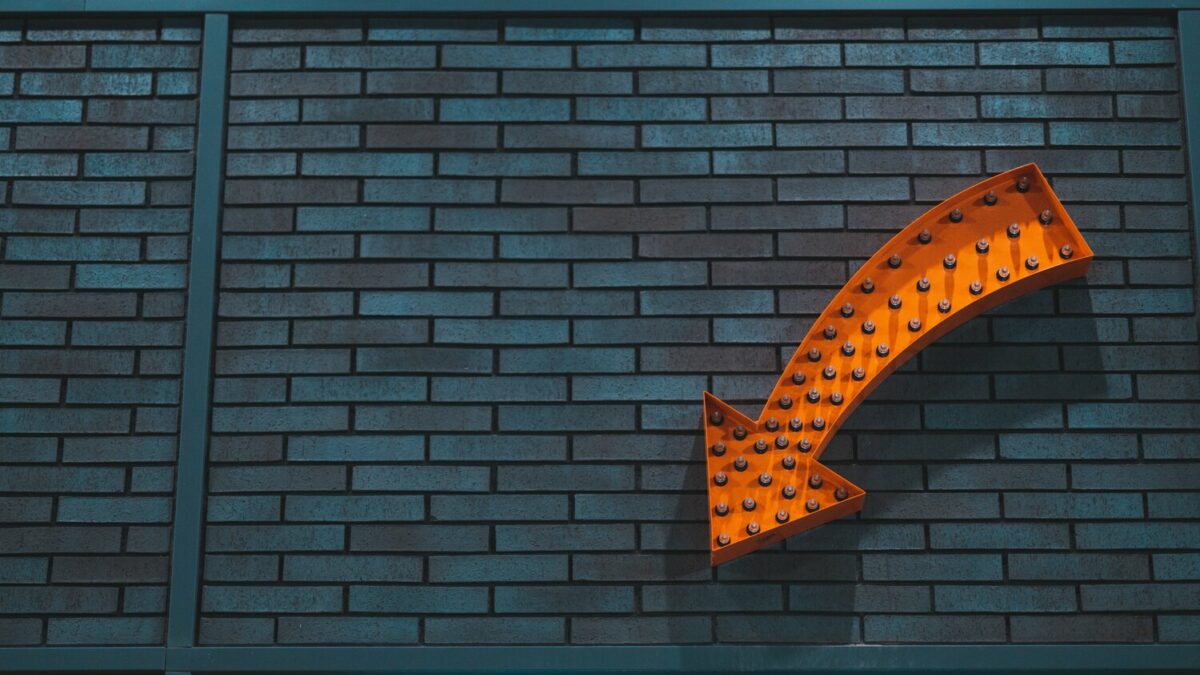
This is the fourth piece in a blog series entitled: “Closing the Growing Gap in Analytics Capability and Effective Use” and this post focuses on how a Centralized analytics organizational model actually increases this gap and reduces the development of effective analytics.
“It Depends” or Does it?
Ask any academic, advisor or consultant, “what analytics organization should I have?” and you will get the standard answer, “it depends.” The list of things on which it depends include:
- Your broader corporate culture, where, for example, if your company operates in a command-and-control mode or if other big functions like Marketing are Centralized then generally it’s presumed that a highly Centralized analytics function is best.
- Your current state of data culture or data literacy, which roughly means – how data smart are the people outside your current analytics functions, aka “the business.” This often assumes, the answer is “not very smart”, in which case a highly Centralized analytics function is proposed to be best.
- The sophistication of your data practices, like data governance, data management, and sometimes the technical aspects like data capture, etc. The assumption here is that all those practices are in development and not yet where they need to be, in which case a highly Centralized analytics function is proposed to be best.
So, in practice it rarely “depends,” in reality it’s often de-facto Centralized. The progression of analytics organizational models towards a higher degree of centralization at large non-digital firms makes sense as firms try to bring order to data chaos and concentrate rare data skills to further themselves along the journey to becoming data driven. We have observed this pattern broadly across industries as analytics has gone from an interesting idea to a business requirement. (For a deeper description of the model types, see “Organizing Analytics” client only research brief, with essential content below for all).
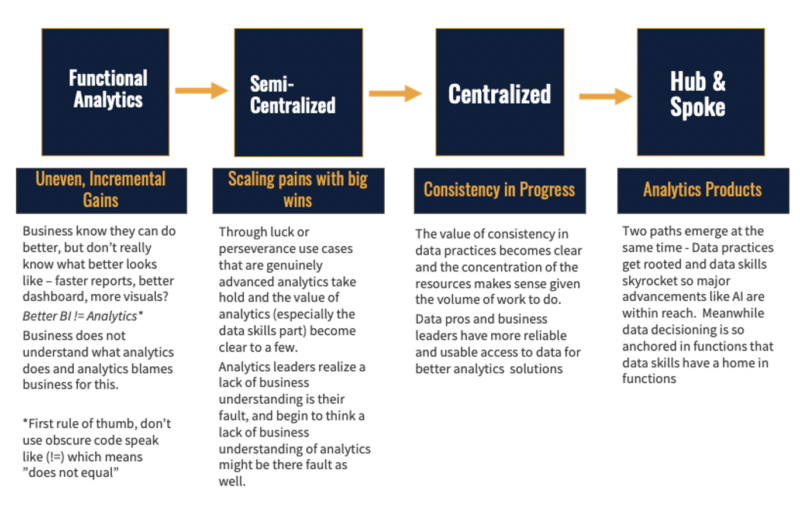
Getting Stuck in Your Analytics Comfort Zone
The problem is that as analytics organizations become Centralized, their size, power, influence, and accountability grows and expands. And reversing the pattern seems to fight against the natural laws of the corporate jungle. It’s rare to meet a corporate leader who says, “I need fewer resources” and there are strong incentives to build large organizations. But, to become as data driven as the much-admired digital natives like Facebook and Amazon, as well as large companies with recent unmatched results, like Microsoft, you must fight the laws of corporate nature. These firms don’t have Centralized analytics organizational models, they have Hub and Spoke models. The analytics function organized in a Hub and Spoke set up will likely be smaller than a Centralized analytics function, since “analytics” activities are pushed out along the spokes closer to where the business decisions are made. Pushing analytics out from the Central analytics function is, by definition, pushing data knowledge, data skills and some data accountability out to the functional areas, increasing data literacy and building a broader data culture. Unfortunately, many with a Centralized function believe that first they build knowledge/literacy/culture out in the functions, and then when that knowledge is deemed acceptable, then and only then will they allow analytics to escape the gravitational pull of the Central function. This concentration of data talent in a Central organization can then move from a blessing to a curse and actually stymie the growth of a data driven culture. This is a key reason that “69% of survey respondents say their organizations are not yet data-driven; an even higher 72% say their firms have yet to develop a data culture.” (2). And the larger the Centralized analytics group, the less likely that group is to be in continuous and deep contact with people driving change on the front lines of the business, and this is seen in several studies that indicate the businesses are not seeing a return on their analytics investment. (3)
Getting Out of The Comfort Zone
While most large firms that are progressing in building analytics capability currently have a Semi-Centralized or Centralized analytics function, most firms who truly leverage data for competitive advantage have a Hub and Spoke organizational model. As much as it’s clear that the most advanced firms use a Hub and Spoke for the obvious reason that action on data drives the value, it’s a bad idea to rapidly switch to a Hub and Spoke model. You do need to build some preconditions to transition into a successful Hub and Spoke. The preconditions can be less well built out than many often think. Luckily, the simple act of thinking “what would we have to do better and differently to be a Hub and Spoke” can help you map and improve those preconditions and develop confidence in making the move.
A good place to start is with the work of the analytics function itself. Since the core role of the analytics Hub is to enable the Spokes to leverage established data practices, componentized analytical models and clear data governance routines, as well support the larger enterprise with finding and exploiting the best of the most advanced analytics tools and techniques, you can start by asking two questions which are polar opposites.
- What processes, methods, platforms, and approaches can we ruthlessly standardize because they support universal needs in uniform ways? Institutionalizing simple and effective methods like the concept of Value Engineering outlined by Bill Schmarzo or establishing business centric analytics prioritization like that designed by IIA Expert Kathleen Maley which she shared on this webinar are two examples.
- What opportunities on the vanguard of analytics are we not applying today, but must do 24 months from now? You can surface the answer to these questions through engaging your analytics team members around tools and techniques that are forever on the “wish list.” Very few of these wishes will deliver business value in the end, but some will make big impacts (think Google’s GPT-3) and in the meantime, the techniques discovered and tools used will be brought forward to improve the standard processes, methods, platforms and approaches mentioned above.
Another approach is to map your analytics value chain activities across two axis – the degree of data vs business skills that are required and the degree of uniqueness vs universality, keeping in mind that a core part of the Hub’s role is to make reusable data and analytics components that enable effective Spokes. With this model it’s critical to push against the tendency that has been developed inside a large Centralized analytics organization to control data and analytics through programs, projects and committees and instead push activities toward the business or the data side, towards the unique or the universal. Avoid the upper left and lower right corner, and especially the middle ground of mediocrity.
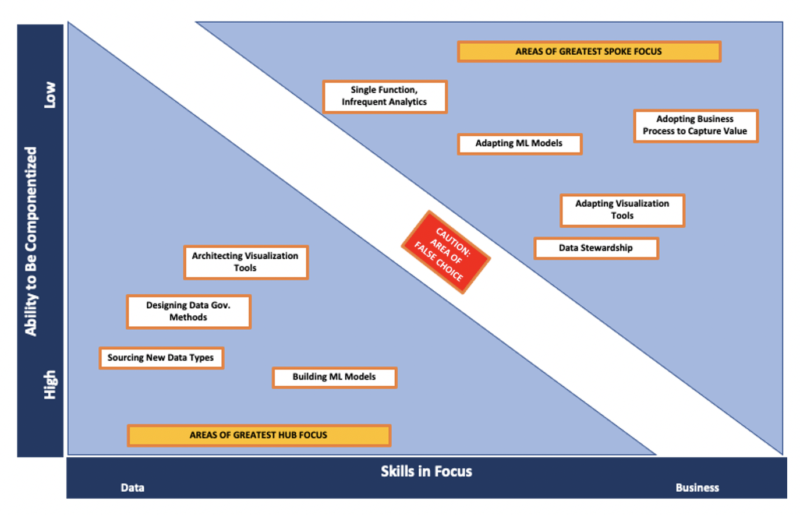
Progress Not Perfection
You will need to move towards a Hub and Spoke model or at least reduce the centralization of analytics and increase analytics in the business lines if you ever hope to “become data driven” or “democratize data.” By definition these ambitions mean that a factorially larger number of people work actively and deeply with data. And to do so you need to find a programmatic way to move the activities out of the Centralized analytics function – that’s for certain. And while these are two approaches, how you do it? Well, that depends.
Appendix
(1)
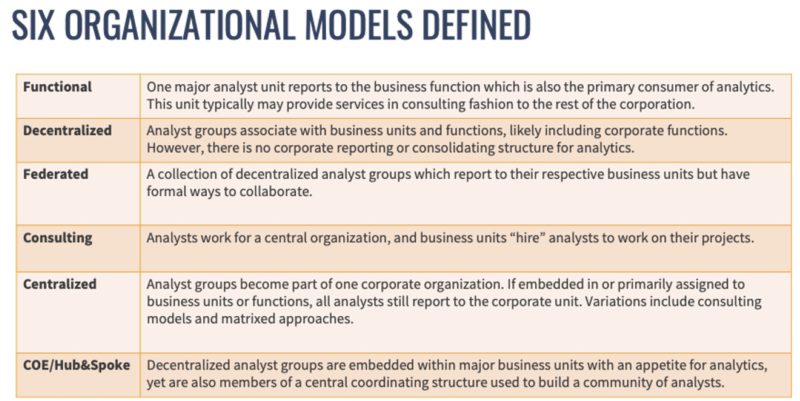
From IIA Research Brief “Organizing Analytics” (client only, contact author for complimentary copy)
(2) https://fivetran.com/blog/how-to-organize-your-analytics-team
(3) https://hbr.org/2021/02/why-is-it-so-hard-to-become-a-data-driven-company
About Drew
Drew has close to 20 years of experience, having worked on both the business side of analytics, leveraging insights for business performance, and on the delivery side of analytics driving the use of enterprise analytics. As the lead of Analytics Leadership Consortium, Drew drives engagement with analytics executives and top analytics practitioners in the IIA Community to help them lead their firm’s journey to analytics excellence.
Before joining the IIA, he led the Enterprise Data Analytics and Governance function at IKEA’s global headquarters in Europe. He leveraged analytics in various leadership roles across the IKEA value chain in both the United States and Europe. He received his MBA from Penn State and his undergraduate degree from Boston University.
About The Analytics Leadership Consortium (ALC)
The Analytics Leadership Consortium (ALC) is a closed network of analytics executives from diverse industries who meet to share and discuss real world best practices, as well as discover and develop analytics innovation, all for the purpose of improving the analytics maturity of their firms and securing the business impact they deliver.
You can view more posts by Drew here.
Follow IIA on LinkedIn, Twitter and Facebook for more updates.