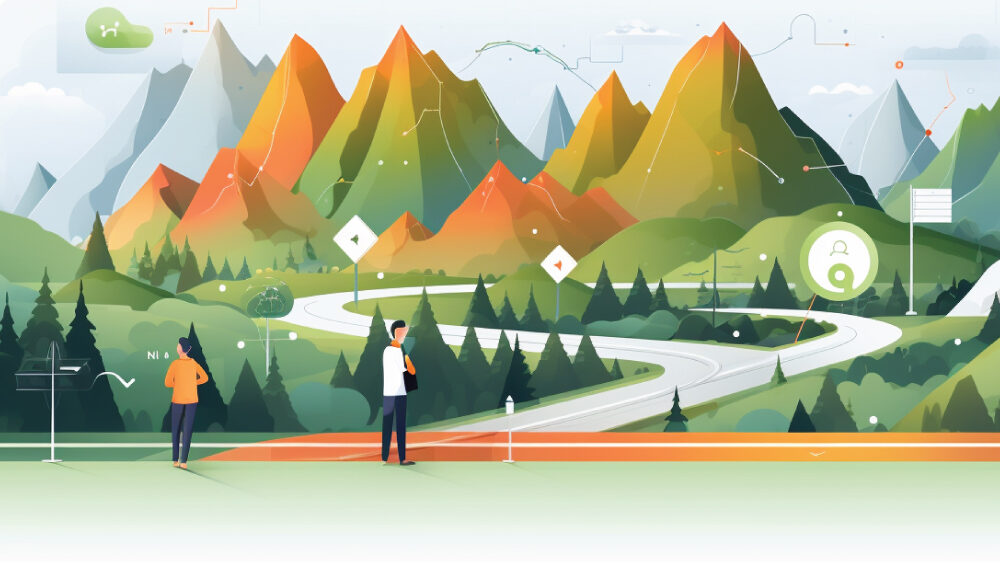
This is part two in a blog series on building a data strategy framework that will empower your organization to transition to advanced analytics and AI. In part one, we explored the essence of a demand-intensive data strategy, and the steps required to set a clear vision that aligns with corporate objectives and assessing your current state (i.e., steps 1-5 in IIA’s data strategy framework). In this article, we’ll discuss how to take the vision you’ve set, the principles you’ve defined, the assessment you’ve undertaken, and then build and execute on a plan.
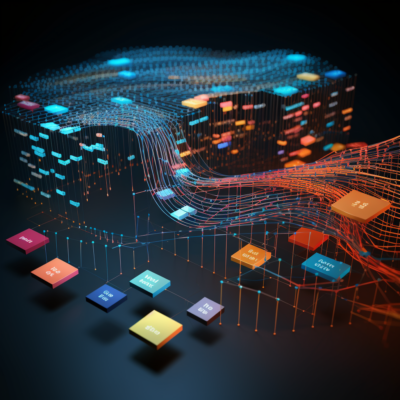
Creating A Data Strategy eBook
The growth in the demand for better decision-making with data means that a comprehensive data strategy is no longer a nice to have. You need a data strategy.
Download this eBook now to get actionable recommendations, on topics including context and vision for data strategy, creating proper objectives, developing a framework to evaluate where you are now and qualitatively assess your end state, and tips to plan your architectural moves and modify business benefit-delivering use cases.
Step 6: Architecture and Theory of Operations
To advance toward the desired end state, focus on the following two workstreams in parallel: architecture and use cases. First, we'll discuss architecture. Architecture is usually about defining a platform, and a set of common services, along with a set of statements — a theory of operations—about how you're going to operate and manage that platform. You need to define both, keeping in mind that there will be a (partially-complete, partially-off-center) version of your end-state architecture, for each major step in your organization's transition from as-is to to-be states. Similarly, your theory of operations will change, as the platform and its assumed level of service matures over time. The essential point, we believe, is this: an architecture is incomplete without its accompanying theory of operations: how the platform(s) works, and who does what, when, how and to whom.
Answer questions such as: How will the data enter? How will metadata get captured? How does data get transformed? How generally is security implemented? What do you and don't you facilitate with common services? How do you support people who want to perform tasks not supported by the platform explicitly?
When it comes to platform technology, you need to think about aspects such as which technology to use for the data integration and engineering layers of the platform, and how you're going to use and integrate the various technologies that control different aspects of the ecosystem. You've already established principles around many aspects of architecture. Now you are specifying details that will help you implement the changes. Ensure that you cover areas such as data acquisition and ingestion, data engineering, resilience and recovery, and governance, to name a few.
Step 7: Use Cases
Gather a broad spectrum of use cases for data by conducting interviews with business stakeholders. Focus on the following areas:
- Renovation: Use cases for existing data producers, systems of record and data consumers that need to be updated.
- Underserved and unserved constituencies: Use cases for existing functional areas that need additional analytics support, or any support at all.
- Speculative: Use cases where no demand exists but that you predict will be required in the future.
Step 8: Road Map
With the architecture, theory of operations and use cases defined, you are ready to generate a road map. The road map is a sequence of platform enhancements, many in service of a particular use case, implemented over time, such that the collection of enhancements and extensions get you from where you are now to where you want to be, within the planning horizon. Some components you must build upfront, such as security and metadata management. However, the trick to good roadmapping is: using business benefit-delivering use cases to drive platform build-out, so that you can show the business value for each new functional enhancement, and allow future use cases to treat that new functionality as part of the "assumed level of service" provided by the platform.
For example, you may decide to solve a booking-to-cash use case early on, because you have many issues with outstanding receivables and getting payment. Solving for this problem may involve solving for getting the required data (from SaaS and on-premises systems) into the data lake, normalizing the data, re-engineering that data so you can persist it in a relational database, and building an analytical application that allows you to model forward collections and cash from the data sets. In solving this use case, you're actually creating a general purpose mechanism for ingesting data from in-house systems and external cloud systems. You're also building a data pipelining mechanism with transformational elements that can be reused, and a use-case specific analytical application.
Step 9: Execution Plan
Data strategies don't return value unless you execute them well. The execution plan answers the question, “What do we have to do to get this going?” There will be thousands of tasks that need to be performed by various teams in a particular order. In fact, there are too many to elaborate on in their entirety here as the tasks become specific to an individual entity.
However, we will highlight that in thinking through your execution plan be sure to consider effective communication throughout. Execution is as much about winning the hearts-and-minds as it is tactically moving the target from one spot to the next.
The execution plan is not a static document. As you work through use cases, issues will arise. You may not initially choose the right technology, or you may encounter dirtier-than-expected data. You will need to regroup to monitor and measure the implementation of the strategy, and update the strategy as needed.
There are also organizational implications along the way, from platform ownership to talent acquisition to alignment with your analytics strategy that may touch on expanded organizational structures as well.
Step 10: Attributes for Success
One of the main predictors for success is the ability to answer the question, “Why are you creating a data strategy?” with something other than, “Because I was asked to produce one.” You must know why improving your information economy is necessary, and what the economic payoff will be. This goes back to step one—i.e., linking your data strategy to corporate strategy and prioritizing your data consumers.
In addition, data strategies have a higher probability of success with the following environmental factors in place:
- Senior leadership agrees that what you have done in the past to make data available for analytical use has not worked, and agrees to provide the leeway, space, budget and resources for you to perform the necessary long-term work.
- A data management organization exists, whether it's led by a chief data officer (CDO) or not, and this organization agrees with the leadership's perception about the current state of data and is committed to thinking about data in new ways.
- That organization recognizes the existing issues with availability, quality and veracity, and recognizes that mental models from the past produced the problems that led to the current data problems. In addition, the organization is confident they can provide data fit for the demand side's intended use.
- The organization has well-informed demand-side constituents who can describe what they need and what they intend to do with the data when they have access to it. Further, these demand-side constituents agree to be vocal when successes occur, and not only when failures or setbacks arise.
- The executive and data management teams understand the demand side of the information economy in the same way, ideally through exposure to a broad spectrum of demand-side use cases.
- The organizers of the data strategy initiative recognize, and have planned for, the substantial change management effort that is required. Far more than any technological changes that need to happen, the initiative is reliant upon colleagues' embracing the necessary mindset shift.
- The organization recognizes that a data management strategy is a dynamic, living document and will need to be adapted as the internal and external data landscape shifts. The worst data strategies are those that fail to start with the demand-side of the information economy front-and-center. Those strategies lead to time, money and effort spent provisioning data, followed by the discovery that demand-side users can't leverage even a small portion of what's been made available. We've been-there-done-that; we need, these days, to do better.