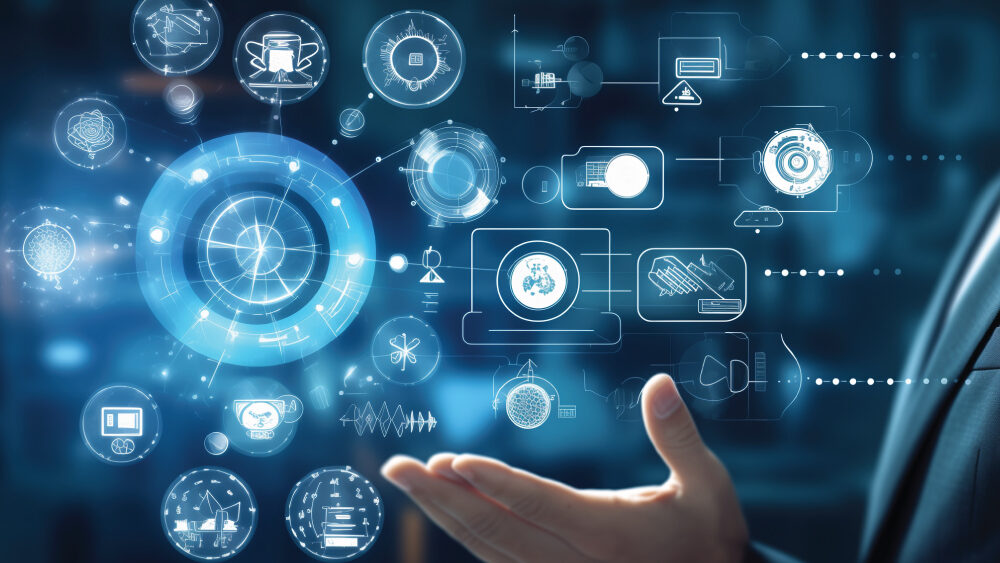
The BI Sufficiency Problem
For over a decade, IIA has assessed and evaluated the analytics maturity of hundreds of companies, guiding these enterprises in their transition to advanced analytics and AI.
Recently, we closely examined a group of clients scoring below the midpoint of 3.0 on IIA's Analytics Maturity Assessment (AMA). Focusing on companies with minimal year-over-year improvement, we identified a set of obstacles collectively termed Business Intelligence (BI) sufficiency.
In practice, companies that have not achieved a level of sufficiency in descriptive and diagnostic BI prior to their strategic turn to advanced analytics and AI face, uniformly, a struggle to develop their advanced analytics and AI competencies. This insight is especially relevant in today’s data and analytics climate, where the buzz around large language models and generative AI has seized the attention of CEOs and boards. Investments in AI technologies are rapidly increasing, placing significant pressure on enterprise data and analytics organizations to implement AI for scaling and optimizing business operations.
To be clear, we do not doubt the potential power of AI for business operations. We’re in a very exciting time for this technology. Yet, this buzz isn’t new, but rather renewed because of ChatGPT’s impressive natural language processing skills and user-friendly interface. We’ve seen too many companies enjoy local analytics success at best with tremendous time, energy, and investment. Nearing ChatGPT’s first birthday, what concerns us most is the temptation to “adopt” AI without reaching the necessary level of BI maturity. AI vendors tout their solution as an accelerant, which may be true in the right conditions. But if your BI house isn’t in order, the result may be an acceleration of failure rates in your data and analytics initiatives.
The BI sufficiency threshold is easy enough to understand. Reaching a sufficiency in descriptive and diagnostic business intelligence has a number of effects on organizational processes, competencies, and perceptions; effects that prepare the organization for the substantially more complex world of advanced analytics and AI.
The Achievements of BI Mature Companies
Companies that have achieved sufficiency in business intelligence share the following achievements:
- Made significant progress in producing fit-for-purpose, current, actionable data sets for BI user communities, covering much of their internal value chain (functions and business units), as well as their external value chain (suppliers, business partners, and downstream channels and customers).
- Developed an architected, platform-oriented approach – whether based on a data warehouse, data mart, data vaulting, or data lakes – to deliver decisional data sets to decision-makers. They are often armed with highly intuitive self-service business intelligence tools designed to increase those decision-makers’ levels of autonomy in creating, evaluating, and sharing business intelligence assets, including dashboards, reports, and analyses.
- Built or purchased collaborative technologies that underpin the essentially group-oriented nature of commercial decision-making, so that analysts of all sorts can share their analyses, benefit from the insights of others, and circulate and raise the level of best practice within the organization.
- Consciously developed strategies and programs to drive BI usage across the strategic, operational, and tactical decision-making tiers in their organization, measuring not “seats licensed,” but rather “queries per hour” and “BI assets in active use.”
- Worked with management teams to embed BI assets and data-driven decision-making practices in all aspects of the company’s operation, raising the organization’s collective intelligence quotient for data-driven decision-making.
These BI sufficiency practices in turn create the sociotechnical preconditions for a rapid transition to advanced analytics. In particular, BI sufficiency:
- Exposes in-house technologists to the very real limits of backward- and inward-oriented historical analysis (often on summarized, normalized data) for [a] predicting future states and conditions and [b] suggesting what actions in the present are more likely to either bring a predicted future state into being or avoid an undesirable future state.
- Raises the imaginative ceiling of demand-side constituencies, allowing experienced BI users to clearly formulate their predictive and prescriptive analytics needs, without prompting or shaping by technologists.
The Pitfalls of BI Insufficiency
Organizations that have not achieved BI sufficiency, in contrast, engage in patterns of response and reaction where data-driven decision-making is both uniform and predictable, based on those organizations’ AMA scores:
- Organizations that have not achieved sufficiency in what we’ve come to call their “BI data stack” produce demand-side constituencies focused primarily or wholly on data acquisition, by whatever means available. This data acquisition includes informal data-gathering networks and the ungoverned use of personal productivity tools like Microsoft Excel as the data integration tool, database, and analytical engine. These organizations, without exception, have a large and poorly defined distributed analytics capability (and analytics cost) in the form of large numbers of employees with non-analytical job titles and responsibilities who spend most of their time engaged in data foraging, data integration, and data analysis, all conducted outside governed channels and platforms. In extreme cases, some of these distributed analytics teams self-formalize, develop technical competencies, and effectively enter into competition with organizationally mandated business intelligence teams.
- Organizations that have not achieved sufficiency in what we’ve come to call their “BI analytical stack” have not succeeded, for the most part, in embedding descriptive and diagnostic business intelligence in their organizations’ ways of working, business processes, and key decision-making processes. In short, they have failed to make data-driven decision-making the norm, day-to-day, in their organizations. As a result, “best practice” in these organizations remain demarcated by the company’s better analysts and storytellers, who are often accorded hero status in the organization (while frequently employing tools and techniques imported into the organization from prior employers). This approach contradicts a "best practice" framework that should be defined by the organization's systemic ability to make more, faster, and better data-driven decisions. This should be embedded in the company’s daily practices and considered the price-of-employment, as opposed to heroic achievement.
Why BI Maturity Matters—Key Takeaways
- Scales data-driven decisions and culture: In our AMA assessments, the vast majority of business stakeholders are leveraging business intelligence. We see data culture and data literacy initiatives driven around BI users and how effectively they can use those assets.
- Unlocks business value from data investment: Business intelligence is the primary point where key stakeholders are accessing data and insights across the organization. We see a lot of frustration from our clients in the level of adoption and engagement across the entire data stack. BI maturity creates an environment where adoption and engagement increase, which then creates a feedback loop for data investment and the business value it brings.
- Enables effective allocation of data and analytics resources: We are in too many analytics maturity assessments where the vast majority of analytics and BI organizations are servicing ad hoc reporting requests, and not operating in the higher value areas of the business. Introducing things like self-service can help free up those resources and make the organization more effective.
- Generates demand for advanced analytics and AI: As organizations get more familiar with incorporating data in their decision process, it generates appetite and demand for more. This directly ties to the BI sufficiency problems discussed above. We know this is true because there’s an imaginative ceiling we see from data consumers, where decision-making needs are reduced to data or access to reports and dashboards. The process of BI maturity gets at that imaginative ceiling and raises it, showing decision-makers the limits of descriptive and diagnostic analytics, thus creating analytic breakthroughs for the enterprise.
- Builds foundation for advanced analytics and AI: Based on multiple assessments, we know that the ability to deliver effective BI solutions forces organizations to resolve their data issues, and build a set of processes and behaviors that are readily applicable to development of advanced analytics and AI.
Based on these insights, IIA has developed a Business Intelligence Maturity Framework for data and analytics leaders and their teams. This framework focuses on essential attributes of business intelligence maturity, providing the groundwork for the development and maturity of advanced analytic and AI. In our upcoming blog in this series on BI maturity and its pivotal role in a successful transition to AI, we will delve into this framework. It encompasses 10 competencies spanning a company's data and analytical stacks and confronts the question: How much BI is enough?