
In the start of this series, I introduced the diamond below and some principles, all grounded on the truth that every co-worker in the organization needs to increase their data knowledge, including the co-workers at the top.
First a refresher on the principles introduced in the first of this series, with a focus on what’s truer to the C-suite:
- Everyone from the CEO to the hourly co-worker needs to have time set aside for data education and training designed to connect to their work – C-suiters especially don’t have “slack time” in their schedule, so if it’s not in the agenda it won’t happen.
- The volume of time increases as you get closer to the middle of the organization, so C-suite needs less time than most.
- The depth of training increases as you get to the middle of the organization, so to some the training will feel fuzzy and surface level, but for the C-suite the depth will be right.
- The hands-on nature of actively working on analytics increases as you get to the middle of the organization, so C-suites will rarely need to practice code or interpret statistical models.
- From the bottom or the top, subjects are added as you get to the middle, so the C-suite, should they wish to get more depth around analytics at their company, can lean on their direct reports.
- The bigger the company, the more strata in the diamond. This diamond represents your average F1000 company and is meant to represent a non-digitally native company (aka 99% of companies), this is especially true in the C-suite where years of experience may well further the digital divide (the average CEO at 50 is not digitally native).
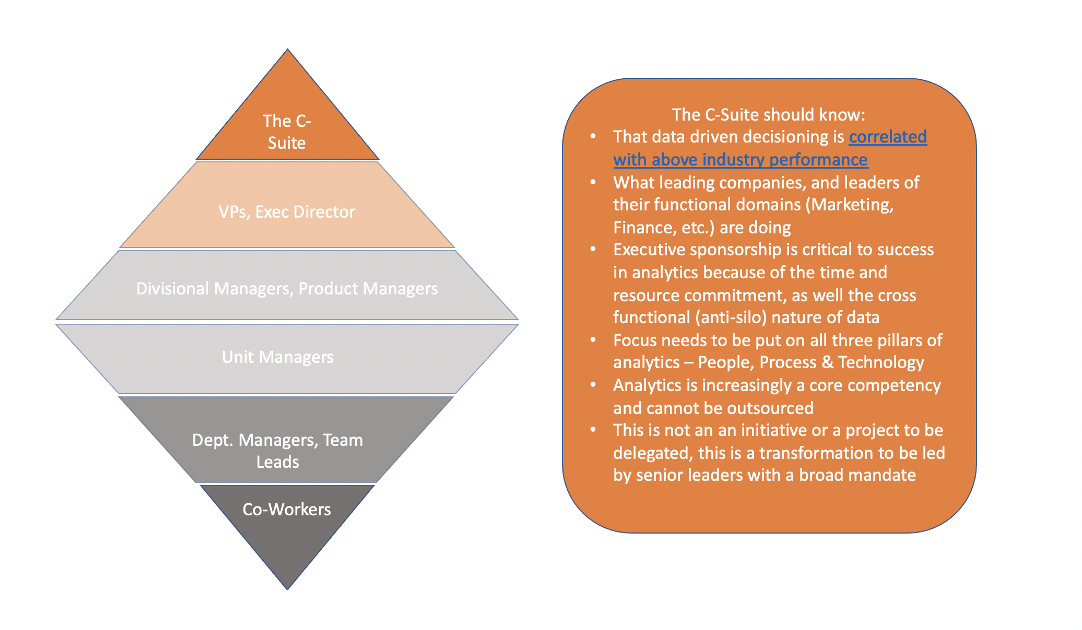
What Do C-Suite suite Folks Need to Know About Data?
For most of the analytics practitioners I speak to the answer to the question above is “way more than the C-suite thinks.””. And for the C-suite folks I talk to the answer is “way less than the analytics practitioner expect me to.” To bridge this divide, it’s helpful to think about where to find the balance between these two answers along several dimensions.
But first, C-suiters should understand a few things. First, companies that make decisions with data make better decisions, more quickly and so outperform their rivals. Second, the road to this nirvana is long and difficult, requires deliberate consistent focus, new capabilities, and sizable budgets. Only the C-suite has the social, political, and financial capital to sustain this journey. For this reason, they should be as knowledgeable about data as they need to be to sustain this high level of support. At a minimum I suggest these four areas to focus on:
1. Data – it’s not asking too much for C-suite executives to understand some of the fundamental aspects of data since an understanding of these fundamentals will enable them to better understand the talent and tech investments that are needed to manage and utilize this data, as well as the importance of basic data hygiene across the company, which requires discipline. They should understand:
- 5 attributes of data volume, velocity, variety, veracity, and value and why different attributes lend themselves to different types of business problems that can be solved. For example, if you want real-time dynamic pricing, you need volume and velocity for sure and likely variety as well.
- The shape of your internal data and your capacity with external data. These two are placed in that order because until your firm has a good understanding of your internal data (its attributes, characteristics, etc.) working with external data will have little reward, but might be highly tempting to C-suite folks who are enamored with stories of Walmart using weather data to price steaks.
2. Algorithms and Models – it’s here that I lose friends and alienate people. Some folks wish for C-suiters to be conversant in the differences between a k-Nearest Neighbor and a simple Ordinary Least Squares Regression algorithm, but I think C-suiters (of large companies specifically) should know some key concepts related to algorithms. Specifically:
- A complex model is not an inherently better model.
- Measuring model performance requires relatively high statistical acumen, but also business knowledge to interpret those statistics into business decisions.
- The gains in complex models, like Convolution Neural Networks for example can be offset by the lack of ability to understand why those models spit out a certain output. They are less transparent or explainable and this choice must be explicitly accepted.
- A good data scientist should be able to explain why they chose the model they chose, why it’s better than other models and why, even though it’s better what, its flaws are.
3. Organizations and Roles – There is no ideal organizational model and data roles are ever-shifting. Still amongst this flux, some key principles apply:
- Most companies that have been building data and analytics competence are pushing analytics out closer to decision-makers and most C-suiters should be concerned if this is happening, which I argued for more depth here.
- Data roles are increasingly specialized and require a more deliberate and robust talent management approach than in the past, as explained by IIA’s Bill Franks in this webinar. Gone are the days when of “get me 50 data scientists and we will sort it out.”
- Analytics is a team sport requiring more engagement from business leaders and, if you’re really ambitious, roles like a Product Manager.
- Altogether these points indicate that, if you want to scale and develop analytics, leadership talent matters as much as analytics acumen.
4. Data Culture – yeah, it’s a thing. It’s a big thing. And the IIA has defined it well:.
“Data-driven businesses let data guide decisions, outcomes and strategies, even when the data goes against inclination and time-honored assumptions. Having a data-driven culture means that you go where the data and your interpretation of the data lead you, rather than using data selectively to reinforce your positions for other reasons or ignoring the signals in your data when those signals don’t align with your preferences, expectations or desired outcome.”
And if this doesn’t describe how you act in the C-Suite, change. And as they say, change starts at the top.