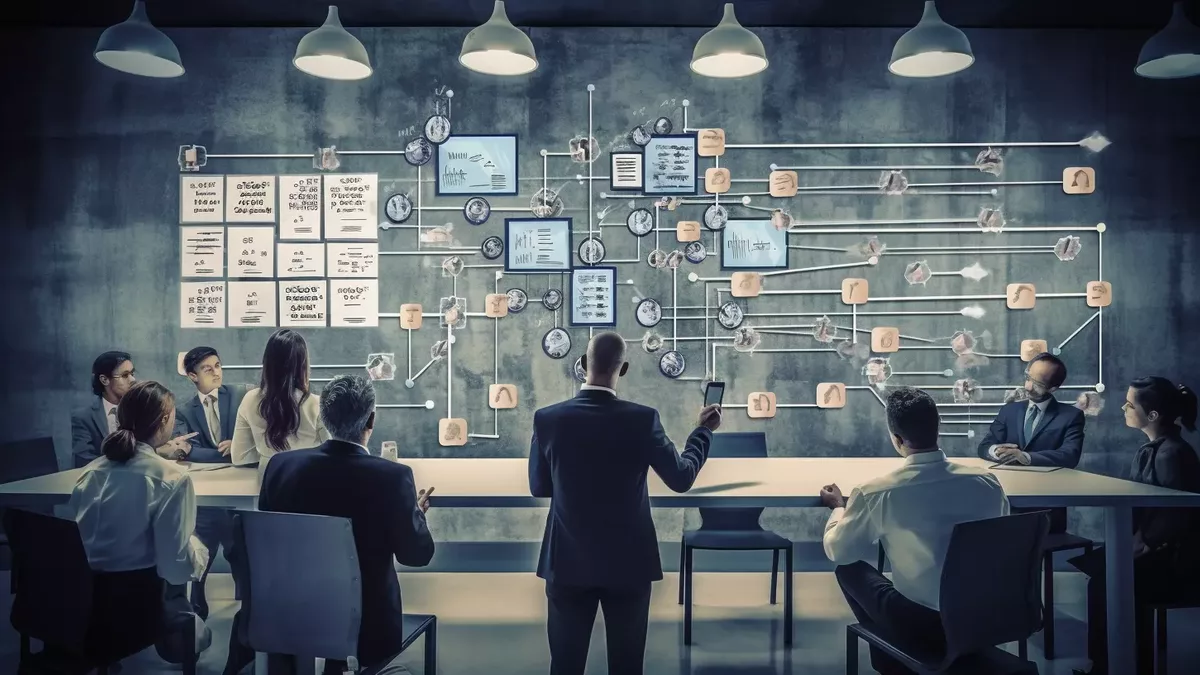
This article is part of a blog series on organizing analytics. Please see the sidebar for the full discussion.
As the first two blogs on selecting the right organizational model and evolving the organization over time made clear, no one organizational model is best in terms of meeting all goals and covering every situation. Whatever the model in place, there will be a need to coordinate across both analytics teams and business units. Even when all analytics and data science resources work in one centralized corporate unit, the customers for their services are spread across the enterprise, and you need coordination mechanisms to manage and meet the internal customers’ demand for analytics.
There are several common coordination mechanisms that can supplement a formal reporting structure by enabling groups to plan and work together, and by developing an enterprise view of priorities and resources. They are ways of fine-tuning your model to help mitigate the weaknesses and buttress the strengths within your organization.
Analytics Governance Council
Governance councils direct enterprise analytics and major project priorities, ensuring progress and fostering unit collaboration. These formal entities, vital in various organizational structures, ideally wield decision-making power to effect change and own data policies. Initially, some may only advise without formal authority. Councils include senior leaders, chief analytics and data officers, CTO/CIO, legal, and corporate strategy and finance members, with potential sub-committees for key projects. Their effectiveness hinges on formal authority over data standards, including defining complex terms like "customer." Without decision-making impact, councils weaken and governance becomes ineffective.
Analytics Translators / Relationship Managers
Experienced staff, known as analytics translators, guide business leaders on leveraging analytics and data science to enhance their units' data consumption. They play a pivotal role in 1) converting business needs for analytics teams, and 2) helping business units apply analytics to seize opportunities. Typically part of sub-teams, they may be data-savvy communicators or analytically adept business professionals. Essential to their role is a product-focused mindset aimed at establishing scalable processes over one-time solutions. Being trusted by both analytics and business personnel is crucial; hence, their selection is strategic. Often, such individuals are sourced from a C-level executive’s chief of staff, acting as the executive’s primary data advisor.
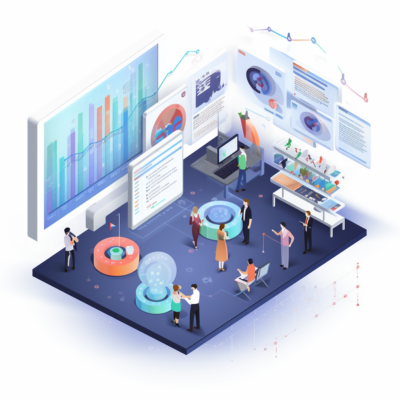
Creating an Analytics Community of Practice eBook
This free IIA eBook provides actionable insights on how to start a CoP within your rorganization, including:
Securing approval and support from business leaders
Suggestions for marketing your CoP internally to maximize interest and momentum
Defining the CoP mission to identify the focus, purpose, value and audience
Analytics Community of Practice (COP)
An analytics community of practice (COP) is an association open to all analytics and data science staff, as well as analytics-minded business and technology colleagues across the enterprise. COPs are good for engagement and retention, as well as knowledge and idea sharing. COPs may host events, internal sharing sessions, and training. They serve as an informal channel for staff development and organizational alignment.
COPs often establish special-interest groups to explore new areas and often lobby management to support items identified as important to the community. Communities can be of particular value in a decentralized model since, given no formal coordination structures, a COP may be the only mechanism available for various teams to interact and align.
Matrix Reporting
In distributed analytics models, teams report to both business units and a central analytics body, with one primary and the other secondary; the structure is influenced by corporate culture. Changing reporting lines can emphasize either coordination or business unit responsiveness. Beware of conflicting priorities and weakening authority in matrix structures. Effective matrix organizations require a formal priority-setting process and performance systems to maintain reporting integrity. Success depends less on matrix specifics and more on stakeholder commitment to collaboration. If issues arise with the current system, it may indicate readiness for organizational redesign in analytics.
Staff Rotation
Staff rotation is a long-term play. Any organizational structure benefits from the movement and cross-pollination of people and expertise. A rotation program can help staff gain experience with multiple areas of the business while bringing each unit different ways of thinking from other parts of the organization. A formal staff rotation program will have defined mechanisms that allow staff to rotate between business units, the central analytics team, and centers of excellence (COEs). This helps staff gain extra skills and exposure while helping to spread common protocols and standards across the organization. Formal rotation programs are very attractive to new hires, especially university hires.
Formal rotation programs for mid- and late-career talent are very hard to manage. For this reason, movement between groups for these more tenured employees is more effective through succession planning between managers and in cooperation with the employee through their career development discussions. For many businesses, this occurs through the annual review process.
Coordination Mechanism Summary
Each of these coordination mechanisms can help fill gaps in the organization and management of analytics and data science teams. Whatever coordination mechanisms you employ, make sure their purposes are clear and their authority levels are sufficient. Expect these mechanisms to also evolve as the organizing model matures and evolves. This is not a static approach that you set up once and leave on autopilot.
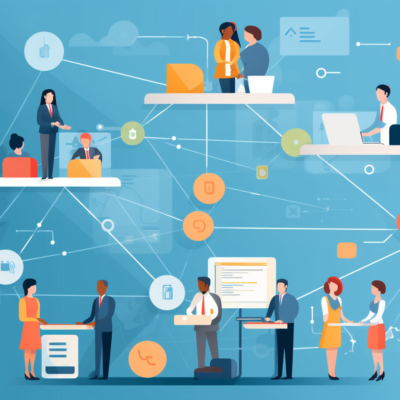
Organizing Analytics and Data Science Orgs Pt. 1 eBook
Experts at IIA have put together this eBook as part of a series on organizing analytics orgs. Part I describes and offers guidance on:
- The fundamental goals and objectives of organizational structures
- How analytics organizations commonly evolve and their paths
- Questions and considerations tied to planning your next structural move
Design Variables
Any organizational design for the data science and analytics function must work in the context of how the business already operates. To evaluate, design, implement, and refine organizational structures, it is necessary to also consider some additional variables that must work in harmony for any organizational model to succeed.
Business Structure
Business structure is about the way the enterprise operates. Are business units highly autonomous or are they closely coordinated? To what extent do business units already share functions, services, and resources? Is power concentrated at the geographical, business unit, or corporate level? Business structure plays a big role in determining the ideal structure of an analytics organization and where it falls on the continuum from centralized to hybrid to decentralized. This is because it is best to implement a structure that matches to corporate precedents and culture.
Reporting Structure
Reporting structure defines authority within an organization, often with matrixed lines to balance stakeholder needs. The analytics and data science unit's central leader—a CAO, CDO, or CDAO—varies in reporting hierarchy. Some report directly to the CEO, emphasizing analytics priority, while others to a C-suite officer like the CIO, COO, CFO, or Chief Strategy Officer, influencing the team's focus and scope. Reporting to a CIO may not be ideal due to potential conflicts between IT cost focus and analytics’ revenue focus, leading to IT prevailing in resource debates. Reporting outside IT can ensure equal footing in executive disagreements.
Physical Location
In the post-COVID era, the significance of physical location varies widely depending on the business, with many companies adopting remote structures. Despite geographical independence, it’s vital for analytics teams to convene regularly to maintain robust relationships and align with business partners. This necessitates intentional planning for team gatherings and team-building activities. Efficiency may still require on-site visits to understand data collection and analytics application in places like manufacturing facilities, underscoring the practical integration of analytics in business operations.
Funding sources
Organizational design must align with funding sources to avoid paralysis. Analytics organizations often have varied funding, including corporate budgets, unit assessments, and project-based chargebacks. A successful strategy involves cost reallocation with the CFO, where operational efficiencies allow funds to be redirected toward hiring skilled data professionals. Digital natives exemplify this, with significant data science teams supported by automated information systems. Effective funding models often reflect company culture rather than rigid rules, combining corporate seed funding and business unit project funding. Stable base funding is critical for team sustainability. As centralized teams expand, they incur greater scrutiny from CFOs, prompting justifications for costs, which can lead to analytics decentralization for clearer cost-benefit alignment.
Infrastructure
Infrastructure encompasses the tools and technology for data handling and analysis, requiring standardized technology and policies to minimize internal barriers. Balancing innovation with maintaining critical processes is essential. IT or operations may oversee production process management, while data science teams often maintain a "sandbox" for experimentation, requiring substantial storage and processing power, especially for model training. Corporate investment in a superior data and technology platform can drive enterprise-wide analytics maturity, necessitating systems integration to facilitate data access across business units. This may lead to a mix of centrally managed and locally managed components within the organizational infrastructure.