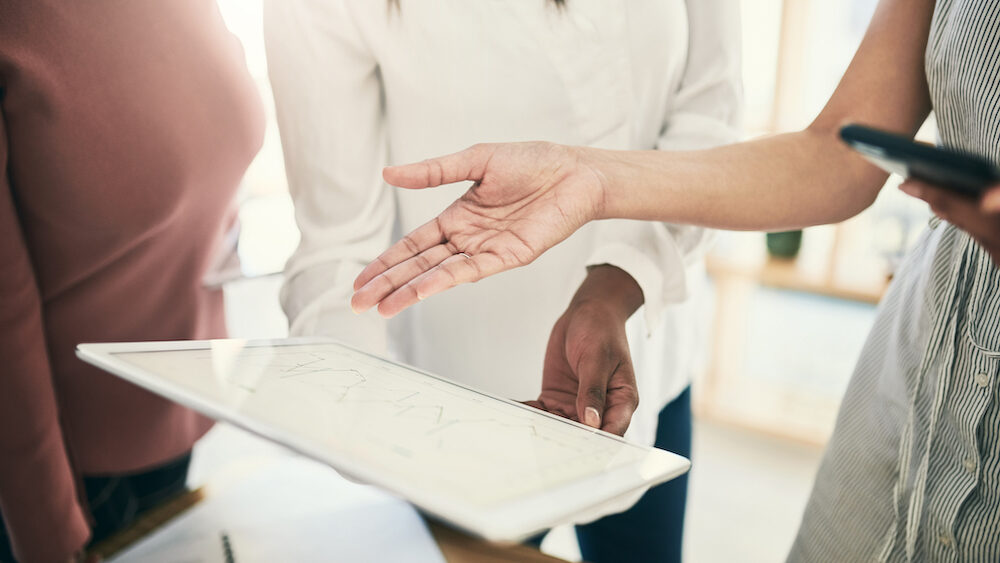
In the start of this series, I introduced the diamond graphic below and some principles, all grounded in the truth that every co-worker in the organization needs to increase their data knowledge. In previous blogs, I highlighted that C-suite leaders need to understand a lot more about data and analytics fundamentals and that non-exec leaders need to do a lot more with data and analytics workers than those at the top. Still, as the allegory of three frogs on a log reveals, deciding to act on data-driven decisions and actually doing so are not the same. In most companies, unit managers, area managers, and folks like that drive the everyday actions. If, hypothetically, your analytics for a QSR reveals both customer satisfaction and profits increase with suggested selling—those analytics mean nothing unless the selling is suggested in the store, or on the app. And managers must lead co-workers everyday to make that happen.
For these managers, the key focus is explaining why data and analytics are being tapped to find new ways to overcome challenges and seize opportunities. For these managers, the principles below should come alive in these ways:
- Everyone from the CEO to the hourly co-worker needs to have time set aside for data education and training designed to connect to their work. As data-driven decisions go from agreed to implemented, it’s critical to bring these managers in (if not earlier). When the decision drives new ways of working, it’s critical to build in time to explain why and how the data drove the decision and to surface concerns early.
- The volume of time increases as you get closer to the middle of the organization; for this group, the time depends greatly on how much data their teams create and how often or how large the changes to the ways of working are as a result of data-driven insights. At the extreme end, there are people managing call centers, transportation links, patient care facilities, assembly plants, and similar large-volume, high-paced environments. These managers need to know that data quality, and by extension data value, starts with them. And, on the other side, analytical optimization engines are likely to put demands on how they work, staff, and plan. Because of the pace of work and how integral data is to it, there is no choice but to integrate the education into everyday work, so the learning will be a little at a time, continuously.
- The depth of training increases as you get to the middle of the organization. Given the extreme bias toward action these folks have, as well as the delivery focus of their teams, classroom training is not likely. Instead there should be live chances to provide feedback on the data-driven decisions, often embedded in systems like labor scheduling systems. Deploying new processes that are born of data insights and leverage data insights offers a great time to anchor some data knowledge. Finally, if these roles lead any manual data entry (yes, that still exists), then helping these folks see how valuable their work is in the data-driven context is essential.
- The hands-on nature of actively working on analytics increases as you get to the middle of the organization. With embedded analytics increasing, these managers will need enough data knowledge to be effective at implementing these solutions—and enough to give feedback when the data-driven way won’t work.
- From the bottom or the top, subjects are added as you get to the middle, and this group plays a key role in why this new approach or change was decided. While they shouldn’t explain how the random forest model selected the add-on sales, they should honor the knowledge and experience of their co-workers. They should share that there was a lot of work put into making the right decision and ask the co-worker to give it a try, in the same way they likely have watched a Netflix recommendation.
- The bigger the company, the more strata in the diamond. This diamond represents your average F1000 company and is meant to represent a non-digitally native company (aka 99% of companies). For large global companies, the challenges this group faces in learning more about data include both time and speed of execution. There’s no great guidance here, except to frame learning about data as a development opportunity and being data driven as a matter critical to the company’s success.
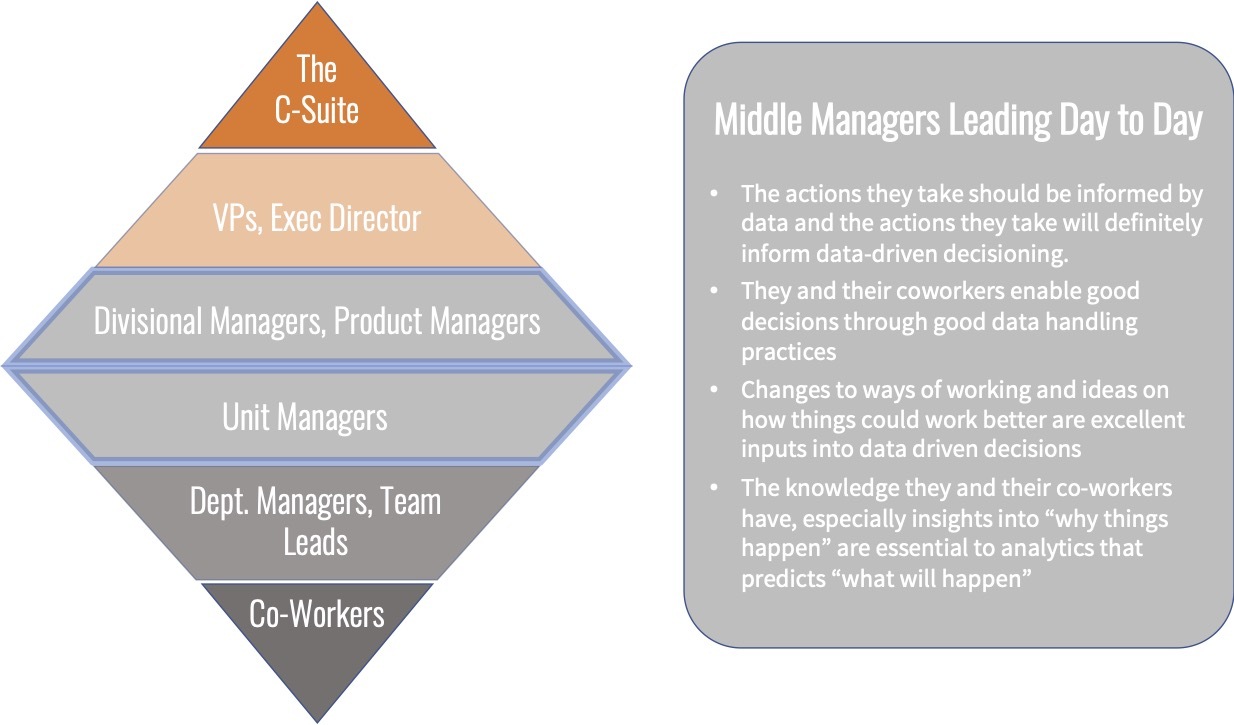
Data Knowledge Focused on the Why
In the previous parts of the diamond, there is much to learn about where to deploy analytics; what data can be leveraged to drive new understanding; and who in the organization should do what. For managers in this group, all of that is good to know, but why and how to deploy data insights is “need to know.”
So, while it’s still good to think about data knowledge across the dimensions below, the focus should be dedicated to the why and the how.
1.) Data – Where managers are being actively developed for more senior roles, they can be taught about some of the more abstract elements of data, like:
- 5 attributes of data that include volume, velocity, variety, veracity, and value, as well as why different attributes lend themselves to different types of business problems that can be solved. Additionally, they should understand the basic technical underpinnings of how data is ingested, stored, processed, analyzed, and presented.
- The shape of your internal data and your potential of external data. For non-execs in most legacy companies, the potential from internal data is far from maxed. However, they should look to inspiration, like Walmart using weather data to price steaks, and use that inspiration to stretch themselves and their departments in terms of data ambition.
- The key focus should be why data quality and integrity matter and how they play an essential role in keeping data fit for purpose.
2.) Algorithms and Models – Again, if these managers aspire to become Executive Directors and VPs, then some abstract knowledge about models could be useful. For example, they should understand that:
- Simple models that drive the most common decisions, like customer attrition, are increasingly commodities that can form the base of analytics in a function. However, investing in the knowledge and capability to build more complex models is likely needed sooner rather than later.
- Since analytical models can be unintentionally discriminatory, these managers should be alert to this possibility and primed to act if they see bad behavior.
- However, the most essential thing they should know is that the best models are imperfect and made more so with bad data. This reality requires a data lesson in the algorithm and model part because they have such an outsized impact on data quality.
3.) Organizations and Roles – There is no ideal organizational model and data roles are ever shifting. Still, amongst this flux some key principles apply:
- Managers might need to know to whom they should speak if the model predictions seem to be off, but otherwise there’s little reason they need to be highly educated about the various ways to organize data teams.
4.) Data Culture – yes, it’s a thing! It’s a big thing. And IIA has defined it well:
- “Data-driven businesses let data guide decisions, outcomes and strategies, even when the data goes against inclination and time-honored assumptions. Having a data-driven culture means that you go where the data and your interpretation of the data lead you, rather than using data selectively to reinforce your positions for other reasons or ignoring the signals in your data when those signals don’t align with your preferences, expectations or desired outcome.”
So if you want to ensure that data-driven decisions are executed, you will want managers who “walk the walk” and call out their co-workers who don’t.