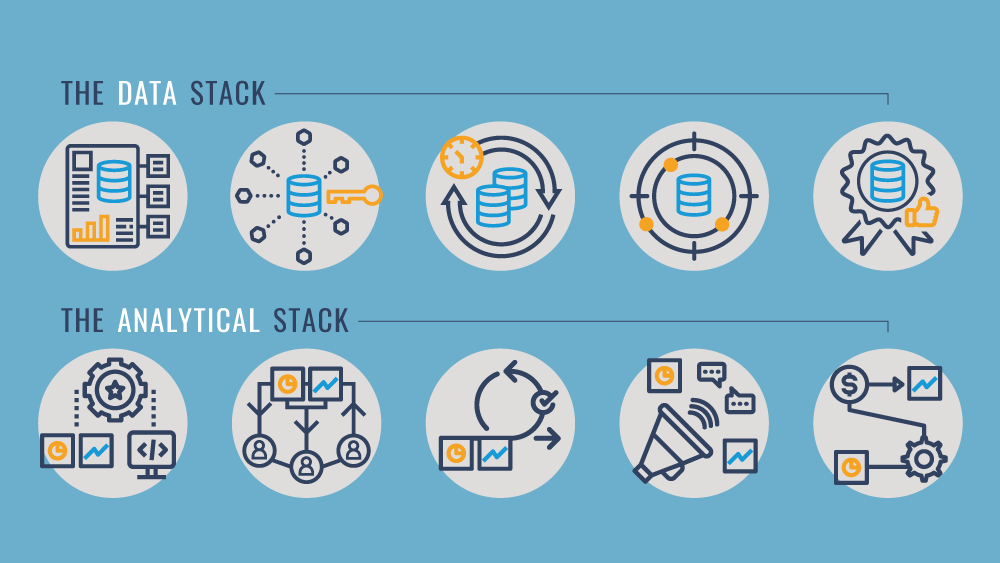
In the first installment of our BI maturity blog series, we explored why BI maturity matters and the achievements of BI-mature companies. We showed that BI insufficiency plays a key role in difficult transitions to advanced analytics and AI at the enterprise-level. In response to client challenges around BI—and getting stuck in stage 2 of the DELTA Plus model—IIA created the Business Intelligence Maturity Framework.
Based on our findings, the IIA Business Intelligence Maturity Framework divides core competencies into two stacks: a data stack and an analytical stack. The stack metaphor is deliberate, as we believe the competencies (read sufficiency) at the top of each stack are “soft”—social and organizational, and more difficult to attain—while the domains at the bottom of each stack are “hard” (i.e., more technical in nature). When applying the framework to assess the state of BI within your organization, recognize that within each layer of each stack, there exists a capability maturity curve, indicating a general progression from “no material capability” to “nearly complete.” The objective is not, of course, to achieve “nearly complete” in each layer of both stacks. This model helps answer important questions about having enough tools for smart data use, and our research shows that the crucial point on these progress lines is when you have just enough capability across the data and analytical stacks to not slow down advanced analytics projects.
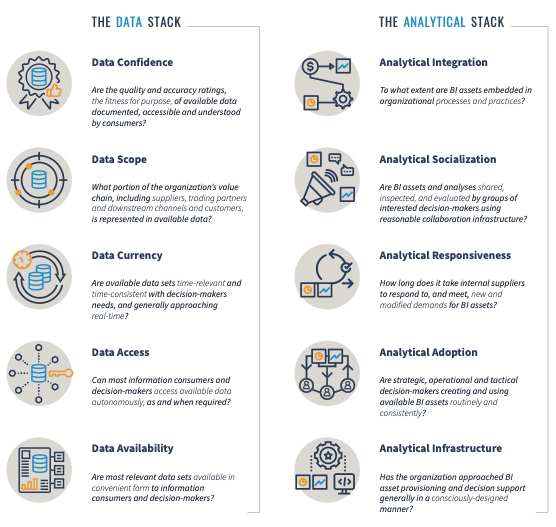
The Data Stack
1. Data Availability: Data availability is the bedrock of the data stack and the single most important aspect of BI sufficiency. Organizations that attempt the transition to advanced analytics before achieving sufficiency in data availability simply carry the data availability problem with them into a new, expansive domain of analytics that places additional, and more varied, requirements on available data than did descriptive and diagnostic BI. On the other hand, organizations that have successfully addressed the data availability challenge for a significant part of their essential data assets discover that advanced analytics projects lead to the development of additional “extraction” and “transformation” pipelines. These are similar to what the organization already understands, has documented, and has put into action, but on a larger, quicker, and more computationally demanding scale.
2. Data Access: Data access, a critical aspect of BI sufficiency, involves the capacity of BI users to acquire and utilize data through standard, predictable methods. Highly efficient BI environments, providing reliable end-to-end data provisioning for human use, stand as a fundamental test for the broader challenges in transitioning to advanced analytics. This shift encompasses not just human users but also programs—computational logic projecting, assessing outcomes, and making decisions. For instance, a “customer churn” concern may initiate as a BI dashboard displaying customer purchases, progressing into predictive analytics identifying churn propensity. Ultimately, it integrates into the sales or channel automation system, directing specific actions for managers based on validated propensity scores. Each advancement adds complexity to questions of data access and integration, underscoring the need for consistent, dependable access to available data for both human and automated decision-makers. This seamless access proves pivotal in the journey toward advanced analytics, ensuring uniformity and reliability in data utilization.
3. Data Currency: IIA frequently guides clients who have mastered data availability and access but are working with datasets that are 30 to 90 days outdated. As advanced analytics and AI emphasizes granularity, demanding transaction-level details, it concurrently urges a shift to real-time data provisioning for immediacy and currency. Organizations consistently delivering transaction-level data in near real-time across their data portfolio to human decision-makers with self-service business intelligence tools are nearing the threshold of achieving BI sufficiency. In contrast, organizations supplying aggregated data 30 to 90 days post-events are ill-prepared to meet the rigorous data currency requirements of advanced analytics projects. This stark contrast in data provisioning timelines underscores the critical role of timely and detailed data access in the successful implementation of advanced analytics and AI initiatives.
4. Data Scope: IIA often finds that its early-stage clients have difficulty expressing the extent to which their available decision-making data products cover:
- Their internal transactional source systems portfolio (which is in many cases itself undocumented or incompletely documented).
- Their value chain, including externally sourced data on or from suppliers, trading partners, and downstream channels, markets, and customers.
The critical factor for achieving BI sufficiency in advanced analytics success lies in the extent to which decisional datasets, finely detailed and nearly real-time, span [a] the organization's internal operations and [b] the external value chain. The scope of available data is the primary impediment to successful data science experimentation, the pivotal first step in creating production-ready advanced analytical applications. In our experience, firms transitioning to advanced analytics often cover less than 50 percent of internal transactional sources and less than 25 percent of the external value chain in their data scope. This insufficient coverage hampers data scientists and business analysts, limiting their exploration and experimentation needed for high-value economic pressure points in early advanced analytics projects. Many of these crucial points exist at the boundary between the organization and its suppliers, trading partners, or downstream channels and customers—areas where the data scopes of numerous clients fall short.
5. Data Confidence: Data quality and accuracy, familiar metrics for data governance and BI professionals, serve as proxies for the pivotal metric determining the success of BI environments: data confidence. This confidence reflects the willingness of data consumers to trust and act on the provided datasets. Easily compromised, it may require only two analysts with conflicting answers to undermine an entire team's confidence in their decisional data sets. Establishing and sustaining this confidence depends on transparency about data trustworthiness dimensions, including sources, accuracy, completeness, and fitness-for-purpose. Over an organization's BI journey, this confidence becomes a crucial foundation for demand-side constituencies in advanced analytics, fostering trust in models and their inferences. The broad deployment of advanced analytics and AI applications relies on perceiving supply-side decision support professionals as reliable, value-adding partners. Organizations using transparent, metadata-based methods to communicate data set characteristics build the necessary confidence for successful in-production advanced analytical applications. Conversely, those lacking such cataloging and advertising struggle to meet the needs of data science teams and fail to establish the crucial rapport between supply-side and demand-side in an advanced analytics-focused environment.
The Analytical Stack
1. Analytical Infrastructure: Many clients still deploy business intelligence assets in isolated stovepipes, lacking an integrated platform for data and information delivery. These disconnected systems hinder the success of advanced analytics projects, demanding a common platform for efficient data productization and information delivery. Whether it's a data warehouse, data lake, data lakehouse, datahub lakehouse, or data mesh, the critical factor is a level of data productization that emphasizes automation, reliable production, and full instrumentation of the information value chain. The common platform must not only exist conceptually but be a widely used environment for decision-makers and analysts. Successful organizations, progressing beyond stovepiped BI, demonstrate substantial advancements in their common platform and a thriving community of use. They find it easier to meet the incremental demands of advanced analytics projects, supporting complex data engineering and deploying sophisticated applications. In contrast, organizations relying on isolated BI delivery lack a foundation for advanced analytics, making it challenging to justify substantial investments in an advanced analytics platform.
2. Analytical Adoption: Analytical adoption is a critical aspect of BI sufficiency often overlooked in analytics strategy. Extensive integration of descriptive and diagnostic BI at all decision-making levels enhances organizational imagination and familiarizes decision-makers with BI's power and limitations. Neglecting to measure real usage and achieve a balanced mix of strategic, operational, and tactical adoption leads to imbalanced BI deployment. The imbalance is often linked to organizational power or budget distribution. Top-down management produces top-light, KPI-focused dashboards, while distributed funding leads to a mixed portfolio, and an edge-first approach results in tactical BI applications. In each case, at least one decision-making tier is underserved and unprepared for advanced analytical applications aiming to engage in decision-making processes. Organizations realizing this imbalance during advanced analytics discussions face resistance from constituencies unfamiliar with deficiencies in their current analytics setup. In contrast, companies focusing on widespread adoption of descriptive and diagnostic BI, measuring adoption in all tiers against targets, swiftly transition demand-side constituencies to needing more guidance for better decisions. This transition is crucial for the successful introduction and acceptance of advanced analytical applications.
3. Analytical Responsiveness: While naturally occurring federated analytics models are emerging, current conditions in the advanced analytics and AI market dictate that production-grade solutions are primarily the domain of well-funded centralized analytics teams. Despite the rise of low-code/no-code AI development environments, the intricacies of model governance and reliable deployment remain beyond the reach of distributed analytics teams. For the foreseeable future, central analytics teams will be the primary source of advanced analytics applications. The main challenge lies in the critique of centralized teams for their perceived lack of responsiveness to shorter-cycle business demands, as evidenced by project backlogs. Organizations with shallower, recent-origin BI backlogs generally fare better in advanced analytics delivery, reflecting a commitment to agile methods. This commitment not only reduces backlogs but also prepares teams and beneficiaries for the agile nature of advanced analytics. Successful advanced analytics projects often correlate with a prior competency in prioritizing backlogged BI projects collaboratively and transparently with business beneficiaries. Transparent, beneficiary-centered reprioritization processes are crucial for effectively taking the right advanced analytical applications into production while maintaining confidence in the analytics team—a critical aspect for a smooth transition to advanced analytics and AI.
4. Analytical Socialization: In the realm of business intelligence, the primary product is often a BI asset—such as a report or dashboard—consumed by human decision-makers. More mature organizations explicitly manage these assets, controlling versions, examining derivatives, and tuning performance. They invest time in understanding how assets are shared and introduced into decision-making processes. These practices are highly beneficial in the advanced analytics context: skills in asset inspection and management directly translate into model governance and inspection. Collaborative infrastructure and insights into how BI assets are leveraged become essential for deploying advanced analytics applications, ensuring tight integration with work practices. Organizations treating BI projects as complete upon asset production, rather than its usage, often learn about the social dimension of analytics after failed advanced analytics projects, leading to confidence and reputation loss. Successful advanced analytics strategies acknowledge the complex social context, employing processes and skills to address social factors and enhance the chances of successful project implementation.
5. Analytical Integration: From IIA’s viewpoint, the crucial difference between the BI and advanced analytics and AI realms is business process integration. Many BI programs lack a focus on business process integration; their projects are deemed complete upon asset delivery, raising questions about actual usage and how assets support business processes. Success measurement in these programs is often based on asset delivery rather than utilization or returned business value. Advanced analytics programs are fundamentally about returned business value, requiring high utilization and effective integration with business processes. Measuring success solely based on model delivery or utilization is ineffective and risky for analytics teams. Organizations with success metrics based on integration with decision-making processes and returned business value are more successful at gaining buy-in, avoiding technology pitfalls, integrating models into business processes effectively, and justifying projects based on added business value, be it cost reduction, revenue enhancement, or risk mitigation.
How Much BI Is Enough?
Reviewing any ongoing descriptive and diagnostic BI program through the lens of this sufficiency model is consistently a sobering experience when approached with a cold, clinical orientation. In discussions with IIA clients about BI sufficiency, two common questions arise:
- Can't we bypass further BI expansion and directly shift to a focus on advanced analytics?
- How much BI capability is adequate for a successful transition to advanced analytics?
The response to the first question is affirmative, but it comes with a caveat—deferred work on data, platform, and user education and adoption must be carried into the new strategic frame centered on advanced analytics. Accelerating the transition to advanced analytics without addressing the backlog can lead to increased costs, decreased responsiveness, and heightened dissatisfaction among business beneficiaries. Another approach involves aggressively achieving sufficiency in the data stack, deploying self-service capabilities in the analytical stack, and reallocating resources from lagging BI projects to leading advanced analytics projects. For the second question, see IIA’s Business Intelligence Maturity Assessment (BIMA), which measures 50 core BI competencies, ranging from data scope and confidence to analytical integration and socialization. This quantitative assessment gives an enterprise – especially those in stage 1 or stage 2 of the DELTA model – an understanding of their BI capabilities and their readiness for advanced analytics and AI.
Here are key themes that we see coming out of IIA’s BIMA:
1. Naturally occurring demand for advanced analytics is a strong signal of BI sufficiency, especially when constituencies express this demand without prompting. If such demand is regular, coming from users familiar with BI infrastructure limitations, sufficiency has likely been achieved; if exceptional and limited to one or two constituencies, sufficiency is approaching.
2. Organizations approaching BI sufficiency have largely automated data provisioning, evident in the broad and heavy usage of BI data sets. Backlogs are defined by requests for additional asset development rather than new data sets, with a focus on computationally intensive analytical needs, especially in pricing, transportation, logistics, and customer behavior.
3. Successful advanced analytics endeavors often follow the widespread deployment and use of self-service BI capabilities across organizations. These capabilities raise the competencies of citizen analysts, pushing them beyond the limits of descriptive business intelligence to formulate requirements for advanced analytics.
4. Organizations quickly achieve BI sufficiency when data-driven decision-making is embedded in corporate values, formulations, and procedures. Full-throated adoption by senior leadership, coupled with top-down demand, propels the organization to recognize advanced analytics as essential, with leaders modeling expected behaviors and funding projects for platform development.
5. BI sufficiency is demonstrated by clear linkages between BI assets and measurable returned business value, ideally articulated by BI consumers themselves.
6. An organization witnessing significant numbers of vocal, competent distributed teams of citizen analysts is not dealing with rogue IT projects but experiencing an early expression of naturally occurring demand for advanced analytics, especially when governed well within a federated model from a central center of excellence.
Ultimately, organizations that are not consciously working on defining and achieving BI sufficiency will arrive at a sufficiency threshold in time. Unfortunately for most of those organizations, that time will be well after they have declared a strategic transition to advanced analytics. In many cases, it may be too late for measurable competitive advantage, either from traditional business intelligence or from advanced analytics.